A recent article in Scientific Reports explored the potential of combining infrared (IR) spectroscopy with machine learning (ML) algorithms for rapid and accurate diagnosis of myasthenia gravis (MG).
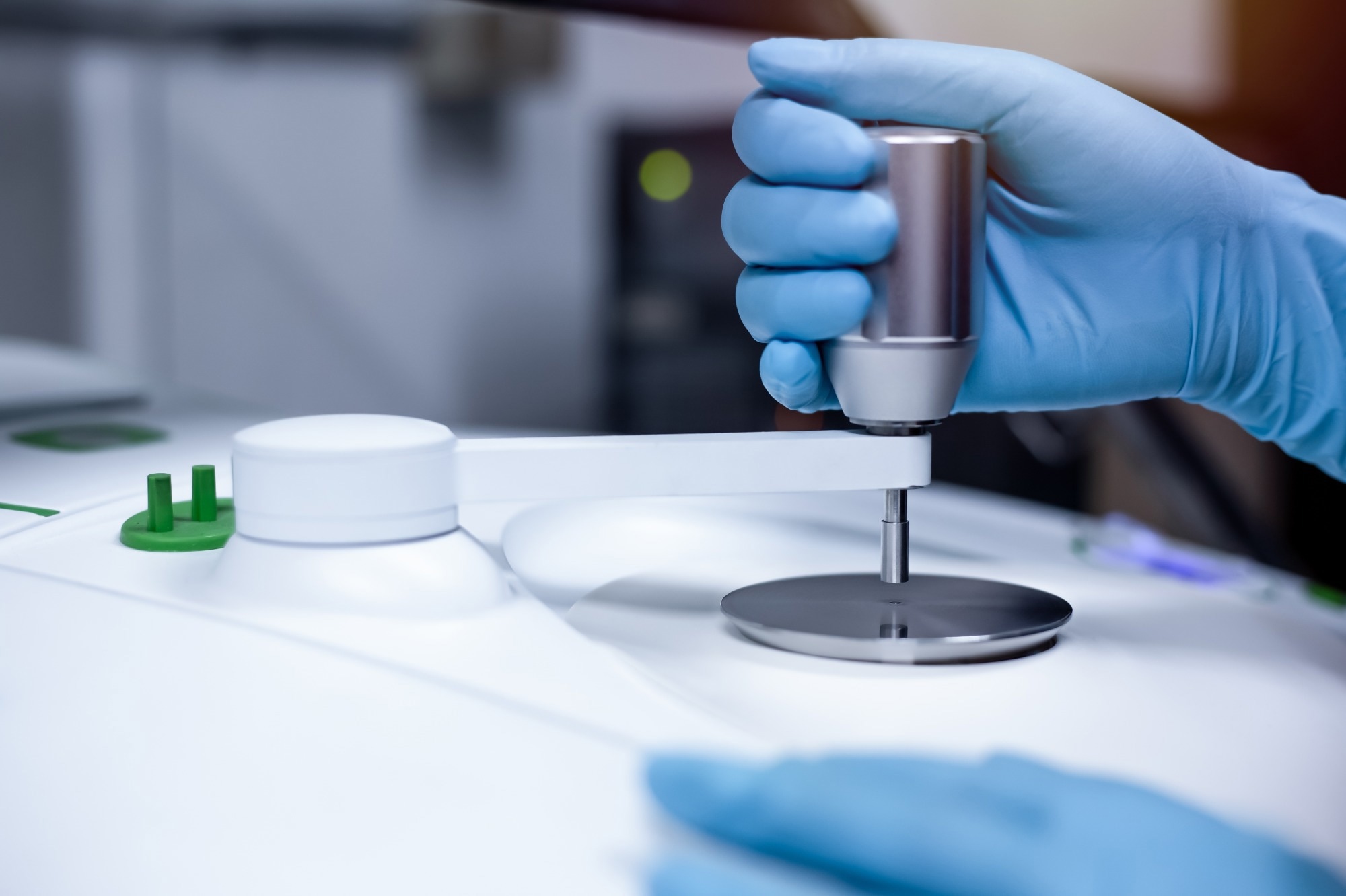
Image Credit: S. Singha/Shutterstock.com
This autoimmune disorder affects nerve-muscle communication. The researchers explored the unique spectral signatures of serum samples from MG patients and healthy individuals, using advanced techniques to identify potential biomarkers and develop a reliable diagnostic tool.
