Reviewed by Mila PereraOct 28 2022
Our lives have undoubtedly been simpler and more productive due to artificial intelligence (AI), which was created to replace traditional industrial reasoning through quick learning and inference. As a representative of machine learning techniques, the artificial neural network (ANN) has the broadest range of uses for resolving issues in the real world.
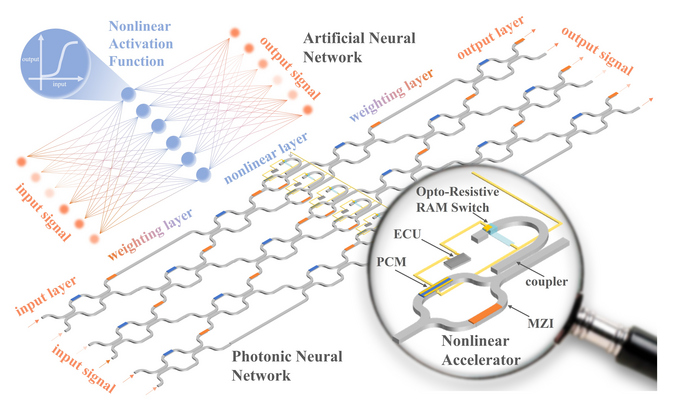
The schematic structure of photonic neural network integrated with nonlinear accelerator, which generates different nonlinear activation functions by programming. (ECU: Electrical Control Unit; PCM: Phase Change Material; MZI: Mach–Zehnder Interferometer). Image Credit: Zefeng Xu
Despite being accelerated by Graphics Processing Units (GPU), Application-Specific Integrated Circuits (ASIC), and Field-Programmable Gate Array (FPGA) chips, ANN applied in the real world is constrained by low calculation speed, high energy consumption, and a few computing parallelisms.
According to one tutorial, “Rise of the learning machines” from Nature Photonics, “Photonic neuromorphic computation appears set to offer huge opportunities” to address this problem.
However, as Professor Aydogan Ozcan, Chancellor’s Professor at the University of California, Los Angeles (UCLA), emphasized in his Q&A titled “Machine learning with light,” the implementation of effective nonlinear activation functions is a significant obstacle to successful photonics integration.
Challenges do exist for all-optical implementations due to lack of highly efficient and practical nonlinear optical processes that can serve as activation functions for large numbers of nodes.
Aydogan Ozcan, Chancellor’s Professor, University of California, Los Angeles
As a result, numerous research projects have been created in an effort to find an effective solution for photonic nonlinear activation functions.
A group of researchers from the Department of Electrical and Computer Engineering at the National University of Singapore (NUS), Singapore, led by Professor Aaron Voon-Yew Thean, Dean of the College of Design & Engineering at NUS, have developed a novel photonic neural network accelerator using a non-volatile Opto-Resistive RAM Switch to attain programmable nonlinear activation functions.
The innovative Opto-Resistive RAM Switch, a coupler, a Mach-Zehnder interferometer with phase shifters in both arms, and an electrical control unit make up the optical-electrical hybrid architecture of the photonic neural network accelerator.
The main accelerator component, the MoS2-based Opto-Resistive RAM Switch, was initially developed and refined to provide outstanding linearity between input optical power and switching voltage.
Additionally, the Opto-Resistive RAM Switch can be used due to its nonlinear switching, circumventing the constrained linear response in typical photonic components such as conventional photodetectors. For the first time, the photonic neural network also uses two-dimensional material.
The accelerator achieves superior programmability when combined with finely adjusted phase shifters and an electrical control unit. This allows it to be used to accurately represent various nonlinear activation functions, such as sigmoid, softplus, and clamped Rectified Linear Unit (ReLU). These play a crucial role in the acceleration of ANN.
On an MNIST handwritten digit recognition task, the accelerator has been successfully demonstrated in an in-situ, full photonic neural network. Comparing the newly built accelerator to other advanced designs, the team achieved a significant accuracy of 91.6%, drastically lowered average power consumption by up to 20 times, and reduced the hardware footprint by nearly 40%.
The reported architecture offers new pathways for developing in-situ photonic neural networks for future edge AI, smart cities, automated driving, and supercomputer due to its extremely high efficiency and computing density.
There is a search for more efficient optoelectronic devices to enable photonic neuromorphic computing. The key components are non-volatile analog switch and thresholding device. Here is a perfect proposal of devices with novel materials that can enable them.
Aaron Voon-Yew Thean, Dean, College of Design & Engineering, National University of Singapore
Zefeng Xu, a graduate student at NUS and a member of Professor Thean’s lab who is concurrently pursuing a Ph.D. and an MBA, is the study’s first author.
Photonics could revolutionize artificial intelligence and motivate a huge emerging market.
Zefeng Xu, Study First Author and Graduate Student, National University of Singapore
Journal Reference
Xu, Z., et al. (2022) Reconfigurable nonlinear photonic activation function for photonic neural network based on non-volatile opto-resistive RAM switch. Light Science & Applications. doi:10.1038/s41377-022-00976-5.