By Ankit SinghReviewed by Susha Cheriyedath, M.Sc.Jul 8 2024
Hyperspectral cameras are advanced imaging devices that capture and process information across a wide range of wavelengths in the electromagnetic spectrum.
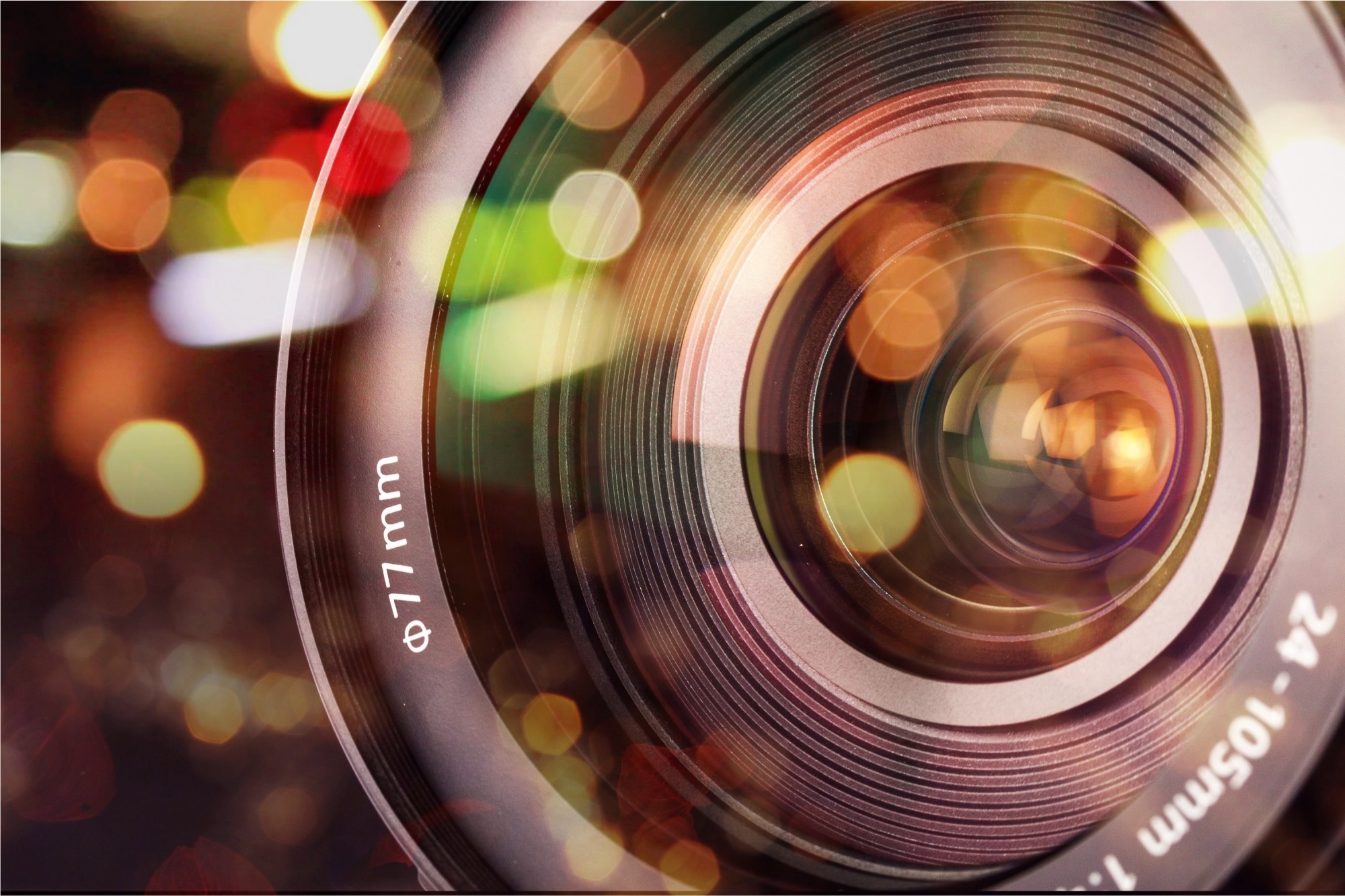
Image Credit: Billion Photos/Shutterstock.com
Unlike standard cameras that capture images using three primary color channels, hyperspectral cameras can record images composed of hundreds of continuous spectral bands. This functionality enables a more detailed examination of the materials and objects in a scene based on their unique spectral characteristics.
