A paper recently published in Biosensors demonstrated the feasibility of using a new method based on hyperspectral microscopic imaging technology and machine learning for skin cancer diagnosis.
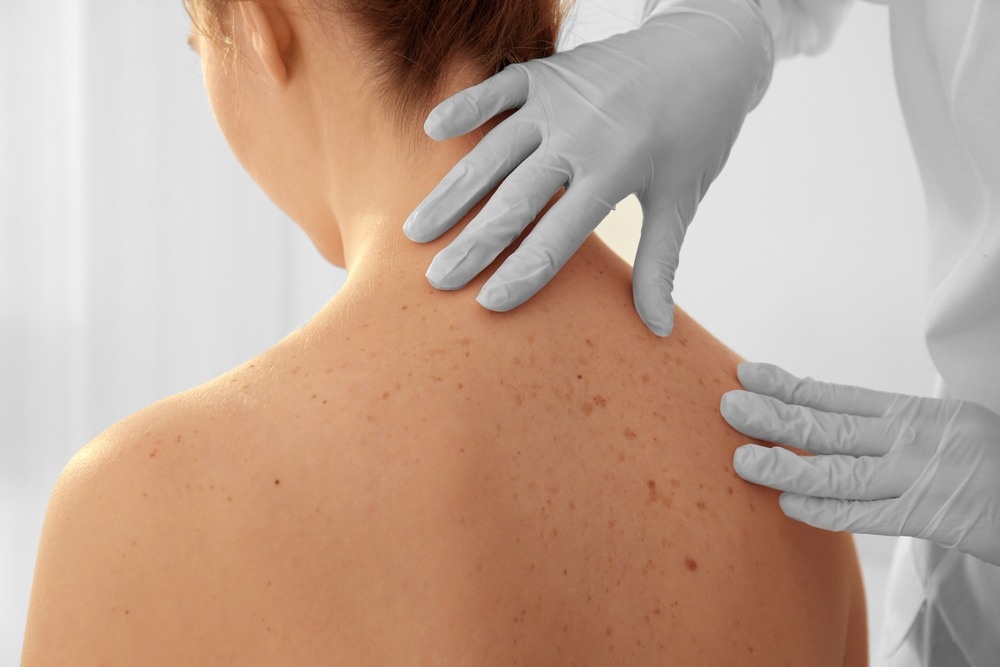
Study: Staging of Skin Cancer Based on Hyperspectral Microscopic Imaging and Machine Learning. Image Credit: Africa Studio/Shutterstock.com
The Growing Need for Early and Effective Skin Cancer Diagnosis
Skin cancer has increased considerably worldwide, specifically among old and middle-aged individuals. This has increased the significance of early disease detection to reduce mortality and morbidity.
Skin cancer is classified into malignant melanoma, squamous cell carcinoma, and basal cell carcinoma. Although a skin cancer diagnosis is traditionally performed using excisional biopsy, punch biopsy, and shave biopsy, the risk of misdiagnosis/missed diagnosis is higher using these methods.
New Method Based on Hyperspectral Microscopic Imaging Technology and Machine Learning as a Potential Solution
Hyperspectral microscopic imaging technology, a non-contact optical diagnostic method, can be used for the effective diagnosis of skin cancer.
This technology integrates hyperspectral imaging technology with microscopy to provide image and spectral information of the targeted tissue.
In the hyperspectral microscopic imaging cube, the spectral data reveal the changes in the internal microenvironment in samples through intensity and waveform, while the microscopic image data exposes the differences in the sample’s structure with high spatial resolution, improving skin cancer classification.
The combination of machine learning and hyperspectral microscopic imaging can considerably improve the accuracy and efficiency of a skin cancer diagnosis. For instance, a cancer detection system based on hyperspectral imaging and machine learning was successfully developed for cancer classification.
Investigating the Feasibility of Using Hyperspectral Microscopic Imaging Technology for Skin Cancer Diagnosis
Researchers used a hyperspectral microscopic imaging system to collect data cubes of squamous cell carcinoma, malignant melanoma, and basal cell carcinoma. They realized the squamous cell carcinoma staging identification based on the spectral data. The study also investigated the influence of the selected spectral region of interest on staging identification models.
