Numerous eco-physiological processes and features correlate with vertical leaf angles and their temporal variation. Monitoring leaf angles of plant canopies in the field is challenging.
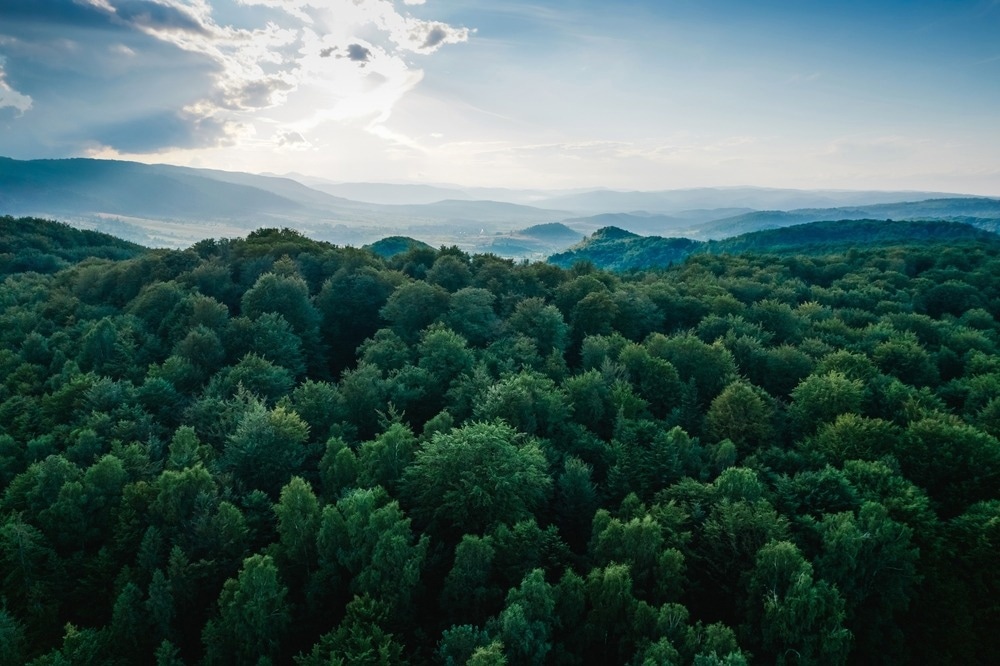
Study: AngleCam: Predicting the temporal variation of leaf angle distributions from image series with deep learning. Image Credit: Olga Danylenko/Shutterstock.com
A recent study published in Methods in Ecology and Evolution introduces an inexpensive time-lapse camera (Angle-Cam) based on deep learning for forecasting leaf angle distributions from horizontal images. Angle-Cam uses convolutional neural networks for pattern recognition. The researchers trained it using leaf angle distributions derived from visual analysis of more than 2500 plant images from various species and settings.
Effect of Environmental Stimuli on Leaf Angle Orientation
Plants display a variety of leaf angle configurations across species and growth patterns. Darwin discovered that the leaf angle arrangement of plants fluctuates with changes in phenology and diurnal cycles. Leaf angle arrangement responds to environmental signals such as light, competition, temperature, or water availability.
This includes nastic movements or non-directional movements responding to stimuli such as irradiance and temperature. It also comprises directed movements in response to a stimulus like the adhesion of precipitation. These environmental influences affect leaf angle orientations in different development forms, species, and even genotypes.
Influence of Leaf Angles on Plant Canopy Measurement
The vertical surface angles of individual leaves in a canopy (0° horizontal and 90° vertical) characterize their orientation. Leaf angle distributions describe the statistical distribution of vertical leaf angles within a canopy. Different fields can benefit from significant detection of leaf angles. The leaf angles directly influence the light absorption and photon production of plants.
