Mar 9 2021
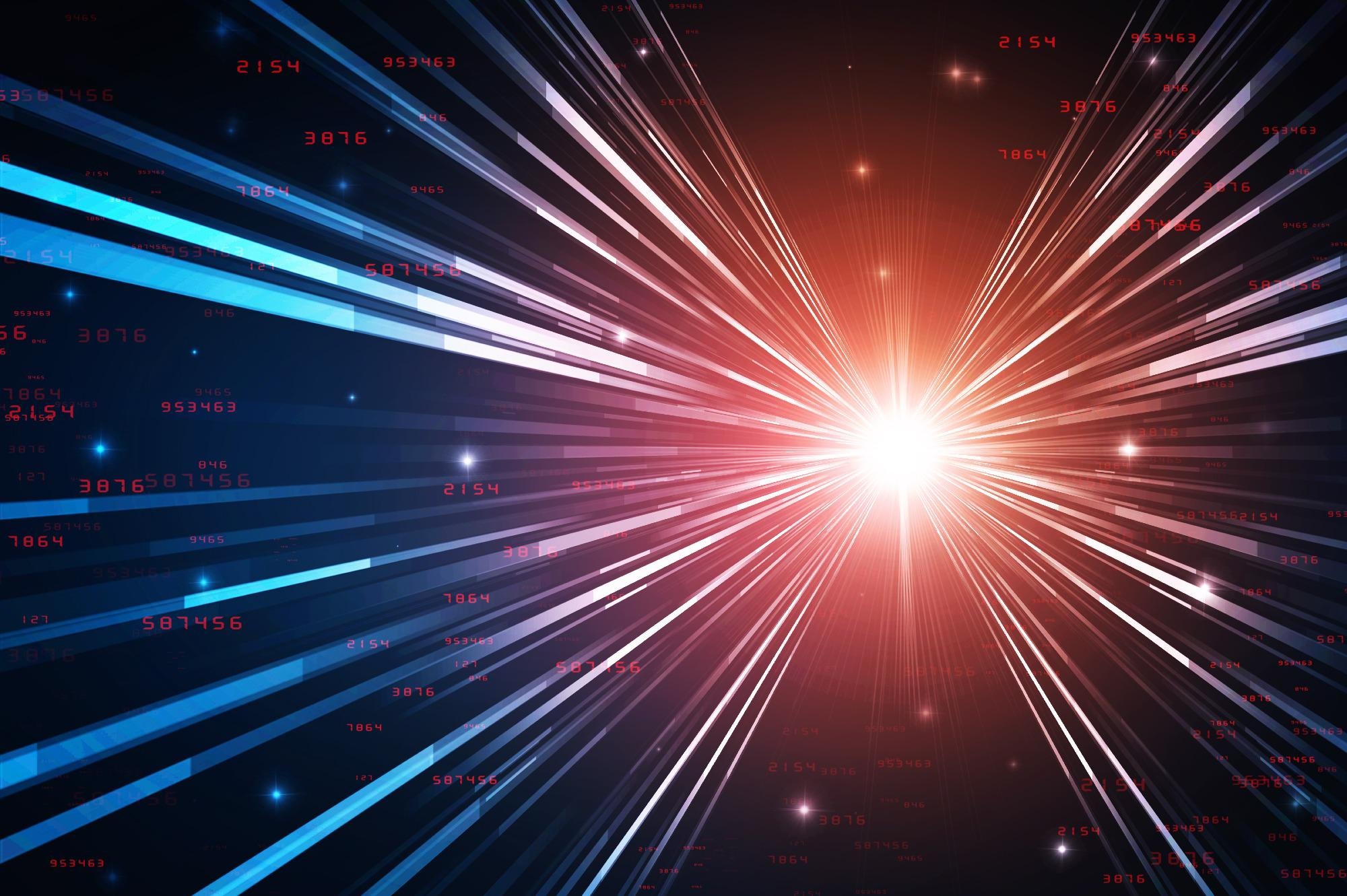
Image Credit: ranjith ravindran/shutterstock.com
Associate Professor Andrea Fratalocchi from KAUST has headed a group of researchers and found that silicon nanoshapes serve as feed-forward neural networks that can be trained in a supervised learning model to carry out user-defined tasks at the speed of light.
