In-season detection of corn grains results in field variability and enhanced nitrogen (N) management. To forecast in-season yield potential, a recent study published in Agronomy compares multiple optical sensors and vegetation indices (VIs) at various corn grain stages. These stages include the normalized difference red edge (NDRE), normalized difference vegetation index (NDVI), and simplified canopy chlorophyll content index (SCCCI).
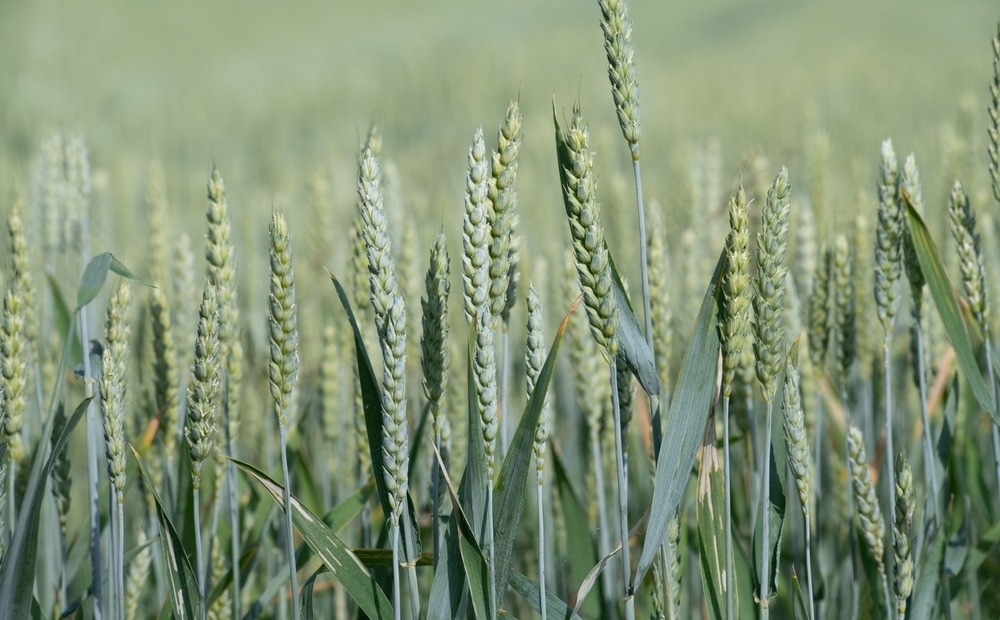
Study: Predicting In-Season Corn Grain Yield Using Optical Sensors. Image Credit: leopictures/Shutterstock.com
Researchers chose eight sites for field trials in Mississippi. GreenSeeker, SPAD sensor, Crop Circle, and unmanned aerial system (UAS) equipped with a MicaSense optical sensor and two proximal optical sensors were used to gather data on crop reflectance.
Yield Goals Result in Low Nitrogen Recovery
Crop Grain yields increase by 30–50% due to nitrogen treatment. Nitrogen treatment sustains a greater population and fosters economic growth. The majority of nitrogen fertilizers used in the US nowadays are utilized to grow corn. Only half of the nitrogen applied is recovered. Lost nitrogen results in agricultural nitrogen pollution.
Using ineffective techniques, such as yield objectives for nitrogen detection contributes to low nitrogen recovery. Mississippi State recommends using the crop yield goal (CYG) strategy. CYG Method is inefficient in Mississippi due to geographical and temporal variabilities.
Non‐Destructive Canopy Reflectance Sensing as an Alternative to CYG Method
Non-destructive canopy reflectance sensing is an alternative to CYG-based nitrogen management. Numerous significant mechanisms in plants depend on nitrogen. Nitrogen is needed in much larger amounts compared to other nutrients. The chemical or physical characteristics of plants show a deficit in nitrogen.
Crop canopy sensors accurately account for nitrogen shortages since they cause plants to be less chlorophyll-rich and green. Multiple vegetation indices (VIs) are used based on variations in crop reflectance to define geographical and temporal N variability.
In-season Crop Grain Yield Detection Based on Optical Sensors
In-season detection of crop grain yield provides a unique environmental nitrogen rate. In-season detection reduces temporal and spatial variability. The ability to forecast in-season yield potential using an algorithm built from on-site data is a prerequisite for developing a nutrient management strategy based on optical sensor technologies. The capacity to discern differences in crop nitrogen uptake by employing distinctions in vegetative indices (VI) allows for the computation of in-season yield potential.
Optical Sensor‐Based Datasets and Corn Grain Correlations
Corn growth stage, vegetative indices, and optical sensors are necessary for accurate in-season corn grain yield prediction. Vegetative and reproductive stages of corn growth are often separated with V1. V1 designates the one-leaf stage up until tasseling which is labeled as VT. Similar to this, R stages start at R1 based on corn kernel development and end at R6 with a mature and ready harvest crop. The V7 to V9 growth stages are the strongest correlation between NDVI and corn grain yields. V7 is the best stage for predicting grain yield. No statistically significant differences exist between the GreenSeekerTM (Trimble Inc., Sunnyvale, CA, USA) and Crop CircleTM ACS430 (Holland Scientific, Lincoln, NE, USA)optical sensors when predicting corn grain yield using an NDVI-derived INSEY technique at the V6 stage.
Investigation of Optical Sensor‐Based Nitrogen Management Applicability
The use of NDRE improves the connection between INSEY and corn grain yield because the red edge wavelength is less affected by saturation effects at later growth stages. Additionally, there is proof that the simplified canopy chlorophyll content index (SCCCI), which contains both NDVI and NDRE is more accurate at predicting grain yield than either NDVI or NDRE.
Oglesby et al. thoroughly evaluated the applicability of optical sensor-based nitrogen management in light of the interconnected elements influencing in-season yield forecast. Analyzing the capacity to forecast yield potential using various optical sensors and VIs at multiple growth phases allowed the nitrogen management assessment. The researchers also assessed the most effective technique for predicting grain yields using VI data. The inferences used to develop an algorithm that could precisely forecast Mississippi's nitrogen needs similar to those developed for other regions were also studied.
Research Findings
This study evaluated four optical sensors and three vegetative indices at various corn grain development stages. There were differences in the ability to estimate corn grain yield between the various vegetative indices, optical sensors, and stages. More specifically, SCCCI and later growth stages predicted corn grain yield most accurately. SPAD optical sensor was best suited for predicting corn grain yields.
The VI data approach was also investigated in this study, and significant variance was discovered. The variance caused by methodological variations emphasized the necessity of evaluating the VI approach while developing future corn grain yield prediction models. The practicality should be considered when an algorithm's end objective is commercial employment.
Reference
Oglesby, C., Fox, A. A. A., Singh, G., & Dhillon, J. (2022) Predicting In-Season Corn Grain Yield Using Optical Sensors. Agronomy, 12(10), Article 10. https://www.mdpi.com/2073-4395/12/10/2402
Disclaimer: The views expressed here are those of the author expressed in their private capacity and do not necessarily represent the views of AZoM.com Limited T/A AZoNetwork the owner and operator of this website. This disclaimer forms part of the Terms and conditions of use of this website.