In an article published in Remote Sensing, researchers presented a point cloud reconstruction approach for a mobile laser scanning technique based on trajectory filtering. The authors employed deep learning to identify railway feature recognition points, and Rauch-Tung-Striebel (RTS) filtering to enhance the trajectory outcomes and correct the odometer data.
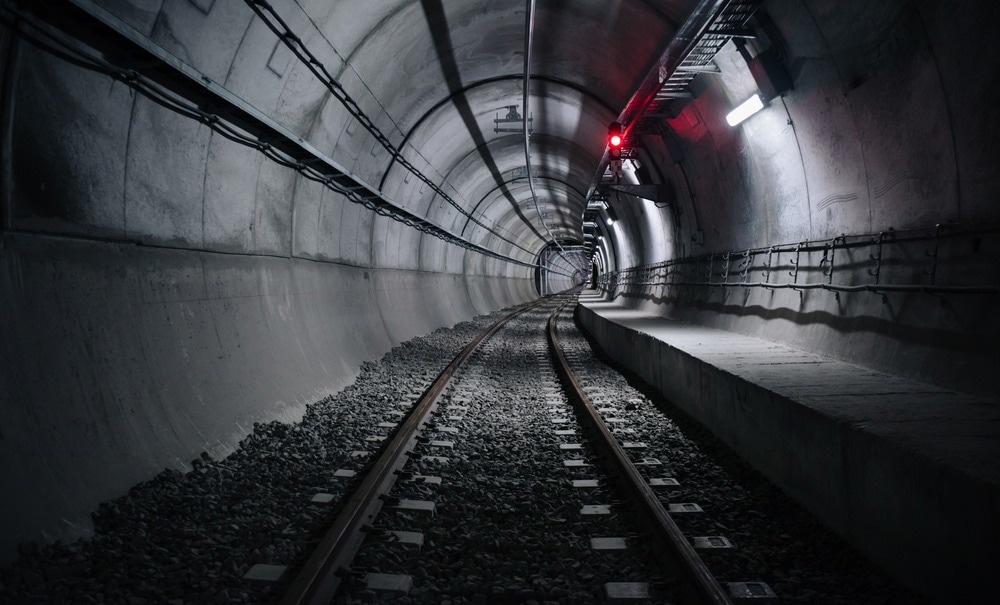
Study: A Railway Lidar Point Cloud Reconstruction Based on Target Detection and Trajectory Filtering. Image Credit: Nina Abrevaya/Shutterstock.com
A manual observation technique, such as a total station measuring approach, is used in a conventional railway survey. Despite the short data set and poor measurement efficiency, this approach has high precision. However, the demands of dynamic continuous high-precision holographic measurements cannot be met manually during railway outages.
A mobile mapping system that uses mobile laser scanning primarily consists of an inertial measurement unit (IMU), a laser scanner, and a panoramic camera. The benefits of mobile laser scanning include automation, high precision, and excellent efficiency. However, merging inertial measurement unit navigation data with mobile laser scanning data is challenging and needs to be resolved to collect accurate three-dimensional information about the railways.
Therefore, this study applied a mobile multi-sensor integrated detection technique to railway detection. The maximum discrepancy between the east and north coordinates was adjusted to within 7 cm when integrated with the railway experimental track data. In comparison, the average elevation error was observed to be 2.39 cm. The proposed multi-sensor mobile laser scanning system was crucial to the healthy growth of the railway system.
The Need for a Comprehensive Railway Inspection Approach
The speed and scope of railway tunnel constructions have made outstanding progress in recent years due to China's goal to improve its transportation system. A total of 16,798 railway tunnels totaling roughly 19,630 km had been constructed and were in use by 2020.
In contrast, more than 6000 km had been covered by built-in subway tunnels. The safety of these tunnels is a top priority for railroad operating companies. These enterprises have devoted time and money to conduct investigations on operation and maintenance inspection, deformation monitoring, etc.
The conventional inspection and engineering monitoring techniques utilize manual analysis, a total station, and a level to assess structural deformation. However, the operating efficiency makes it challenging to fulfill future super-large-scale network and routine maintenance detection requirements. Therefore, to inspect the operational tunnel while the skylight is open, the operation and maintenance department urgently requires a comprehensive and precise inspection system to replace the current inspection approach.
With the development of technology, comprehensive detection technologies have been created for the rail transit sector. Non-contact detection techniques based on mobile laser scanning have seen significant developments recently. Mobile laser scanning technology benefits from equipment downsizing, measurement, and imaging, with no requirement for lighting and cheap operating costs.
