A team of researchers from several institutions, including the Universities of Warwick and Oxford, has recently published a paper on the medRxiv* preprint server describing a new diagnostics method for the identification of SARS-CoV-2. Making use of a combination of fluorescent labeling, widefield microscopy, and machine learning, the team's new approach can accurately identify SARS-CoV-2 in just a few minutes. This is significantly faster than current approaches.
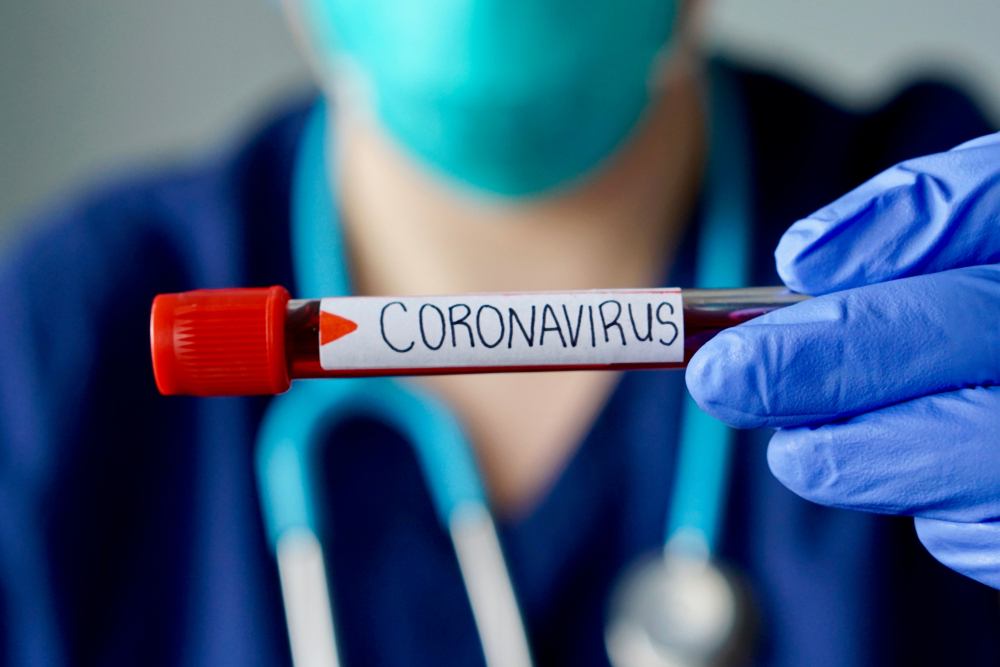
Image Credit: SamaraHeisz5/Shutterstock.com
SARS-CoV-2 is the novel coronavirus responsible for the current COVID-19 outbreak. It has caused global disruption and more than one million deaths worldwide. With concerns rising as global infection rates continue to grow, many governments have endeavored to increase SARS-CoV-2 testing capacity. This is as a means of tracking the virus’s spread throughout the population and monitoring the success of the control measures introduced.
Part of the challenge for increasing testing capacity is the slow nature of the current diagnostic approaches for SARS-CoV-2. At present, reverse transcriptase-polymerase chain reaction (RT-PCR) is considered the ‘gold standard’. However, such analysis can only be carried out in specialist labs with results taking several hours to acquire. As recently seen in the UK, RT-PCR also requires many specialist chemicals that mean testing capacity can be negatively impacted by supply chain issues.
Quick SARS-CoV-2 Testing Alternatives
The study offers an alternative approach to diagnosis in just a few minutes. By tagging the virus particles with highly fluorescent DNAs, the team could then use wide-field microscopy to image the labeled viruses.
As different virus types and strains have different surface chemistry, sizes, and shapes, each has its own unique distribution of fluorescent tags on its surface. The team was able to capture images of this fluorescence distribution using wide-field imaging on a commercially available fluorescence microscope.
Imaging a single virus is no easy task owing to its small size. Even with some of the viruses picking up multiple fluorescent labels, the excellent spatial resolution of the microscope was still needed to achieve clear images.
Faster than the Eye
Rather than performing a time-consuming manual analysis of the images, the team also used a machine learning approach to identify and differentiate between different types of viruses.
The team needed to firstly find a way to filter the microscope images for unwanted artifacts and translate the information contained in the image into a format that could be inputted to the convolutional neural network they were using. This is a specific type of machine learning algorithm.
The process of the initial data sorting could be automated, taking just two seconds to process the image for subsequent analysis. Samples containing viruses showed significantly higher overall signal levels than virus-free samples, so this preliminary processing could already identify which samples contained viruses or not.
Distinguishing between similar viruses such as influenza and SARS-CoV-2 proved to be slightly more difficult as the surfaces of the viruses and their uptake of the tagging molecules are similar. However, while the differences were invisible to the human eye, the team found its machine learning algorithm was capable of detecting subtle differences in the images obtained from influenza or SARS-CoV-2 viruses and could accurately distinguish between the two.
Read more: Optical microscopes on the market today
Machine Learning for SARS-CoV-2 Identification
The team demonstrated that its approach was successful at identifying SARS-CoV-2 not just from laboratory samples but throat swabs and other clinical samples. These are typically more challenging for image recognition algorithms. This may be because the clinical samples contain a wide range of virus concentrations, the viruses are obscured by residual cellular material, or simply that they have been collected by different sampling techniques.
Overall, the team has estimated a limit of detection for SARS-CoV-2 of 6x104 PFU/mL for its current approach. This does mean that, at present, their microscopy-based approach is less sensitive than amplification-based methods such as RT-PCR. However, the team is confident it can be improved substantially with the incorporation of different sample preparation techniques.
By making use of a compact, commercially available microscope and automated machine learning, the team has made an important step in finding an approach towards a much more rapid SARS-CoV-2 diagnosis which is not so heavily dependent on complex sample preparation.
*Important Notice
medRxiv publishes preliminary scientific reports that are not peer-reviewed and, therefore, should not be regarded as conclusive, guide clinical practice/health-related behavior, or treated as established information.
References and Further Reading
Shiaelis, N., Tometzki, A., Peto, L., Mcmahon, A., Hepp, C., Bickerton, E., … Robb, N. C. (2020) Virus detection and identification in minutes using single-particle imaging and deep learning. MedRxiv, 1–15. https://doi.org/10.1101/2020.10.13.20212035
Disclaimer: The views expressed here are those of the author expressed in their private capacity and do not necessarily represent the views of AZoM.com Limited T/A AZoNetwork the owner and operator of this website. This disclaimer forms part of the Terms and conditions of use of this website.