A recent study published in Remote Sensing suggests an information-reinforced depth completion network based on single sparse depth input. The researchers used a multi-resolution dense progressive fusion structure to maximize the multi-scale information and point-fold the overall scenario. They re-aggregated the confidence and placed a new depth limitation on the pixel depth to bring the depth approximation closer to the experimental values.
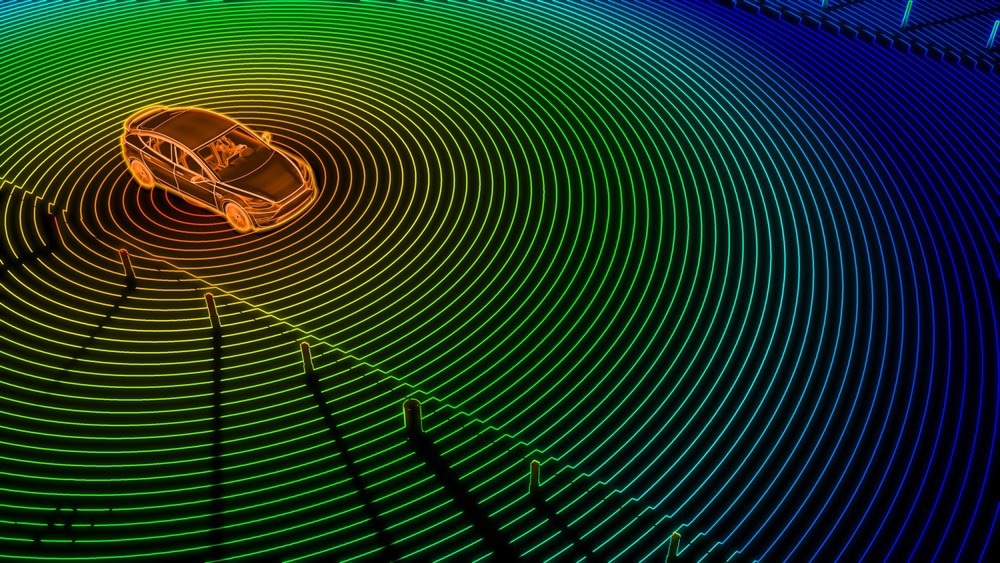
Study: An Efficient Information-Reinforced Lidar Deep Completion Network without RGB Guided. Image Credit: temp-64GTX/Shutterstock.com
Limitations of Traditional Interpolation Depth Completion Methods
Depth information is crucial for computer vision applications. Autonomous navigation systems, self-driving automobiles, and virtual reality need precise depth completion data. However, depth is limited and partially absent because of restrictions on the device and the surroundings. It is terminal in the reconstruction of 3D data.
Traditional depth completion methods frequently fall short of producing satisfactory results due to scarcity and insufficient prior information on the missing depth. Deep neural networks can be used for depth completion and outperform conventional interpolation techniques.
Single Sparse Depth Image for Enhanced Depth Information
The majority of depth completion networks use RGB images as an instruction to fill in the gaps in the depth information. It can offer useful edges for differentiating the shapes of various objects. The mapping relationship between a color image and sparse depth is formed through deep learning networks. The dense depth is regressed collectively after that. These data are constructed on the coordinated actions of several sensors, which necessitates the crucial supposition that the point cloud and the image correspond to one another even though they produce a better outcome. The datasets enable effective problem control. However, the reliability of the task cannot be guaranteed in a practical application due to the cost and unpredictable errors caused by the combined calibration of heterogeneous sensors.
Deep learning networks can be more appropriate for real-world circumstances by using a single sparse depth image for depth completion. Confidence re-aggregation with depth dependability of the neighborhood enables more precise local pixel depth estimation. The outside depth outside is more intricate and variable than the inside depth. Improving the overall texture and widening the range of variation for each target is necessary.
The point folding module and structurally dense progressive fusion processing further increase the precision of global prediction. It removes the extra information from RGB images and uses only one-fourth of the data present in other approaches, avoiding the need for error-prone, complex operations. It also significantly lowers deep learning network traffic while meeting accuracy and speed criteria, making it more applicable to real-world situations.
Relationship between Convolution and Confidence Enhance Depth Completion
The connection between confidence and convolution has attracted much attention to improve depth completion. N-CNN presented an algebraic restricted convolutional layer for sparse input deep learning networks using confidence as a twofold value mask to remove lost measurements. N-CNN used signal confidence as a convolution bound by confidence and an incessant measure of data uncertainty to improve depth completion.
SPN used confidence in an unsupervised manner by combining global and local information. The corresponding confidence maps are used to weigh the anticipated depth maps. PNCNN used the input confidence in a self-supervised manner to classify the interference depths in the input. They presented a probabilistic variant of N-CNN to provide a significant degree of ambiguity for the final prediction.
Multi-Scale Structure for Enhancing Global Depth Information
U-Net created the contraction and expansion path following the standard convolutional deep learning network structure. The multi-scale structure can increase the reliability of global data, which is highly beneficial for data gaps with limited depth. However, it ignores the original data of various resolutions and merely compensates for the loss of resolution brought on by convolution. The global depth completion impact can be enhanced by combining the global depth data from various scales.
Development of Deep Completion Network with Single Sparse Depth Input
Point cloud completion and depth completion are virtually identical. Both of them are 3D information approximations. The distinction is in how they depict three-dimensional data. In the point cloud completion, Folding-Net wrapped a fixed 2D grid into the form of an input 3D point cloud. The network enhances the likelihood of more points since it incorporates mappings from lower dimensions. Similar demands for additional pixels are made for sparse-depth images.
Wei et al. created a point folding module from 1D to 2D. It can expand the amount of information available globally. The researchers build a deep completion network based on single sparse depth input that combines local and global information, strengthening the knowledge and omitting the need for color image guidance. A confidence re-aggregation method that successfully re-aggregates the local region depending on the confidence of the pixel neighborhood was used to increase the estimation accuracy of local details. A dense progressive fusion network structure increases the accuracy of global completion even further by utilizing data from several scales.
Research Findings
In this study, the researchers created a deep learning network that can instantly complete a sparse depth problem without using a color image. They re-aggregated confidence to improve detail compared to the majority of previous approaches. The dense progressive fusion structure and point folding module enhance global information. The best use of information is made possible by the integration of modules.
Reference
Wei, M., Zhu, M., Zhang, Y., Sun, J., & Wang, J. (2022). An Efficient Information-Reinforced Lidar Deep Completion Network without RGB Guided. Remote Sensing, 14(19), 4689. https://www.mdpi.com/2072-4292/14/19/4689
Disclaimer: The views expressed here are those of the author expressed in their private capacity and do not necessarily represent the views of AZoM.com Limited T/A AZoNetwork the owner and operator of this website. This disclaimer forms part of the Terms and conditions of use of this website.