In a recent article published in Scientific Reports, researchers introduced a conveyor belt surface monitoring system to improve safety, efficiency, and sustainability in industrial production.
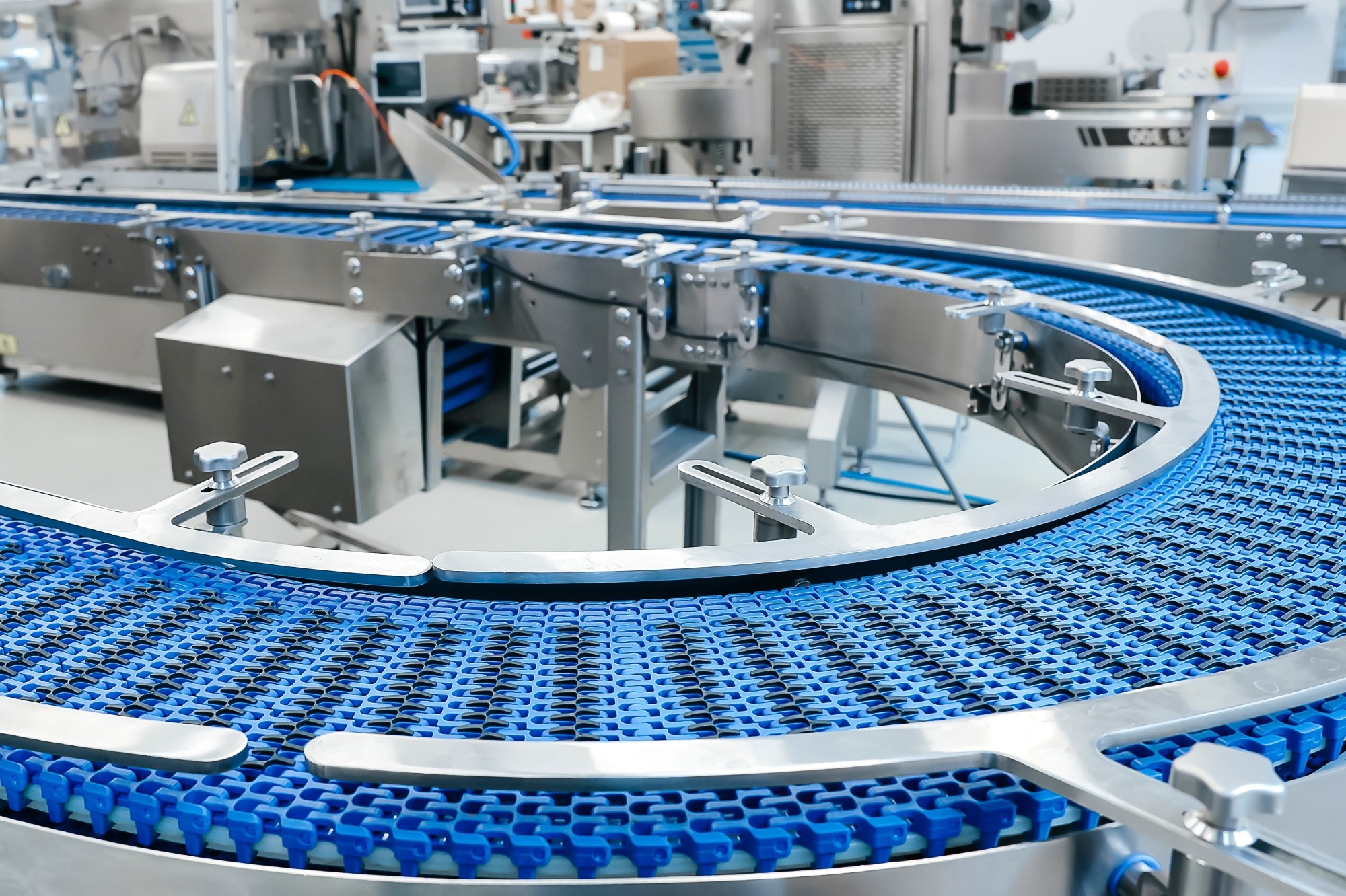
Image Credit: MariaTsygankova/Shutterstock.com
The system combines laser scanning technology with deep learning for high-precision, real-time monitoring, addressing the limitations of traditional methods. The aim was to tackle challenges in industrial environments, such as early detection of belt damage, which is important for maintaining operational efficiency and safety in harsh conditions.