Historical Background
The development of hyperspectral cameras can be traced back to the late 1970s and early 1980s with the advent of imaging spectrometry. The initial systems were bulky and complex, primarily used in remote sensing applications by NASA and other research institutions. The foundational work on these early hyperspectral imaging systems paved the way for the subsequent miniaturization and technological advancements in this field.1
In the 1990s, significant improvements in sensor design and data processing capabilities occurred. The development of pushbroom scanners, which use a line of detectors to capture spectral information as the sensor moves across a scene, marked a significant milestone. These systems featured high spatial and spectral resolution, making hyperspectral imaging more practical for various applications.1
The 21st century has witnessed a rapid evolution in hyperspectral camera technology. Advances in detector materials, such as indium gallium arsenide (InGaAs) and mercury cadmium telluride (MCT), have enhanced the sensitivity and range of hyperspectral sensors. Moreover, integrating advanced computing technologies has facilitated real-time data processing, enabling more widespread use of hyperspectral cameras in fields like agriculture, medical diagnostics, and environmental monitoring.1
How Do Hyperspectral Cameras Work?
Hyperspectral imaging operates on the principle that different materials reflect, absorb, and emit electromagnetic radiation differently. Hyperspectral cameras capture and analyze a wide spectrum of light across many narrow wavelength bands. This comprehensive spectral information allows for the identification and differentiation of materials based on their unique spectral signatures.2
Understanding the operational mechanism of hyperspectral cameras requires an in-depth exploration of their key components, the underlying process of hyperspectral imaging, and the methodologies utilized for data analysis.
Components of Hyperspectral Cameras
A typical hyperspectral camera consists of the following key components:
Optical System: This includes lenses and mirrors that collect light from the scene and focus it onto the dispersive element. The spatial resolution of the captured images depends on the quality of the optical system.
Dispersive Element: This is typically a prism or a diffraction grating that separates the incoming light into its constituent wavelengths. The dispersive element plays a crucial role in defining the camera's spectral resolution.
Detector Array: Hyperspectral cameras contain an array of detectors to capture dispersed light. These detectors are sensitive to specific wavelength ranges and are arranged to allow simultaneous capture of multiple spectral bands. Common detector materials include silicon (for visible light) and InGaAs or MCT (for infrared wavelengths).
Data Acquisition System: This system converts the analog signals from the detector array into a digital format that can be processed by a computer. It typically includes analog-to-digital converters (ADCs) and high-speed data transfer interfaces.
Data Processing Unit: The raw data captured by the detector array is processed to create a hyperspectral data cube. This unit uses advanced algorithms to correct for sensor noise, perform calibration, and extract meaningful information from the spectral data.
Hyperspectral Imaging Process
The process of hyperspectral imaging involves the collection, dispersion, capture, and processing of light to generate hyperspectral images, which can be broken down into the following steps:
Light Collection and Dispersion: The optical system collects light from the scene and directs it towards the dispersive element. The dispersive element separates the light into its constituent wavelengths, spreading them across the detector array.
Image Capture: The detector array captures the dispersed light, with each detector element corresponding to a specific wavelength band. This enables simultaneous capture of multiple spectral bands for each pixel in the scene.
Data Cube Formation: The captured data is organized into a three-dimensional hyperspectral data cube. The data cube has two spatial axes (x and y) corresponding to the scene and one spectral dimension (λ) corresponding to the wavelength bands. Each voxel (volumetric pixel) in the data cube contains spectral information for a specific point in the scene.
Calibration and Correction: The raw hyperspectral data is corrected for sensor noise, distortions, and environmental factors. This step often involves dark current subtraction, flat-field correction, and wavelength calibration to ensure the accuracy and consistency of the spectral data.
Data Analysis and Interpretation: The processed hyperspectral data is analyzed to extract meaningful information.
This process involves three key steps. First, spectral unmixing converts each pixel's spectral signature into its constituent components, allowing the identification of different materials in a mixed pixel.
The pixel spectral signatures are then compared to a library of known spectral signatures to identify and classify materials.
Finally, Principal Component Analysis (PCA) improves visualization and interpretation by reducing the dimensionality of the hyperspectral data and preserving the most significant spectral information.
Machine Learning Algorithms: Advanced machine learning (ML) techniques like support vector machines (SVM) and neural networks are used to automate and refine hyperspectral data analysis. These algorithms can identify intricate patterns within the spectral data, enhancing the accuracy and efficiency of material identification and classification processes.
