In a recent article in Nature Communications, researchers introduced a novel approach to characterizing the structure and dynamics of large proteins in solution using nuclear magnetic resonance (NMR) spectroscopy. They presented a groundbreaking method that utilizes deep neural networks (DNNs) to transform 13C-1H correlation spectra of uniformly 13C-labeled proteins.
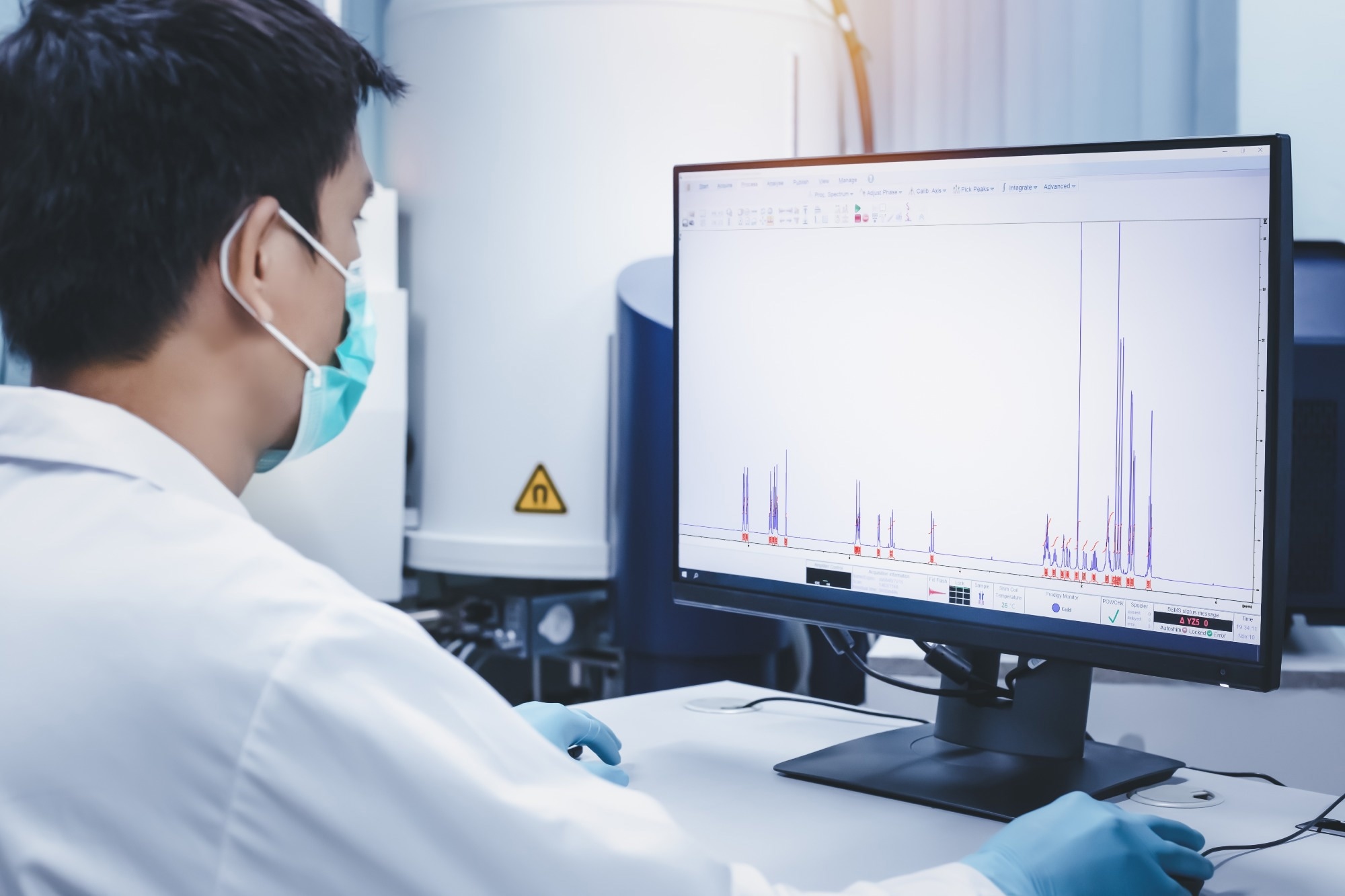
Image Credit: S. Singha/Shutterstock.com
Background
NMR spectroscopy is a powerful tool in materials science, chemistry, structural biology, and clinical diagnosis. It provides unprecedented insights into the functional motions and non-covalent interactions of biomolecules at the atomic level. However, this technique is inherently insensitive, and the size limitations of biomolecular systems have been a persistent challenge.
