In an article published in Remote Sensing, researchers examined the feasibility of ZY1-02D hyperspectral images for extracting non-optical active water quality parameters, such as dissolved oxygen (DO), total phosphorus (TP), and permanganate index (CODMn), in urban rivers and lakes.
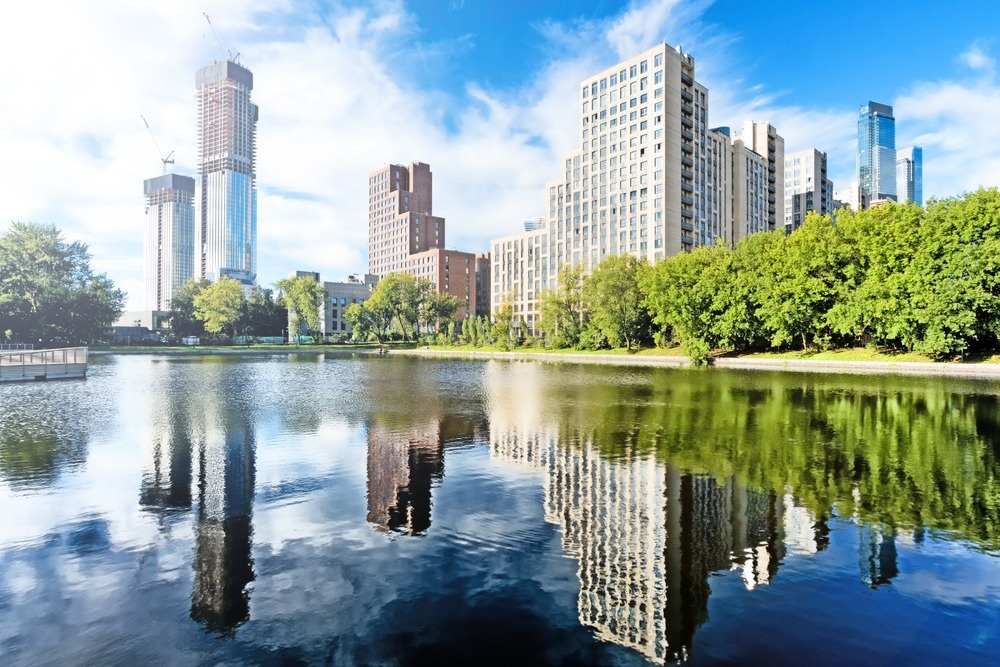
Study: Water Quality Retrieval from ZY1-02D Hyperspectral Imagery in Urban Water Bodies and Comparison with Sentinel-2. Image Credit: vaalaa/Shutterstock.com
Applying Sentinel-2 and ZY1-02D spectral response functions, the authors simulated the reflectance observed in situ to the satellite equivalent reflectance. The retrieval performance of the ZY1-02D and Sentinel-2 sensors was compared with various bandwidths using four machine-learning models. For TP, CODMn, and DO, the mean absolute percentage errors (MAPE) were 37.04%, 18.44%, and 24.28%, respectively. While the corresponding root mean square errors (RMSE) were 0.07, 0.96, and 1.67 mg/L.
The consistency and precision of aquatic products extracted from Sentinel-2 and ZY1-02D images were then verified. Compared to Sentinel-2 remote sensing reflectance (Rrs), the ZY1-02D reflectance outputs were marginally exaggerated.
When mapping CODMn products in urban water, ZY1-02D showed excellent precision and consistency. The findings highlighted ZY1-02D hyperspectral images' potential for mapping non-optically active parameters of urban water quality.
Advantages of Remote Sensing Techniques in Urban Water Quality Detection
Water resources are essential for various city activities, including landscape, industrial production, and drinking water. A significant portion of urban water bodies is rivers and lakes. However, the rapid enhancement of urban culture and economy has resulted in the growth of living standards.
As a result, the amount of residential, agricultural, and industrial water discharged exceeds the capacity of water bodies to self-cleanse, seriously polluting urban rivers and lakes. Loss of ecological integrity and drinking water safety are consequences of declining urban water quality, which also impacts biodiversity and human health.
An essential component of assessing water quality is water quality monitoring. The traditional methods for monitoring water quality, such as manual field sampling, laboratory analysis, or automatic in situ measurements, offer high levels of accuracy. However, these manual methods are labor-intensive, and the automatic station demands substantial costs for both building and maintenance. Remote sensing has been utilized extensively since the 1970s to track the temporal and spatial features of urban water quality.
