X-Ray fluorescence (XRF) is a well-established technique in material analysis.1 It quantifies the elemental distribution of complex materials, thereby revealing their structure and composition.2 Conventionally, further multivariate analysis of XRF results is required to derive useful information.
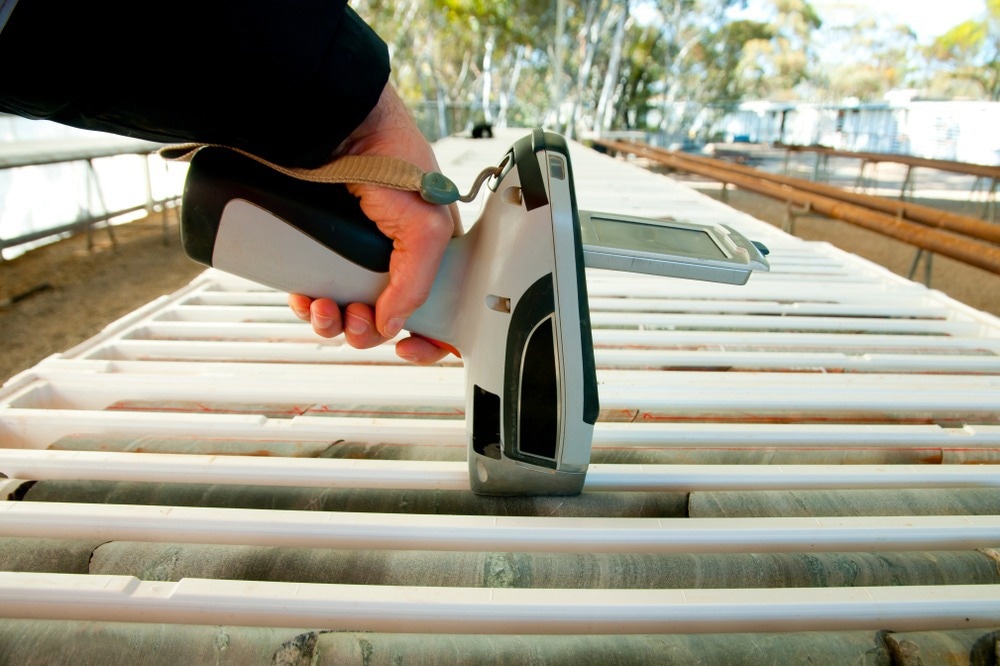
Image Credit:Adwo/Shutterstock.com
With the advancement of machine learning (ML) techniques, the speed and accuracy of XRF data analysis and postprocessing activities have increased.1 ML models can overcome physical constraints, such as probe profiles, and enhance the resolution of images.2
This article investigates how the convergence of XRF and ML is advancing material analysis capabilities.
XRF and ML Integration: The Basics
XRF is a non-invasive and non-destructive elemental analysis technique for solids and liquids.3,4 Samples are probed using a strong X-Ray beam, and the emitted fluorescent X-Rays are collected using an energy or wavelength-dispersive detector.
The energy/wavelength of the emitted X-Rays assists in identifying the elemental composition, while their intensity reveals the concentration of elements.3
XRF spectroscopy is favored for the tomographic visualization of materials due to the high penetration depth of X-Rays (up to tens of microns).2 Multivariate or pattern recognition techniques are used for qualitative or quantitative analysis of XRF results.1 The XRF intensity measurements can be modeled as a convolution between the sample and X-Ray probe, integrated with other scanning parameters like step size.2
ML enables computers to learn and replicate intelligent human behavior through training data without being explicitly programmed. An ML system can perform different functions using the training data, such as descriptive (describing what happened), predictive (predicting future events), and prescriptive (suggesting actions).5
ML models like support vector machines, gradient boosting, random forests, and neural networks can be integrated with XRF for data pre-processing, pattern recognition, and performing predictive analytics using the entire XRF spectra.4
ML models can successfully decouple the X-Ray probe impact from the XRF signal using a residual dense network. This improves the accuracy and enhances the visuals in image super-resolution tasks.
ML can extract scale-variant features from XRF data and bypass abundant low-frequency information, thereby increasing XRF image resolution and revealing distinct features that are otherwise invisible.2
Enhancing Precision and Efficiency in XRF Analyses
Some XRF analyses can only reveal qualitative information, largely dependent on time-consuming operator involvement. This can be overcome by integrating ML in XRF analysis and using predictive analytics.
ML not only reveals precise qualitative information but can also quantify these details; hence, enhanced XRF detection capabilities can be observed.1 Additionally, with low human interference, there is a reduction of false positives or negatives.
ML algorithms can also be used to create and implement calibrations. While traditional empirical modeling could create only material-specific calibrations, different ML models can be used in combination to improve these calibrations.
For example, ML-XRF analysis of archaeological ceramics can produce calibration curves with sufficient cross-validation for lighter elements like Si, Al, and Na, even when the instrument is working at higher voltage settings.4
The integration of ML algorithms with XRF spectroscopy has enabled material classification through qualitative assessment. This allows for real-time optimization of XRF operation parameters, complete spectrum assessment, and identification of minor variations.