Leaf angles are an important characteristic examined in community ecology to understand plants' competitive capacities. Leaf angles also affect productivity. The energy balance of canopies can be significantly altered by excessive radiation, heat, and drought stress. The interaction of incoming radiation with leaf angles significantly impacts how plants scatter light across wavelengths, influencing reflectance and fluorescence signals in earth observation data.
Limitations of Current Leaf Angle Detection Methods
There is no effective or automated way to monitor the temporal fluctuation of leaf angles or their distribution in plant canopies under field settings. Tracking the movements of individual leaves with inertial measurement units (IMU) has proven successful, but this method cannot be scaled to observe the distribution of leaf angles across entire plant canopies.
Methods based on 3D point clouds created by photogrammetry or terrestrial laser scanning enable estimation of leaf angle distributions, but they require expensive equipment and complex data acquisition and processing techniques, making them scarcely scalable for monitoring changes over extended periods and at high temporal resolutions (e.g., hourly).
Potential of Stereo Imaging for Multitemporal Analysis of Leaf Angles
The multitemporal analysis benefits from stereo imaging methods using rigs of standard cameras and image matching or depth analysis. Such methods necessitate highly technical hardware calibration, configurations, and image acquisition in the nadir view. Stereo imaging is an easier and less expensive approach based on ordinary cameras, in which a human interpreter estimates the angles of leaves that are perpendicular to the camera orientation from the images.
Although this method was successfully used with various plant species and timing phases, it lacks automation and is inefficient. Deep learning and computer vision-based pattern recognition advancements raise the possibility that artificial intelligence will eventually take over these interpretation responsibilities.
Deep Learning-Based Cameras for Leaf Angle Detection
Convolutional neural networks (CNNs) learn the image features needed to predict the target attribute. CNNs are currently the most used deep learning technique for predictive image analysis. Research in recent years has effectively used CNN to predict vegetation features using traditional RGB cameras.
Depending on how sophisticated they are, these models can take a while to train, but they can quickly predict outcomes once trained. Such a deep learning-based approach is a successful way to track temporal variation in leaf angles in conjunction with the improving performance of outdoor-capable cameras.
Angle-Cam an Effective Technique for Accurate Estimation of Leaf Angle Distributions
Kattenborn et al. introduced and assessed an approach (Angle-Cam) for estimating leaf angle distributions using plant images and deep learning-based pattern recognition methods. The researchers used independent samples and leaf angle estimates using TLS to evaluate Angle-Cam for various and complicated leaf shapes of various plant species. They also evaluated Angle-Cam for long-term monitoring of leaf angle distribution under field settings using high temporal resolution.
Research Findings
The productivity of plants and ecosystems, and Earth observation depend on vertical leaf angles and their variation over time, which are closely related to several eco-physiological processes and features.
Angle-Cam estimates leaf angles from horizontal plant images using CNNs and low-cost outdoor cameras, even if efficient ways to measure leaf angles are lacking. Angle-Cam successfully evaluated species and growth forms with reference data from independent samples and leaf angle estimations produced from TLS performance.
The Angle-Cam approach was tested in conjunction with consumer-grade outside cameras (3-min intervals, 4-month observation period). Environmental factors accurately account for the projected temporal change in leaf angles, supporting the method's validity and applicability.
Angle-Cam's output and potential derivatives are immediately compatible with various modeling applications, including radiative transfer, functional-structural plant, and Earth system modeling.
Reference
Kattenborn, T., Richter, R., Guimarães-Steinicke, C., Feilhauer, H., & Wirth, C. (n.d.). AngleCam: Predicting the temporal variation of leaf angle distributions from image series with deep learning. Methods in Ecology and Evolution, n/a(n/a). https://besjournals.onlinelibrary.wiley.com/doi/10.1111/2041-210X.13968
Disclaimer: The views expressed here are those of the author expressed in their private capacity and do not necessarily represent the views of AZoM.com Limited T/A AZoNetwork the owner and operator of this website. This disclaimer forms part of the Terms and conditions of use of this website.