Conveyor Belt Monitoring: Advancements and Challenges
Conveyor belt systems are essential in industries such as mining, manufacturing, and power generation, where they transport bulk materials over long distances. However, harsh operating conditions can cause wear and damage, leading to safety risks and operational inefficiencies. Traditional inspection methods, which rely on manual visual checks, are labor-intensive, time-consuming, and prone to errors, resulting in costly downtimes and hazards. Automated techniques capable of real-time monitoring are therefore needed.
The combination of laser scanning technology with deep learning offers advancements in addressing these challenges. Laser scanning provides high-resolution surface imaging, detecting small defects that manual methods may overlook. When integrated with deep learning models such as You-Only-Look-Once version 7 (YOLOv7), the system can quickly and accurately identify surface issues, including cracks and foreign objects, improving safety and operational efficiency.
An Intelligent Conveyor Belt Surface Monitoring System
In this paper, the authors introduced a novel intelligent conveyor belt surface monitoring system that combines programmable logic controllers (PLCs), high-performance industrial cameras, laser scanning technology, and deep learning algorithms.
The system's key feature is its PLC-integrated laser scanning capability, which adjusts automatically based on the conveyor belt's operational status. It is designed to address challenges in industrial environments, such as poor lighting and dust pollution in power plants and coal preparation facilities. Using a high-power laser scanner with an 880 nm wavelength, the system can detect defects as small as 1 mm on belts moving at speeds of 2–5 m/s.
The system comprises three main components: a detection device, a control box, and a host computer. The detection device, located beneath the conveyor belt, contains high-precision laser emitters and industrial cameras. The control box manages the power supply, on-site operations, and data transmission. The host computer, an industrial-grade PC (IPC), is responsible for image analysis, processing, and the human-machine interface (HMI). The hardware, including the IPC, Hikvision industrial camera, lens, laser emitter, and network switch, was selected to meet the system's functional and environmental requirements.
The software was developed using Siemens STEP 7-MicroWIN SMART for PLC control and included an intuitive HMI for operators. The PLC program’s modular design improved readability, maintainability, and scalability. For conveyor belts wider than the detection range of a single device, the system employs a dual-machine collaborative approach. The YOLOv7 model was chosen for defect detection due to its effective balance of speed and accuracy.
Experimental Outcomes and Performance Evaluation
The system was tested in a controlled indoor environment, capturing continuous images at 41 frames per second for real-time defect detection. The tests demonstrated its ability to accurately identify surface defects such as cracks, perforations, foreign objects, and wear patterns, even in dusty conditions. It achieved a mean Average Precision (mAP) of 90.7 %, outperforming models like residual network (ResNet) and matching Sparse R convolutional neural networks (CNNs) while requiring fewer computational resources. The system recorded a precision of 91.0 %, a recall of 89.5 %, and an F1-score of 90.2 %.
Grayscale images were used instead of red-green-blue (RGB) images, optimizing processing speed and reducing computational load without affecting accuracy. Field tests in quarries, cement plants, and coal mines further validated the system's robustness and adaptability to industrial environments, demonstrating its practicality for real-time conveyor belt monitoring.
Applications and Industrial Relevance
The presented conveyor belt monitoring system offers high accuracy, real-time performance, and adaptability, making it suitable for various industrial applications. Its reliable operation in harsh environments, such as mining and power generation, addresses the need for effective conveyor belt monitoring.
Real-time defect detection enables prompt issue resolution, minimizing production downtime and improving workplace safety. Integrated data logging and analysis support predictive maintenance strategies, reducing unexpected breakdowns and enhancing operational efficiency.
Conclusion
The conveyor belt surface monitoring system demonstrates progress in industrial automation and monitoring. By combining laser scanning and deep learning, it achieves high accuracy and adaptability, addressing the limitations of traditional inspection methods. The system highlights the importance of real-time monitoring for maintaining conveyor belt integrity and improving operational efficiency. As automated technologies are increasingly adopted, this system offers potential for enhancing productivity and safety in demanding industrial environments.
Future developments could focus on refining detection capabilities, incorporating additional sensors for greater precision, and integrating predictive maintenance features to minimize unplanned breakdowns and address minor defects proactively. This research provides a foundation for more efficient and reliable industrial operations.
Journal Reference
Wang, R., et al. (2024). PLC based laser scanning system for conveyor belt surface monitoring. Sci Rep. DOI: 10.1038/s41598-024-78985-0, https://www.nature.com/articles/s41598-024-78985-0
Disclaimer: The views expressed here are those of the author expressed in their private capacity and do not necessarily represent the views of AZoM.com Limited T/A AZoNetwork the owner and operator of this website. This disclaimer forms part of the Terms and conditions of use of this website.