Applications of Hyperspectral Imaging
Hyperspectral imaging significantly enhances precision agriculture by enabling detailed monitoring of crop health, soil properties, and pest infestations. This technology allows for early detection of issues like nutrient deficiencies and diseases, leading to targeted interventions and improved crop management.3
In environmental monitoring, hyperspectral cameras provide insights into land cover changes, water quality, and pollution levels. They are used to map vegetation types, monitor deforestation, and assess aquatic ecosystem health, aiding in environmental conservation and climate change research.4
In the medical field, hyperspectral imaging aids in non-invasive diagnostics and surgical guidance. It helps detect conditions like cancer and diabetic retinopathy by analyzing tissue composition and blood oxygenation levels, enhancing early diagnosis and treatment precision.5
Industrially, hyperspectral cameras are used for quality control and material sorting. They detect contaminants in food, assess pharmaceutical quality, and identify manufacturing defects. This technology improves accuracy and efficiency in various industries, including waste management and recycling.6
Advancements in Miniaturization and Portability
One of the significant trends in hyperspectral camera technology is the miniaturization and development of portable systems. Improved microelectronics and sensor fabrication have led to the development of compact hyperspectral cameras that can be mounted on drones or handheld devices.7
These portable systems expand the range of applications, from in-field agricultural monitoring to on-the-go medical diagnostics. Miniaturized hyperspectral cameras are also being developed for smartphone integration, potentially bringing this advanced imaging technology to the consumer market.7
Real-time Data Processing and ML Integration
Integrating ML algorithms with hyperspectral imaging enhances the real-time analysis capabilities of these cameras. Trained ML models can identify intricate patterns in spectral data, enabling quicker and more precise detection of specific materials or conditions.8
Recent studies have demonstrated the effectiveness of deep learning techniques in classifying hyperspectral images. It has significantly improved the accuracy of crop disease detection and mineral exploration. The synergistic integration of hyperspectral imaging and artificial intelligence is opening new pathways for developing more automated and intelligent decision-making frameworks.8
Advantages and Limitations
More from AZoOptics: Hyperspectral vs Multispectral Imaging: Advantages and Disadvantages
Hyperspectral cameras offer several advantages, including the ability to detect and identify materials based on their unique spectral signatures, high spectral resolution, and the capability to provide detailed information about the composition and condition of objects. However, they also face certain limitations, such as the large volume of data generated, the need for extensive calibration, and the high cost of the technology.2
Conclusion
Hyperspectral cameras are powerful tools for acquiring and analyzing spectral data across a broad range of wavelengths. Their capacity to furnish comprehensive insights into the composition and state of materials and objects renders them indispensable in domains such as agriculture, environmental surveillance, medical diagnostics, and industrial quality assurance.
With ongoing technological advancements, hyperspectral cameras are well-positioned to assume a more pivotal part in propelling scientific investigation and enhancing practical applications.
References and Further Reading
- Calin, MA., Calin, AC., Nicolae, D. N. (2020). Application of airborne and spaceborne hyperspectral imaging techniques for atmospheric research: past, present, and future. Applied Spectroscopy Reviews. DOI: 10.1080/05704928.2020.1774381
- Boldrini, B., Kessler, W., Rebner, K., Kessler, R. (2012). Hyperspectral imaging: a review of best practice, performance and pitfalls for inline and online applications. Journal of Near Infrared Spectroscopy. DOI: 10.1255/jnirs.1003
- Sethy, PK., et al. (2022). Hyperspectral imagery applications for precision agriculture - a systemic survey. Multimed Tools Appl. DOI: 10.1007/s11042-021-11729-8
- Stuart, MB., McGonigle, AJS., Willmott, JR. (2019). Hyperspectral Imaging in Environmental Monitoring: A Review of Recent Developments and Technological Advances in Compact Field Deployable Systems. Sensors. DOI: 10.3390/s19143071
- Zhang, Y., Wu, X., He, L., Meng, C., Du, S., Bao, J., & Zheng, Y. (2020). Applications of hyperspectral imaging in the detection and diagnosis of solid tumors. Translational Cancer Research, 9(2), 1265–1277. DOI: 10.21037/tcr.2019.12.53
- Liyanage, DC., Tamre, M., Hudjakov, R. (2022). Hyperspectral/Multispectral Imaging Methods for Quality Control. In M. Habib (Ed.), Handbook of Research on New Investigations in Artificial Life, AI, and Machine Learning. DOI: 10.4018/978-1-7998-8686-0.ch017
- Tran, MH., Fei, B. (2023). Compact and ultracompact spectral imagers: technology and applications in biomedical imaging. Journal of Biomedical Optics. DOI: 10.1117/1.jbo.28.4.040901
- Wieme, J., Mollazade, K., Malounas, I., Zude-Sasse, M., Zhao, M., Gowen, A., Argyropoulos, D., Fountas, S., Van Beek, J. (2022). Application of hyperspectral imaging systems and artificial intelligence for quality assessment of fruit, vegetables and mushrooms: A review. Biosystems Engineering. DOI: 10.1016/j.biosystemseng.2022.07.013
Disclaimer: The views expressed here are those of the author expressed in their private capacity and do not necessarily represent the views of AZoM.com Limited T/A AZoNetwork the owner and operator of this website. This disclaimer forms part of the Terms and conditions of use of this website.