DO, CODMn, and TP are crucial chemical indicators for monitoring water quality. Due to their inability to be readily estimated from spectral features and the fact that they do not absorb light, these are known as non-optically active parameters. DO represents the amount of oxygen in the water and is influenced by Chlorophyll-a and algae.
The amounts of organic contaminants in water are represented by CODMn, the quantity of potassium permanganate oxidant used to treat water samples. Several of these significant indices of urban water quality have been measured using indirect methods for remote sensing. The remote sensing techniques are based on the strong association between the optically active and the non-optically active parameters.
The authors employed an in situ observation modeling method in this study to extract the urban water quality metrics from Sentinel-2 and ZY1-02D images. Four machine learning methods were used to calibrate and train models between concentrations and simulated in situ reflectance. The in situ observation-based inversions of urban water quality metrics required accurate atmospheric correlation. In contrast, a precise atmospheric correction was unnecessary for approaches that relied on image-derived data.
The remote sensing reflectance and water quality products produced from ZY1-02D were compared with those from Sentinel-2. The ZY1-02D and the Sentinel-2 sensors' comparisons showed the ZY1-02D hyperspectral imagery's excellent capacity for extracting urban water quality metrics.
Experimental Set-Up
Twelve field measurements of the remote sensing reflectance were made in 90 Shanghai rivers between September 2018 and November 2021. The remote sensing reflectance from the river bottom could not alter the Rrs readings of surface water since the average transparency of these rivers was less than 0.6 m. Several rivers were measured at various times and locations to define the link between optically active and non-optically active parameters.
Multispectral data was employed from a Sentinel-2 with a spatial resolution of 10–60 m. 13 bands and short temporal resolution were features of the Multispectral Instrument (MSI) onboard the Sentinel-2 (A/B). The Chinese ZY1-02D satellite's Advanced HyperSpectral Imager (AHSI) collected hyperspectral data together with concordant Sentinel-2 images.
The correlation between the urban water quality metrics and the satellite equivalent remote sensing reflectances was described using the Pearson-based correlation analysis. Between -1 and +1 was the range of the Pearson correlation coefficient (r). A positive correlation or significant inverse between the variables was shown when the r value was close to +1 or -1, respectively. However, the r-value around zero showed no association between the variables.
Waterbody extent extraction, atmospheric correction (AC), and remote sensing reflectance assessment were significant steps in preprocessing satellite images. First, the digital number values were converted to top-of-atmosphere radiances using the radiometric calibration coefficients of the AHSI. Second, the bottom of the atmosphere reflectance product of the Sentinel-2 Level 2A was generated using the Sen2Cor processor.
The findings revealed that the relationship between the spectral reflectance between 700 nm and 400 nm and the DO content was inverse. The spectral reflectance peak at 705 nm and trough at 675 nm was more prominent when the DO content was more significant. Similarly, the concentration of CODMn was negatively correlated with the reflectance between 400 nm and 700 nm. When CODMn levels were more than three mg/L, the spectral reflectance from 700 to 900 nm was identical.
Significance of the Study
In this study, the authors investigated the inversion of non-optically active parameters of urban water quality from the hyperspectral ZY1-02D data at rivers and lakes in Shanghai. The study used an in situ observation modeling approach based on machine learning. Due to the greater spectral resolution of ZY1-02D, the findings showed that its machine-learning models performed better than those of Sentinel-2.
Assessments conducted on the models' applicability in various temporal and spatial contexts revealed that the models based on in situ data from Shanghai could be applied to Taihu Lake. The Rrs and water quality products obtained from ZY1-02D were compared with those derived from Sentinel-2 images to evaluate their precision and consistency. Rrs data comparisons revealed significant agreement in bands with high energy. More agreement was seen between the CODMn products than between DO and TP.
The field-based models were used for a ZY1-02D image that was atmospherically corrected. Overall, the results highlighted the significant potential of ZY1-02D hyperspectral images for aquatic-focused applications. However, retrieving accurate non-optically active parameters of urban water quality metrics was still challenging, and more research was required. Future research would investigate precise AC pre-processing techniques for ZY1-02D images regarding quantitative urban water quality.
Reference
Yang, Z., Gong, C., Ji, T., Hu, Y., Li, L. (2022) Water Quality Retrieval from ZY1-02D Hyperspectral Imagery in Urban Water Bodies and Comparison with Sentinel-2. Remote Sensing, 14(19), 5029. https://www.mdpi.com/2072-4292/14/19/5029/htm
Disclaimer: The views expressed here are those of the author expressed in their private capacity and do not necessarily represent the views of AZoM.com Limited T/A AZoNetwork the owner and operator of this website. This disclaimer forms part of the Terms and conditions of use of this website.