A study published in Remote Sensing used multi-temporal remote sensing optical imaging to investigate the deformation characteristics of landslides and their evolution pattern. The analysis and identification of landslide hazards contribute to their prevention and provide a foundation for the early detection and investigation of similar incidents.
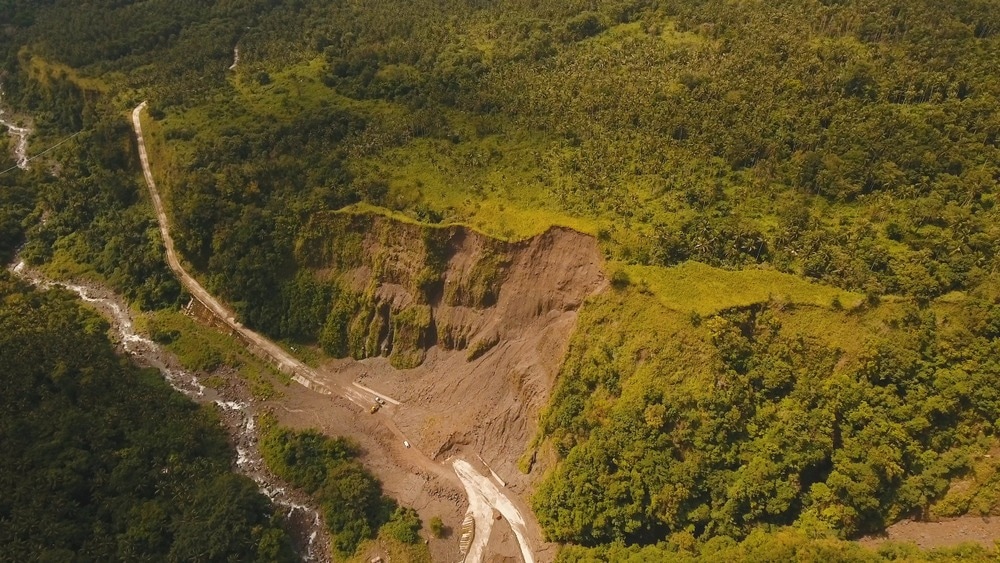
Study: Comprehensive Remote Sensing Technology for Monitoring Landslide Hazards and Disaster Chain in the Xishan Mining Area of Beijing. Image Credit: Alex Traveler/Shutterstock.com
Landslides: A Geological Hazard
Landslides are one of the most common and dangerous geological disasters on Earth. Human engineering activities and extreme climatic conditions have led to an increase in landslide frequency.
Landslides threaten residents' safety and property due to their abrupt nature, quick movement, and strong concealment.
Landslide identification is critical for assessing and managing landslide risk. Early detection of landslides can significantly reduce possible casualties. With the development of remote sensing technology, they can be identified by visually interpreting topographic surfaces and remote sensing images.
Remote Sensing Technology for Landslide Hazard Identification
In recent years, remote sensing imaging has grown more prevalent, and associated technologies such as multi-temporal high-resolution optical image analysis technology and interformetric synthetic aperture radar (InSAR) have seen substantial applications in geological hazard identification.
Multi-temporal remote sensing technology
Multi-temporal, high-resolution optical images are ideal for identifying geological hazards with visible deformation because of their extensive geographical coverage and abundant storage.
InSAR technology
In regions with strong surface coherence and modest deformation rates, InSAR technology excels in identifying geological disasters. It is also suitable for detecting large-scale geological disasters.
Study Area: Xishan Mining Area
The Xishan coal mines in Beijing, China, have been exploited for almost 1,000 years until 2008 when all coal mines were closed. However, the stability of the mountain was already affected by underground mining, particularly due to the operations of some minor coal businesses that included shallow mining depths, high mining intensities, and high disturbance, resulting in landslides and other geological hazards.
Landslides and their induced hazard chains are highly catastrophic in the mountain region, yet they are difficult to detect due to strong concealment.
Beijing is China's cultural and political center, and the Xishan mining district is highly inhabited. However, few researchers have investigated the landslides in this region; therefore, it is crucial to identify geological and other potential hazards.
Using Optical Imaging and InSAR for Landslide Evolution Pattern and Hazard Identification
In the study area, 19 landslides and 32 collapses were investigated. Researchers collected multi-temporal remote sensing observations from the Xishan mining region and identified landslides by extracting deformation data from time-series InSAR and optical images.
They extracted the location of collapses and tension cracks induced by the lower slope swelling and estimated the changes in geomorphology, vegetation, and slope. SAR data and remote sensing images were compared to differentiate the texture and tone between landslides and their surrounding areas.
Finally, researchers categorized the landslide evolution pattern and examined the hazard chain's triggering elements and disaster patterns.
Significant Findings of the Study
The four stages of the development of the landslides observed in the Xishan mining region include initial deformation, rupturing and joining of the rear portion, slope front swelling and collapsing, and landslide creeping. The complete evolution process takes more than ten years. During these stages, surface deformation was evident.
Landslides are classified into three categories based on their development strata, sliding properties, and deformation stage.
The first type is triggered by the collapse of a high-level rock mass. It forms in the coal-bearing stratum's upper layers. This landslide continues to distort after its collapse. The rupture of goaves causes the second and third types of landslides.
The second kind occurs mainly in the underlying layers of coal-bearing strata. The majority of these landslides are at the early deformation stage. The third type develops in coal-bearing strata. These landslides are at the creeping stage and deform much faster than the second type.
The precipitation and topography of the Dongjianggou and Anzigou ditch are conducive to debris flows. Therefore, most landslides in this region are vulnerable to a goaf-landslide-debris flow hazard chain in the case of heavy rain.
Surface and subsurface disturbances in a goaf can be severe, and their underground systems are complicated. In these places, landslides and chain catastrophes can occur in addition to ground subsidence and fissures.
Identifying areas with geological hazard risks using multi-platform remote sensing technologies is crucial to reduce and prevent the damage caused by land sliding and other geological hazards in these areas. Moreover, remote sensing can be accompanied by a comprehensive monitoring system that collects and verifies the data using spaceborne, airborne and ground observations.
Reference
Jiao, R., Wang, S., Yang, H., Guo, X., Han, J., Pei, X., & Yan, C. (2022). Comprehensive Remote Sensing Technology for Monitoring Landslide Hazards and Disaster Chain in the Xishan Mining Area of Beijing. Remote Sensing. https://www.mdpi.com/2072-4292/14/19/4695/htm
Disclaimer: The views expressed here are those of the author expressed in their private capacity and do not necessarily represent the views of AZoM.com Limited T/A AZoNetwork the owner and operator of this website. This disclaimer forms part of the Terms and conditions of use of this website.