This enhanced precision and efficiency of XRF analyses has been observed in several applications, such as identifying brine pH levels, recognizing wood species, and differentiating between dinosaur bones.4
Expanding the Scope of XRF Applications with ML
As an imaging technique, XRF finds applications in various physical and biological disciplines, such as materials science, chemistry, microbiology, archaeology, and geosciences.
However, the resolution of XRF imaging is constrained by the X-Ray probe profile and scanning step size. The integration of ML techniques can increase the spatial resolution of XRF images by eliminating blurring effects due to the probe profile.2
XRF spectroscopy innovations are governed by advancements in X-Ray sources, photon detection tools, and computing methods. Thus, the integration of ML in XRF has opened new avenues of research and expanded the scope of XRF applications.
XRF-ML integration has enabled the dating of French gilt bronzes, the determination of carbon in geological samples, and the identification of soil moisture levels.4
The most prominent use of XRF is in analyzing materials of cultural heritage structures.1 XRF is often employed to assess ancient paintings—to determine the period to which they belong and attribute authorship. However, such analysis becomes too complex due to multiple layers of materials painted on different supports.1
The application of ML models provides a better understanding of the past, as ML-enabled XRF can handle complex materials or detect trace elements.4
ML-XRF: Challenges and Solutions
The convolutional neural network is an ML model often used to analyze visual images. However, CNN training requires a large amount of data, for which synthetic spectra are generated, and a transfer learning technique is applied.
The analysis results are found to be good for synthetic spectra; however, they are only modest for the real spectra. To overcome these disadvantages, autoencoder neural networks have been developed.1
Natural images are generally used to train ML models, which poses a challenge in XRF data analysis. There is a domain gap between XRF data and natural images due to the specific noise features (like photon-counting noise or background fluorescence) and inherent complexities of XRF datasets.
Thus, special adaptation strategies are required for models initially trained on natural image datasets comprising noise in the form of pixelation, compression artefacts, or light and color variations.2
Apart from the ML-XRF integration hardware requirements and costs, the use of ML in data analytics presents inherent challenges. Explaining the workings and decision-making processes of ML models poses difficulties, and the models can be misled by even minor inaccuracies in the training data. Thus, errors and biases in human-created training data can be incorporated into ML models, perpetuating these issues further.5
Future Perspectives
Artificial intelligence (AI) tools can increase the sustainability of XRF analysis by increasing process speed and reducing an operator’s manual work. Autoencoder neural networks act as a dimension-reduction tool for XRF data, extracting the most informative features and allowing the most appropriate reconstruction of the primary XRF spectrum.1
As compared to traditional ML methods, deep neural networks can recognize essential patterns in raw spectra more efficiently. They enhance model accuracy and robustness with minimal human involvement in preprocessing and feature selection. Thus, deep learning and AI tools are being increasingly used for sustainable spectra treatments.1
Future developments in the field of XRF analysis will be powered by ML, including automation, AI-driven predictive maintenance, and real-time analysis.
Smaller and more powerful XRF instruments can be expected in the future, which, on integration with ML and AI, allow newer material investigations and reinvestigation of past analyses.4
Adopting interdisciplinary approaches that cover all aspects, from tool design to result analysis, is important for advancing material analysis technologies.
More from AZoOptics: Exploring the Impact of Skin Color on Optical Properties: Insights for Medical Optical Technologies
References and Further Reading
1. Andric, V., Kvascev, G., Cvetanovic, M., Stojanovic, S., Bacanin, N., Gajic-Kvascev, M. (2024). Deep learning assisted XRF spectra classification. Scientific Reports, 14(1). https://doi.org/10.1038/s41598-024-53988-z
2. Zheng, X., Kankanallu, VR., Lo, C.-A., Ajith Pattammattel, Chu, Y., Chen-Wiegart, K., Huang, X. (2024). Deep Learning Enhanced Super-Resolution X-Ray Fluorescence Microscopy by A Dual-Branch Network. Optica. doi.org/10.1364/optica.503398
3. Santiago, M. (2017). Basic Concepts of X Basic Concepts of X-ray Fluorescence. [Online] University of Puerto Rico. Available at: https://www.uprm.edu/geology/wp-content/uploads/sites/111/2017/03/xrf.pdf
4. Shugar, A. (2021). Advancements in portable and lab-based XRF instrumentation for analysis in cultural heritage: A change in perspective. Microscopy and Microanalysis. doi.org/10.1017/s1431927621009077
5. Brown, S. (2021). Machine learning, explained. [Online] MIT Sloan School of Management. Available at: https://mitsloan.mit.edu/ideas-made-to-matter/machine-learning-explained
Disclaimer: The views expressed here are those of the author expressed in their private capacity and do not necessarily represent the views of AZoM.com Limited T/A AZoNetwork the owner and operator of this website. This disclaimer forms part of the Terms and conditions of use of this website.