The new flat optics paves the way for a significant technological revolution by providing compact, low-cost, versatile alternatives to existing processors and for a completely new generation of devices.
Published in Light: Science & Applications, the new study by the group headed by Professor Andrea Fratalocchi from Primalight Laboratory of the Computer, Electrical and Mathematical Sciences and Engineering (CEMSE) Division, King Abdullah University of Science and Technology (KAUST), Saudi Arabia, described a new patented, scalable flat-optics technology made using low-cost semiconductors.
The KAUST-designed technology exploits a previously unidentified aspect of optical nanoresonators, shown to have a physical layer completely equivalent to a feed-forward deep neural network.
What we have achieved is a technological process to cover flat surfaces, which in optical jargon are called ‘flat optics’, with ‘physical’ neural units that are able to process light as a neural network does with an electrical signal.
Andrea Fratalocchi, Professor, Primalight Laboratory, Computer, Electrical and Mathematical Sciences and Engineering Division, King Abdullah University of Science and Technology
These novel flat optics realize near unity efficiencies (of up to 99%) in the visible range in ultrathin surfaces, thus offering vectorial and broadband light control in both transmission and reflection with the preferred wavefront shape.
The nanoshape silicon surface is also ultrathin (with a thickness of 60 nm, where 1 nm = 1/1,000,000 of 1 mm) and can be tailored on flexible surfaces.
The program employed to develop the nanosurface runs on the Shaheen-II supercomputer at KAUST, which is a Cray XC40 that delivers more than 7.2 Pflop/s of theoretical peak performance, and is performed with the Autonomous Learning Framework for Rule-based Evolutionary Design software created by Fratalocchi and his colleagues.
We have developed a program that uses artificial intelligence to design the nanoresonators. The algorithm works using evolutionary techniques: in simple terms, the algorithm is able to train itself and improves its results after every cycle to produce surfaces of increasing efficiency every time that it is run.
Andrea Fratalocchi, Professor, Primalight Laboratory, Computer, Electrical and Mathematical Sciences and Engineering Division, King Abdullah University of Science and Technology
“In our article, we showed experimental components with better performance than the current state of the art in flat-optics or from commercial devices available from leading companies, such as Thorlabs and Newport,” added Fratalocchi.
At present, the KAUST team is planning to use flat optics to create new flat devices that could completely transform older technologies that are based on bulk optics.
Among the novelties, Fratalocchi and his colleagues are developing a human-eye camera, a biosensor capable of 'reading' cells infected with malaria, and displays of new kinds.
“There are really endless applications ,” explained Fratalocchi, “because almost all existing measurement systems, in principle, could be substituted by their cost-effective, compact flat-optics versions.”
Fratalocchi continued, “We are developing a statistical learning approach that, for any given measurement task, designs a corresponding flat-surface that ‘encodes’ the measure into a single optical image, or logogram. With this approach, the entire field of sensing and metrology could become natural language processing based on nonlinear logograms.”
“One of our current projects is a flat camera that can see even better than the human eye, which is limited by using only three primary receptors for color vision. We can also miniaturize any component, no matter how bulky,” he added.
The key concept here is that a neural network is a universal approximator that can learn any function. For this reason, we can train our flat optics to perform any task, or a sequence of tasks currently performed by electronic systems, just in less space and at the speed of light.
Andrea Fratalocchi, Professor, Primalight Laboratory, Computer, Electrical and Mathematical Sciences and Engineering Division, King Abdullah University of Science and Technology
“With proper funding and resources, in five to 10 years’ time, most of today’s bulky technology will have shrunk to pocket size, with a similar revolution that electronics experienced at the end of the last century,” Fratalocchi concluded.
Journal Reference:
Getman, F., et al. (2021) Broadband vectorial ultrathin optics with experimental efficiency up to 99% in the visible region via universal approximators. Light: Science & Applications. doi.org/10.1038/s41377-021-00489-7.