A study published in Forests explored and compared multiple remote sensing methods and machine learning algorithms to estimate the forest growing stock in large-scale forests.
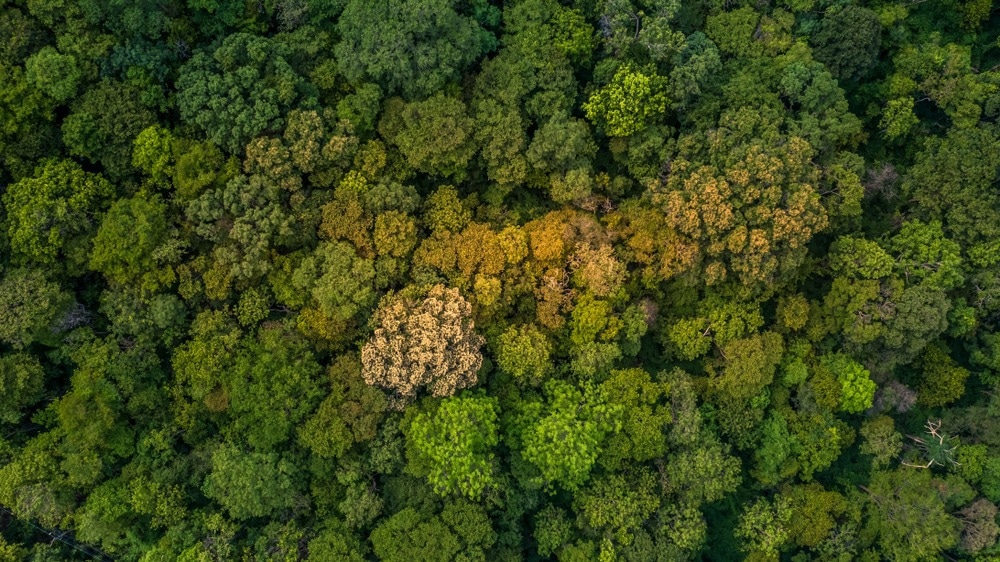
Study: Comparison of Multiple Machine Learning Models for Estimating the Forest Growing Stock in Large-Scale Forests Using Multi-Source Data. Image Credit: Avigator Fortuner/Shutterstock.com
Forest Growing Stock
Forest growing stock is an essential indicator for monitoring forest resources, and its quantitative estimation is critical.
Methods to Estimate Forest Growing Stock
Traditional survey methods
Traditional surveying techniques provide accurate and reliable forest resource monitoring and management data, but they are labor-intensive and time-consuming.
In addition, the lengthy time frame renders these conventional approaches ineffective for providing accumulated data in a dynamic trend.
Satellite remote sensing
Satellite remote sensing images are a valuable data source for collecting forest stand attributes at various temporal and spatial scales. Satellite remote sensing coupled with other auxiliary data is frequently utilized for quantitative forest stock estimation.
Optical remote sensing
Due to their extensive spectrum of information, optical remote sensing images are a common data source for estimating forest growing stock. However, clouds can interfere with optical remote sensing data.
The forest density severely obstructs the optical remote sensing echo signal, making it impossible to determine the forest's three-dimensional parameters, such as tree height.
Radar remote sensing
Radar remote sensing can penetrate the forest canopy to collect the spatial structural properties of the targeted forest and is not affected by atmospheric conditions. However, the problem arises when the backscattering signal becomes saturated and its intensity stops increasing.
Combined remote sensing data
Radar and optical remote sensing have their benefits and drawbacks. But, by combining the strengths of multiple sources, the shortcomings of a single remote sensing method can be compensated, and the accuracy of the estimated forest growing stock can be increased.
In addition to remote sensing data, soil survey data and data from digital elevation models (DEMs) are also being utilized to help forecast forest-related variables.
Significance of Machines Learning Algorithms in Estimating Forest Growing Stock
Remote sensing and forest growing stock data are too complex to be processed by single or multiple linear regression algorithms.
Machine learning algorithms address the shortcomings of non-linearity and spatial autocorrelation by determining the model structure in a data-driven way. Due to their versatility, these algorithms are frequently used to create complicated nonlinear tree models for estimating forest growing stock.
Gradient-boosting algorithms
Gradient boosting is a common machine learning algorithm that iteratively produces strong prediction models from weak prediction models. CatBoost, XGBoost, and GBDT are a few examples of gradient-boosting algorithms.
They are widely employed in prediction with superior performance to conventional machine learning algorithms.
Using Multi-Source Data and Machine Learning Algorithms to Estimate Forest Growing Stock
In this study, researchers used a combination of multiple data, including digital elevation model (DEM) data, Sentinel-2 optical remote sensing data, Sentinel-1 radar remote sensing data, the Lasso operator, inventory data for forest design and management planning, and three machine learning algorithms, CatBoost, XGBoost, and GBDT, to estimate forest growing stock.
Finally, the best estimate algorithm and multi-source data were combined by comparing a range of performance metrics.
Principle Findings of the Study
The Lasso algorithm efficiently decreased the number of independent variable components while retaining the primary characteristics, accelerating the model's training process and enhancing its generalization capability.
Radar remote sensing waves can effectively penetrate the forest surface to estimate the vertical characteristics of the forest. It partially compensates for the limitations of optical remote sensing data sources and can increase the accuracy of forest growing stock estimation.
The performance of the estimation models was greatly enhanced by adding category features, which allowed for more precise estimation. However, this is primarily reliant on the contributions of two factors.
One is the incorporation of the forest population and dominating species category features. The other is the addition of aspect direction and humus thickness category features, as they reflect the relationship between environmental factors and plant growth. The estimation model's accuracy increases after adding category features.
The CatBoost is the most effective algorithm for estimating the forest growing stock among the three gradient boosting models. Combining efficient machine learning algorithms and high-precision remote sensing data will pave the way for a more precise assessment of forest growing stock.
Reference
Huang, H., Wu, D., Fang, L., & Zheng, X. (2022). Comparison of Multiple Machine Learning Models for Estimating the Forest Growing Stock in Large-Scale Forests Using Multi-Source Data. Forests. https://www.mdpi.com/1999-4907/13/9/1471/htm
Disclaimer: The views expressed here are those of the author expressed in their private capacity and do not necessarily represent the views of AZoM.com Limited T/A AZoNetwork the owner and operator of this website. This disclaimer forms part of the Terms and conditions of use of this website.