The fusion of artificial neural networks (NNs) with photonics has generated significant implications across various scientific fields and industries. A new tutorial review published in the journal Advances in Optics and Photonics provides a comprehensive exploration of this convergence. The tutorial, primarily aimed at a scientific and industrial audience, underscores the pivotal role neural networks are set to play in optical research, engineering, computer science, and applied mathematics.
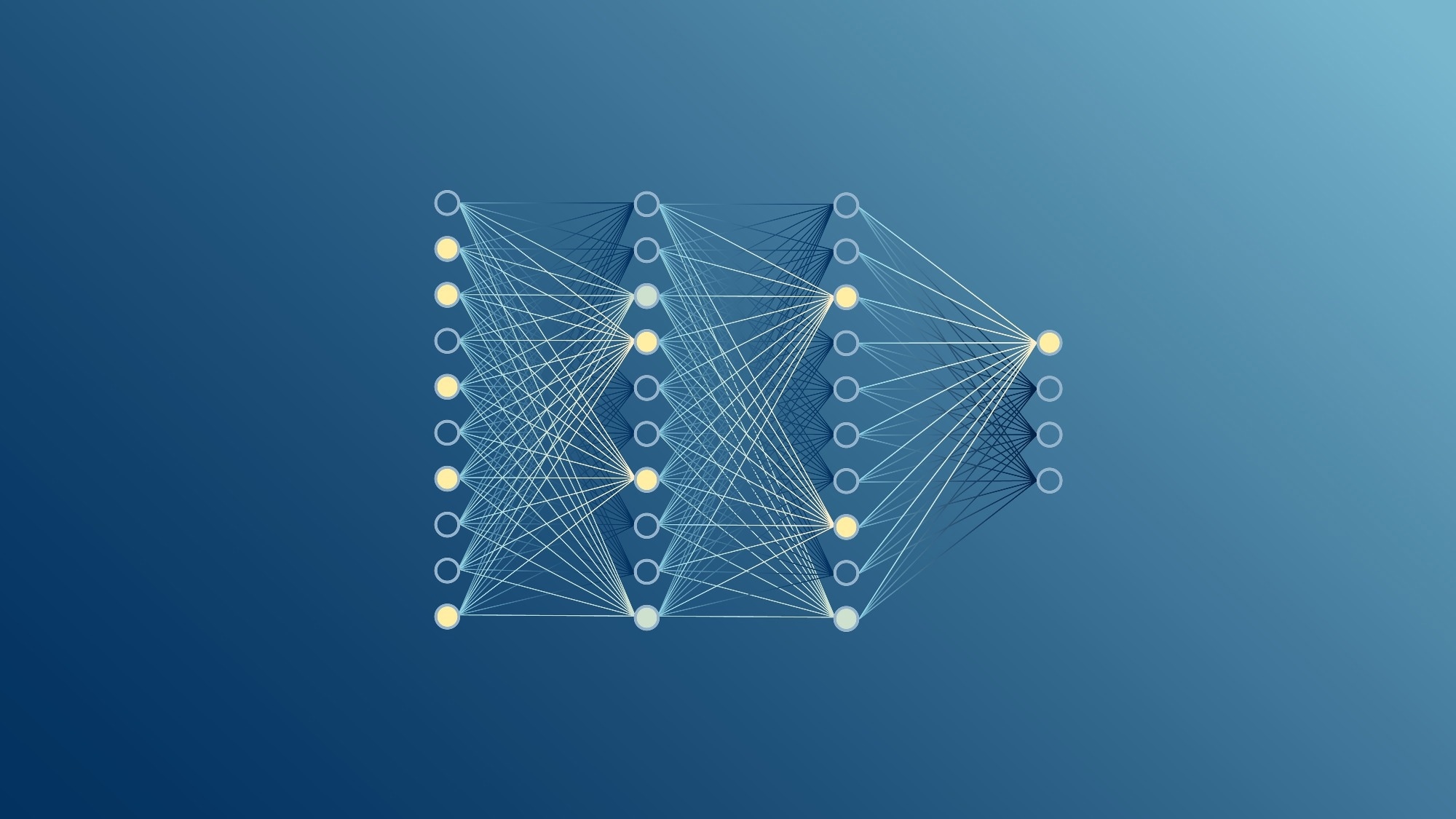
Image Credit: Qpt/Shutterstock.com
Neural Networks in Photonics Overview
Understanding the critical neural network types is fundamental. Convolutional neural networks (CNNs), recurrent neural networks (RNNs), and feedforward neural networks serve as the foundational elements. These neural architectures bridge the gap between NN design theory and practical hardware implementation.
For instance, CNNs are instrumental in image analysis within photonics. They excel at pattern recognition and feature detection, offering applications in enhancing imaging resolution and precision. Industries reliant on high-precision imaging, such as medical diagnostics, microscopy, and remote sensing, stand to benefit significantly.
Balancing technical depth with clarity remains a challenge, underlining the importance of interdisciplinary knowledge exchange.
Applications of Neural Networks in Photonics
The authors provide a section of the research that delves into the practical applications of NNs in photonics. Recent advancements promise transformation in optical communications, imaging, sensing, and materials/laser design.
In optical communications, where intricate nonlinear equations govern signal propagation, NNs excel in modeling and controlling optical channels with precision. This opens opportunities for improved data transmission reliability in telecommunications, with substantial commercial implications.
Furthermore, in optical applications dealing with notable noise impact, machine learning methods exhibit strong noise mitigation capabilities. This stands to benefit industries reliant on reliable data transmission, such as optical signal processing, ensuring consistent connectivity and minimizing data loss.
Commercial Relevance and Future Prospects
The surge in optical data generation from advanced optical systems presents commercial opportunities. The researchers state that NNs enable more efficient data utilization, benefiting industries like manufacturing, remote sensing, and healthcare, resulting in informed decisions, process improvement, cost reduction, and product quality enhancement.
The application of NNs in material science and optical engineering holds the potential for innovative materials design and characterization. Customized materials with unique optical properties have the potential to revolutionize fields such as solar energy harvesting and optical device development, lowering production costs.
The amalgamation of data-driven methods and complex photonic systems allows for the discovery of underlying physical models and master equations. This presents an opportunity for more precise product design and optimization, reducing waste and increasing efficiency in sectors like semiconductor manufacturing.
The collaboration and knowledge exchange between data scientists and optical experts fostered in this tutorial-review promise disruptive technological advancements in the near future.
Conclusion
The fusion of neural networks and photonics offers more than theoretical musings; it yields tangible commercial prospects. These technologies have the potential to redefine the generation, processing, and interpretation of optical data. Industries ranging from telecommunications to healthcare can expect more efficient processes, improved product quality, and cost savings.
As neural networks evolve and their applications expand in photonics, they will lead to innovations transcending existing technological boundaries. The convergence of these two disciplines will fuel progress in both fields, ushering in a new era of commercial possibilities.
Reference:
Pedro Freire, Egor Manuylovich, Jaroslaw E. Prilepsky, and Sergei K. Turitsyn, "Artificial neural networks for photonic applications—from algorithms to implementation: tutorial," Adv. Opt. Photon. 15, 739-834 (2023) https://opg.optica.org/aop/fulltext.cfm?uri=aop-15-3-739&id=539680