High-stability lasers can be used in several applications, including quantum computing, optical clocks, and fiber optic monitoring. Many of these applications involve the use of an interferometer, where two or more light sources are mixed, and the interference pattern is observed.
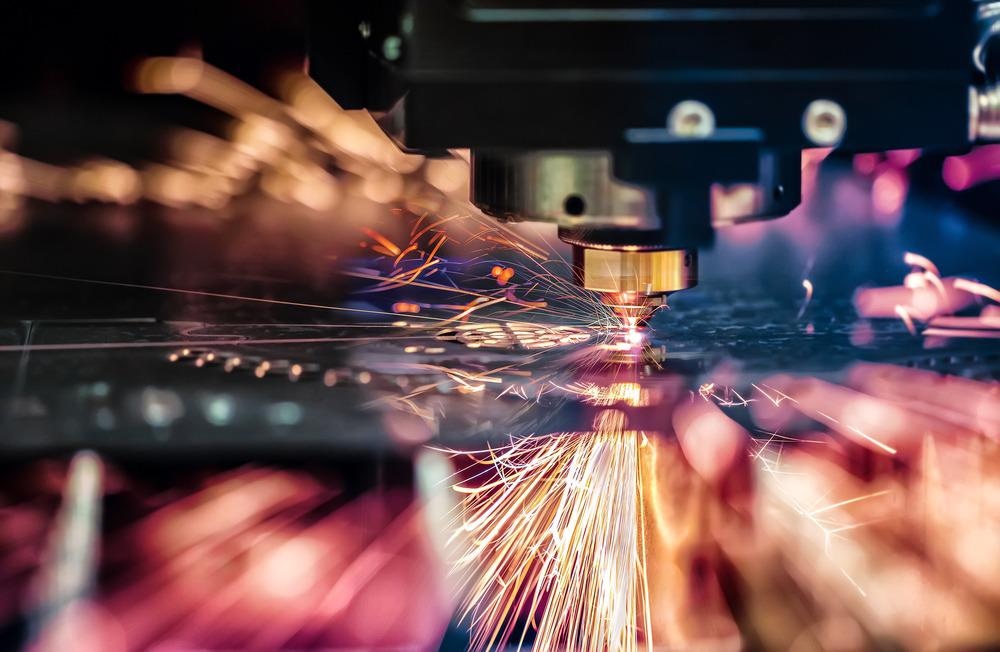
Image Credit: Andrey Armyagov/Shutterstock.com
Typically, a beam splitter is used to split a single laser beam into the multiple arms or different optical paths that make up the interferometer design. Any noise fluctuations caused by the source laser are the same in all the interferometer arms.
Interferometers are used for the most sensitive measurements, including metrology work. This means there needs to be a high degree of confidence that any perturbation of the interference pattern comes from a change in the system being measured, not in the laser source. One of the most famous, demanding examples of this technology is the optical interferometer at the heart of LIGO for the detection of gravitational waves.1
Laser Phase Noise
There are several types of fluctuations that can affect the overall noise associated with a laser pulse. This includes fluctuations in the intensity and the spectral profile. Some fluctuations can be relatively easy to correct with suitable diagnostics, such as the use of a reference measurement to monitor changes in intensity.2
However, for pulsed laser systems, accurate corrections rely on detectors being able to record single-shot measurements to make shot-to-shot diagnostics, which can be challenging at higher repetition rates.
Phase noise in laser systems can be more problematic. The phase noise refers to the fluctuation in the optical phase of the laser pulse and these fluctuations give an inherent linewidth to the laser output. However, these fluctuations can be more complex than just a shift in the central frequency but can also manifest as unequal changes in different parts of the sinusoidal oscillation of the electromagnetic field.
There is not one unique cause of phase noise either. There is the inherent quantum noise associated with the pulse, which comes from spontaneous emission of the gain medium where lasing occurs. Imperfections in optical components also lead to various optical losses that contribute to this quantum noise. Environmental fluctuations such as vibrational or thermal changes can also disturb the resonator cavity and contribute to the phase noise present.
The Noise Problem in Lasers
Many aspects of optical design try to minimize the effects of environmental influence on the laser components. This includes the use of heavy optical tables to minimize vibrational disturbances of the system and hermetically sealed components are increasingly becoming part of laser systems to avoid changes in humidity affecting performance.
While such engineering is sufficient for many applications, dealing with noise is one of the biggest obstacles to optical methods of larger-scale quantum computing.3
As noise-free lasers do not exist and some amount of quantum noise is inherent to the lasing process, it is important to characterize and understand non-random noise processes to be able to correct them.
Recent work from the Technical University of Denmark (DTU), in collaboration with NKT Photonics, has taken another look at how phase noise behaves in high repetition rate fiber lasers using a highly sensitive experimental set-up to measure how the laser amplifier noise affects the phase fluctuations of the signal.4
Looking Ahead to Machine Learning
The group at DTU has been developing many machine learning-based approaches for photonics applications that are recognized as an excellent tool for identifying relationships and structure in the measured noise of the signal.5
With this information, it is possible to design filters that could dynamically remove noise from the signal, leaving only the inherent quantum noise remaining.
The team has had significant success in reducing the phase noise with a suitable choice of filter based on the information obtained from their measurements 4 The ability to perform precise phase noise measurements means it is possible to work out the noise limit a given laser system could hope to achieve and then design filters to remove any additional perturbations.
Machine learning could offer a route to the dynamic evolution of those filters to potentially adapt to changing environmental factors. While most laboratory laser sources exist in relatively controlled environments, fiber optic cables can operate over very large distances in harsh and unpredictable conditions.
Eliminating all unnecessary noise will be key for improvements in the optical laser systems for many quantum technologies that rely on the preservation of coherences, be that for cold atom trapping or quantum computing. It is this noise that contributes to the information loss and degradation of these systems. The next big challenge is developing efficient machine learning algorithms to perform these corrections without unreasonable computational power and energy demands.
References and Further Reading
- Hall, E. D., Matichard, F., Lantz, B., Mittleman, R., Mason, K., Kissel, J., Allwine, E., Barnum, S., Birch, J., Celerier, C., & Clark, D. (2015). Seismic isolation of Advanced LIGO : Review of strategy , instrumentation and performance sensitivity Seismic isolation of Advanced LIGO : Review of strategy , instrumentation and performance. Classical and Quantum Gravity, 32, 185003. https://doi.org/10.1088/0264-9381/32/18/185003
- Lang, B. (2018). Photometrics of ultrafast and fast broadband electronic transient absorption spectroscopy: State of the art. Review of Scientific Instruments, 89(9). https://doi.org/10.1063/1.5039457
- Harper, R., Flammia, S. T., & Wallman, J. J. (2020). Efficient learning of quantum noise. Nature Physics, 16, 1184 https://doi.org/10.1038/s41567-020-0992-8
- Zibar, D., Pedersen, J. E., Varming, P., Brajato, G., & Da Ros, F. (2021). Approaching optimum phase measurement in the presence of amplifier noise. Optica, 8(10), 1262. https://doi.org/10.1364/OPTICA.431668
- Gao, L., Chai, Y., Zibar, D., & Yu, Z. (2021). Deep learning in photonics: introduction. Photonics Research, 9(8), DLP1. https://doi.org/10.1364/PRJ.428702
Disclaimer: The views expressed here are those of the author expressed in their private capacity and do not necessarily represent the views of AZoM.com Limited T/A AZoNetwork the owner and operator of this website. This disclaimer forms part of the Terms and conditions of use of this website.