The methyl transverse relaxation-optimized spectroscopy (methyl-TROSY) approach, which uses methyl-bearing side chains as probes, has significantly expanded the size range accessible to solution-state NMR, allowing the study of systems up to the megadalton molecular weight range.
However, this method requires the protein to be prepared with an extremely high level of deuteration, which can be costly and result in lower protein yields, particularly for eukaryotic and membrane proteins.
About the Research
In this paper, the authors developed a method to characterize large, non-deuterated, uniformly 13C-labeled proteins, overcoming the limitations of the traditional methyl-TROSY approach.
They employed two DNNs, based on the FID-Net architecture they previously developed for other NMR applications. The first DNN was trained to remove the effect of one-bond 13C-13C scalar couplings and sharpen the signals in the 13C dimension, while the second DNN was trained to sharpen the signals in the 1H dimension.
The DNNs were trained and validated on synthetic data generated by simulating realistic NMR spectra with various parameters. The researchers then applied their method to experimental data of three proteins of increasing size; all uniformly 13C-labeled in a protonated background.
They compared the spectra with those obtained by conventional methyl-TROSY methods on deuterated and specifically labeled samples, showing that their method can produce spectra of similar quality with comparable line widths and peak positions.
Research Findings
The results showed that the presented technique could successfully decouple and sharpen the signals in the 13C-1H correlation spectra of uniformly 13C-labeled proteins, making them more easily interpretable and comparable to methyl-TROSY spectra.
The method recovered all the expected peaks from the methyl-TROSY spectra and additional peaks from other methyl groups. It was also extended to three-dimensional NOESY spectra, providing structural information and chemical shift assignments.
Applied to a 3D 13C-HSQC-NOESY-HSQC spectrum of MSG, the researchers observed NOE cross-peaks among the methyl protons within a distance of 3.0 to 5.0 Å of each other. As expected, the NOE cross-peak volumes correlated with the interproton distances.
This method could be generally applied to large proteins and complexes without deuteration, offering more probes for studying biomolecular behavior. Additionally, it provided information on additional methyl groups, such as alanine, threonine, and isoleucine 13Cγ2, which were not accessible by conventional methods.
Applications
This approach has significant potential implications in the field of biomolecular NMR spectroscopy. It enables the study of large proteins that are challenging to produce in bacterial systems, such as eukaryotic and membrane proteins. It also provides comprehensive information on the structure and dynamics of large proteins by accessing all methyl-bearing residues rather than a subset.
Moreover, it reduces the cost and complexity of sample preparation since it requires only uniform 13C labeling instead of perdeuteration and specific labeling. It also facilitates chemical shift assignments and structural characterizations of large proteins, generating high-resolution and sensitive 3D NOESY spectra.
Conclusion
This novel methodology is effective for the structural and dynamic characterization of large proteins. It represents a significant breakthrough in the field of biomolecular NMR.
By overcoming the limitations of traditional methyl-TROSY methods, this technique paves the way for the characterization of large, non-deuterated proteins, expanding the scope of NMR applications and complementing other structural biology techniques, such as cryo-electron microscopy and AI-based predictions.
What is Cryo-Electron Microscopy?
The researchers have demonstrated the feasibility and robustness of this approach, which is expected to have a transformative impact on the study of complex biomolecular systems, ultimately enhancing our understanding of their structure, function, and regulation.
Moving forward, researchers acknowledge limitations and challenges such as peak shape and intensity loss, size limits, and data availability. They suggested developing DNNs for quantitative mapping in relaxation experiments and exploring GAN or transfer learning for NMR spectrum sensitivity and resolution enhancement.
Diversifying synthetic data realism and integrating experimental data into DNN training could boost robustness and generalizability.
Journal Reference
Karunanithy, G., Shukla, VK., Hansen, DF. (2024). Solution-state methyl NMR spectroscopy of large non-deuterated proteins enabled by deep neural networks. Nat Commun. doi.org/10.1038/s41467-024-49378-8
Disclaimer: The views expressed here are those of the author expressed in their private capacity and do not necessarily represent the views of AZoM.com Limited T/A AZoNetwork the owner and operator of this website. This disclaimer forms part of the Terms and conditions of use of this website.