Background
IR spectroscopy is a non-invasive technique that analyzes the vibrational modes of molecules in a sample. When IR radiation interacts with the sample, specific wavelengths are absorbed, creating a unique spectral fingerprint that shows the sample's molecular composition and structure.
This method is widely used in chemistry, materials science, and biomedicine. In biomedicine, IR spectroscopy is used to study the composition and structural changes of biomolecules such as proteins, lipids, and nucleic acids in blood, tissue, and cell samples. This data helps identify disease-specific molecular patterns and supports the development of diagnostic tools for early disease detection and monitoring.
About the Research
The authors aimed to create a novel diagnostic tool for MG by analyzing serum samples using attenuated total reflectance Fourier transform infrared (ATR-FTIR) spectroscopy combined with supervised ML algorithms. Serum samples were collected from 24 untreated MG patients and 42 age- and sex-matched healthy individuals. These samples were analyzed using ATR-FTIR spectroscopy to obtain their spectral fingerprints.
The researchers then used various supervised ML algorithms, including principal component analysis (PCA), support vector machine (SVM), discriminant analysis, and a neural network classifier, to analyze the spectral data and differentiate between MG patients and healthy individuals.
The study focused on four spectral regions: the whole region, lipid region, protein region, and fingerprint region. Additionally, techniques such as peak signal-to-noise ratio (PSNR) analysis were utilized to evaluate the signal quality and accuracy of the spectral data. The parameters of the ML algorithms were also optimized to improve the classification accuracy.
Research Findings
The study found significant differences in the spectral signatures of serum samples from MG patients compared to healthy individuals. The most notable differences were observed in the protein region of the spectra, suggesting changes in protein composition and structure in MG patients. This outcome indicates that protein-related alterations may play a key role in the development of MG.
The ML algorithms classified MG patients and healthy individuals with high accuracy, sensitivity, and specificity, especially when trained on the protein region. They achieved nearly perfect classification scores (approximately 100 %) for all classifiers in the protein region, highlighting the potential of this method for accurate MG diagnosis.
The authors also observed increased lipid peroxidation in MG patients, shown by a significant decrease in the unsaturation index and an increase in saturated lipid concentration. An increase in protein concentration in the serum of MG patients was confirmed by both spectral analysis and biochemical assays.
Additionally, MG patients had higher deoxyribonucleic acid (DNA) and ribonucleic acid (RNA) concentrations, suggesting changes at the gene level. Increased protein phosphorylation, which is important for various physiological processes, was also noted.
The study further identified structural changes in proteins and lipids in MG patients, including reduced lipid dynamics and altered protein structure. The researchers also used hierarchical clustering analysis to evaluate the relationships between the spectral signatures and the clinical data of the patients.
Applications
This research has significant implications for diagnosing and managing MG. ATR-FTIR spectroscopy with ML techniques offers a fast, cost-effective, and non-invasive diagnostic method suitable for clinical settings. This approach could reduce the time and cost of traditional diagnostic methods while improving diagnostic accuracy.
The identified spectral biomarkers could also help monitor disease progression and treatment response, offering valuable insights for personalized medicine. The authors suggested training the ML algorithms on larger datasets to improve their performance and exploring the potential of this method for diagnosing other autoimmune diseases.
Conclusion
The combination of ATR-FTIR and ML algorithms demonstrated significant effectiveness in diagnosing MG, with high classification performance that could revolutionize diagnosis by enabling earlier detection and timely treatment. This approach offers several advantages over current methods, potentially improving clinical practice through earlier diagnosis, improved patient outcomes, and personalized treatment strategies.
Future work should focus on validating these findings with larger patient groups and exploring the method's potential to differentiate between different antibody subtypes in MG. Additionally, developing a spectral database with more MG and healthy control serum spectra could further enhance the diagnostic capabilities of this approach.
More from AZoOptics: Laser Technology's Vital Role in Modern Medicine
Journal Reference
Severcan, F., et al. (2024). Decoding myasthenia gravis: advanced diagnosis with infrared spectroscopy and machine learning. Sci Rep. DOI: 10.1038/s41598-024-66501-3, https://www.nature.com/articles/s41598-024-66501-3
Disclaimer: The views expressed here are those of the author expressed in their private capacity and do not necessarily represent the views of AZoM.com Limited T/A AZoNetwork the owner and operator of this website. This disclaimer forms part of the Terms and conditions of use of this website.