A total of 39 malignant melanoma cases, 63 squamous cell carcinoma cases, and 34 basal cell carcinoma cases were used for the study.
A push-broom hyperspectral microscopic imaging system with 465.5–905.1 nanometer wavelength range, three-nanometer spectral resolution, and a total of 151 bands was utilized in the experiments.
The magnification, field of view, and actual resolution of the system were 28.15 ×, 400.18 micrometers × 192.47 micrometers, and 1.10–1.38 micrometers, respectively.
A 2048 × 985 × 151 hyperspectral microscopic imaging data cube containing spectral and image information was obtained using the hyperspectral microscopic imaging system.
In the data cube, specific points or regions of interest were selected for spectral analysis. The spectral information in the cube reveals the microenvironment variation and chemical composition within samples. The squamous cell carcinoma staging identification was performed using spectral data.
Spectral preprocessing methods, including the standard normal variate transformation (SNV), multiplicative scatter correction (MSC), and the first derivative (FD) and second derivative (SD) method, were used to reduce the influence of system scattering and external noise on the hyperspectral microscopic imaging system.
Dimensionality reduction methods, including partial least squares (PLS) and principal component analysis (PCA), reduced the hyperspectral microscopic imaging dataset dimension to enhance the model accuracy.
The skin cancer staging identification models were developed using four classification methods: random forest, decision tree, support vector machine, and extreme learning machine.
The model performance was evaluated based on kappa values and accuracy. The kappa value was used to assess the consistency of the judgment, with a 0.0-0.20 value indicating slight consistency and a 0.80-1.00 value indicating near-perfect consistency.
The original spectra of squamous cell carcinoma were preprocessed using SNV, MSC, and FD, respectively. Subsequently, PLS and PCA were performed on the original squamous cell carcinoma spectra at three stages for dimensionality reduction.
The PLS method more effectively extracted the spectral features and compressed the hyperspectral microscopic imaging data cube compared to the PCA method. The PLS method was utilized for spectral dimensionality reduction and the first ten principal components were used for the squamous cell carcinoma staging identification.
The dataset was then classified into a test and training set using the hold-out method. Eventually, the squamous cell carcinoma staging identification models of random forest, support vector machine, decision tree, and extreme learning machine were established.
MSC and SNV were used for spectra preprocessing, while extreme learning machine and random forest were used as the staging models to determine the effects of spectral regions of interest on squamous cell carcinoma staging identification.
Significance of the Study
The spectra preprocessing methods significantly influenced the kappa value and accuracy of the staging identification models, with the SNV performed most efficiently in every model.
Among all staging identification models, the highest staging accuracy of 0.952 ± 0.014 and kappa value of 0.928 ± 0.022 was observed in the random forest staging identification model, followed by the extreme learning model, support vector machine, and decision tree.
The spectral regions of interest influenced the staging results. For instance, the staging accuracies significantly improved, and the highest accuracy of 0.999 ± 0.001 and a kappa value of 0.998 ± 0.001 were recorded when the spectral regions of interest were nuclear compartments.
However, the kappa values and accuracies were lower when peripheral regions were selected as spectral regions of interest, indicating the more significant contribution of nuclear compartments to squamous cell carcinoma staging than peripheral regions.
The findings of this study demonstrated that squamous cell carcinoma staging identification can be performed with high accuracy based on machine learning and hyperspectral microscopic imaging technology, which can help effectively diagnose skin cancer.
This study also paves the way for research on spectral-spatial feature extraction to classify hyperspectral medical images.
Reference
Liu, X., Zhang, Z., Qu, J. et al. (2022) Staging of Skin Cancer Based on Hyperspectral Microscopic Imaging and Machine Learning. Biosensors. https://www.mdpi.com/2079-6374/12/10/790
Disclaimer: The views expressed here are those of the author expressed in their private capacity and do not necessarily represent the views of AZoM.com Limited T/A AZoNetwork the owner and operator of this website. This disclaimer forms part of the Terms and conditions of use of this website.