A nanosecond-scale volatile modulation scheme, including a phase-change material, was developed by scientists. This was undertaken as a step in the development of optical computing.
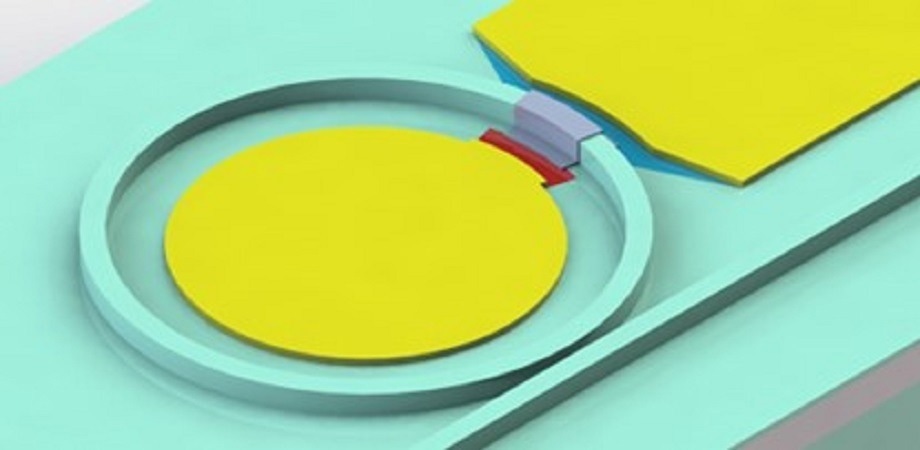
New approach to photonic memory integrates a phase-change material to enable in-situ training of optical neural networks. Image Credit: Maoliang Wei and Kai Xu
Technological advances like computer vision and autonomous driving are driving a surge in demand for computational power.
Optical computing, with its energy efficiency, high throughput, and low latency, has attracted significant attention from industry and academia. But present optical computing chips available experience limitations in power consumption and size, which stops the scalability of optical computing networks.
As a result of the increase in nonvolatile integrated photonics, optical computing devices have the potential to obtain in-memory computing while functioning with zero static power consumption.
For obtaining photonic memory and nonvolatile neuromorphic photonic chips, phase-change materials (PCMs) have become promising candidates. PCMs provide high refractive index contrast between various states and reversible transitions, thereby making them perfect for large-scale nonvolatile optical computing chips.
While the guarantee of nonvolatile integrated optical computing chips is tantalizing, it comes with a number of difficulties. The requirement for quick and frequent switching, needed for online training, is an obstacle that scientists are determined to overcome. Making a path towards rapid and efficient training is a crucial step on the journey to the free the complete potential of photonic computing chips.
Recently, a discovery by scientists from Zhejiang University, Westlake University, and the Institute of Microelectronics of the Chinese Academy of Sciences was achieved.
As reported in the journal Advanced Photonics, the researchers came up with a 5-bit photonic memory with the potential of quick volatile modulation. They suggested a solution for a nonvolatile photonic network assisting rapid training.
This was made feasible by combining the low-loss PCM antimonite (Sb2S3) into a silicon photonic platform.
The photonic memory makes use of the carrier dispersion effect of a PIN diode to obtain volatile modulation with a quick response time of under 40 ns, thereby maintaining the stored weight information.
Once the training is done, the photonic memory makes use of the PIN diode as a microheater to allow multilevel and reversible phase changes of Sb2S3, thereby enabling the storage of trained weights in the photonic computing network. This results in an unbelievably energy-efficient photonic computing process.
The research group simulated an optical convolutional kernel architecture using the illustrated photonic memory and working principle. They obtained more than 95% precision in identifying the MNIST dataset.
This displays the possibility of quick training via weight storage and volatile modulation via 5-bit non-volatile modulation.
This innovative work fixes a new paradigm for photonic memory and provides an encouraging solution for performing non-volatile devices in fast-training optical neural networks. With such progress, the future of optical computing looks brighter than ever before.
Journal Reference
Wei, M., et al. (2023) Electrically programmable phase-change photonic memory for optical neural networks with nanoseconds in situ training capability. Advanced Photonics. doi.org/10.1117/1.AP.5.4.046004
Source: https://spie.org/?SSO=1