The railway mobile laser scanning measurement device was five to 10 times more effective than conventional measurement techniques and provided faster measurement speeds and richer data. This mobile laser scanning system was expensive in terms of hardware and software.
In this work, data was gathered using a mobile laser scanning device. The Kalman filter (KF) optimized the trajectory data to produce high-precision data. Mileage correction and automatic feature recognition were made possible by using orthophoto images.
Constructing the Novel Railway Mobile Laser Scanning System
The mobile laser scanning device and the position and attitude system were integrated into the system platform to fulfill the objective of the three-dimensional absolute coordinate measurements. The instrument platform was attached to the mobile laser scanning carrier to enable it to move with the carrier while performing quick dynamic scanning.
A multi-sensor integration box was created considering the platform's simplicity of construction and disassembly, the obstruction of the camera's field of view, the stability of multi-sensor installation, and the obstruction of the global navigation satellite system (GNSS) antenna.
A German Z+F 9012 section scanner was chosen as the mobile laser scanning device since it utilized the laser ranging principle, emitted laser pulses to the targeted surface, and recorded data on distance, reflection intensity, and angle. The device scanned at over one million points per second, with a maximum scanning speed of 200 rpm and a distance resolution of 0.1 mm, quickly and densely acquiring a significant amount of point cloud data about objects.
Three fiber optic gyroscopes and three accelerometers made up the inertial measurement unit. The inertial measurement unit oversaw determining the carrier's acceleration and angular velocity and communicating this data to the information processing circuit. The information processing circuit used the acceleration and angular velocity calculated by the inertial measurement unit for settlement and navigation.
Enhancing the recorded point cloud intensity values was required to show the differences between the point cloud intensity values of the feature recognition objects from railway scanning. Full-scale grayscale histogram stretching was applied. The findings revealed a few spots with low or high intensities in the initial scanned point cloud.
In this work, authors extracted fasteners from the orthophoto as a typical feature recognition point using the deep learning YOLOv3 target detection technique. The YOLO target recognition algorithm had some flaws because of the complicated environment of mobile laser scanning. Only deep learning could fully extract the coordinate data of feature recognition points. As a result, the target set of fasteners found by deep learning was initially segmented using the distance clustering method.
Significance of the Study
This study applied deep learning to identify objects in the generated orthophoto. Additionally, a mileage correction approach based on visual feature recognition was employed. The precise motion trajectory of the mobile laser scanning carrier was then recovered after optimizing the dead reckoning trajectory with RTS filtering. Also, the railway point cloud data in the coordinate system was obtained using the multi-sensor data fusion method.
The trajectory produced by the RTS filtering method and the scanning data were combined to create the three-dimensional point cloud data. According to the experimental findings, the mileage correction technique had good positioning precision and stability. The positioning error for the mileage was observed to be approximately 6 cm. Such precise mileage resulted in accurate trajectory computation. Additionally, the maximum errors of the 23 measured coordinate positions were all within 7 cm. Both the point's position and elevation had an average inaccuracy of 1.60 cm and 2.39 cm, respectively.
In contrast to the conventional method, which relied on manual railway detection, this research suggested a new approach employing a mix of inertial navigation data and calibrated mileage. The mobile laser scanning system's repeated calibration stops were avoided by segmentally calibrating the mileage using visual feature recognition points. It reduced the impact of the traditional method's trajectory error, which increased with time.
A real three-dimensional scene point cloud in an absolute coordinate system was obtained using the measuring technique in combination with inertial navigation and mobile laser scanning. The measurement point's speed and information output considerably outpaced conventional techniques. Extraction of railway components, such as the contact wire, track centerline, and catenary wire, could be finished in the future as per the point cloud data.
Additionally, point cloud data could be used to create three-dimensional models of railway structures. High-precision point cloud data could also assist in quickly completing the infrastructure information along the route. It was essential for digital transformation, intelligent operation and maintenance, and railway digital survey and design.
Reference
Liu, H., Yao, L., Xu, Z., Fan, X., Jiao, X., Sun, P. (2022) A Railway Lidar Point Cloud Reconstruction Based on Target Detection and Trajectory Filtering. Remote Sensing, 14(19), 4965. https://www.mdpi.com/2072-4292/14/19/4965
Disclaimer: The views expressed here are those of the author expressed in their private capacity and do not necessarily represent the views of AZoM.com Limited T/A AZoNetwork the owner and operator of this website. This disclaimer forms part of the Terms and conditions of use of this website.