Live-cell imaging at the nanoscale is challenging. Super-resolution microscopy techniques enable the observation of sub-cellular configurations below the optical resolution limit, but the spatial resolution is still insufficient for reconstructing the structures of biomolecules.
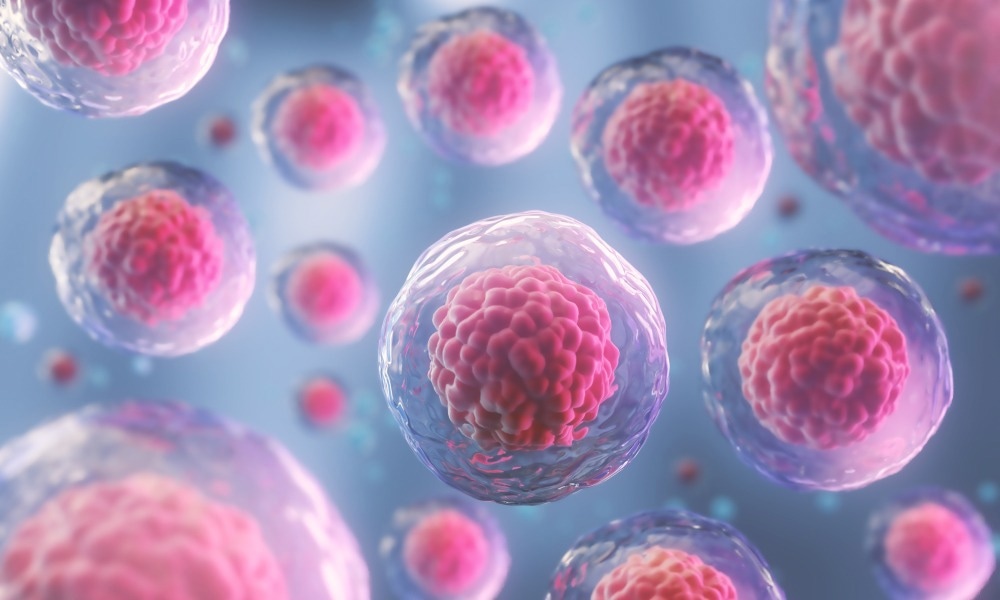
Study: Super-Resolution Reconstruction of Cytoskeleton Image Based on A-Net Deep Learning Network. Image Credit: Anusorn Nakdee/Shutterstock.com
A recent study published in Micromachines suggests that the A-net deep learning network can increase the resolution of cytoskeleton images taken by an optical microscope. The noise and flocculent structures that initially interfered with the cellular configuration in the raw image are removed using the DWDC algorithm to create new datasets and take advantage of A-net neural network's features. The spatial resolution is increased by a factor of 10 as a result. The study demonstrates a general method for accurately extracting structural information about macromolecules, cells, and organs from low-resolution images.
Monitoring of Biomolecules at Nanoscale
Nanoscale biomolecular structures play crucial roles, with the cytoskeleton functioning as a mechanosensor.
Monitoring the dynamics of biomolecules at resolutions of tens of nanometers, such as the 50 nm persistence length of DNA and 24 nm thickness of microtubule fiber, is crucial in understanding the underlying mechanism of cellular behavior. Transmission electron microscopy (TEM), stimulated emission depletion (STED), scanning electron microscopy (SEM), and cryogenic electron microscopy (Cryo-EM) are capable of achieving such resolution.
Limitations of Current Image Processing Methods
Live-cell imaging and in vivo molecular dynamics monitoring are not suited for TEM, SEM, and Cyto-EM. STED microscopy is an effective method. However, the presence of particular fluorophores, overly complicated operational procedures, and expensive costs restrict its applicability.
Image processing methods involve deep-learning and classical image processing algorithms. The super-resolution convolutional neural network is an end-to-end image processing network based on sparse coding to produce images with sharper edges and higher resolution. The tradeoff of processing speed to achieve an acceptable restoration quality is the drawback of a super-resolution convolutional neural network.
An update to the super-resolution convolutional neural network is the rapid super-resolution convolutional neural network. It significantly increases speed however, because of the severe smoothing, details are lost.
Development of A-net Deep Learning Network
Chen et al. developed the A-net deep learning network proposed by enhancing the structure of the U-net network. The finer details of a microtubule network acquired by a confocal microscope can be recovered with improved resolution when used in conjunction with a conventional degradation model to analyze label images.
Threshold denoising and three-dimensional Gaussian interpolation were first applied to raw images. Then, the matching label images were captured using the DWDC approach to extract finer structures, which combines Lucy-Richardson deconvolution and discrete wavelet. The datasets on which the A-net network was trained were pairs of original images and the accompanying label images. The test images were finally processed using the A-net network.
Improvement in Image Resolution
An innovative technique that combines existing image preprocessing procedures based on the degenerate model and A-net network is provided in this research to improve the resolution of a poor-quality image inherently, i.e., recover the real structure from a blurred and noisy image.
The image pairs of the original and label images are needed to create the training datasets for the A-net deep learning network. Due to the rarity and specificity of biological images, the researchers had to develop the dataset of biological microtubule images using the DWDC method, which consists of several preprocessing techniques, Lucy-Richardson deconvolution techniques, discrete wavelet techniques, and post-processing techniques. The training dataset is fed into the network to train the A-net network to map low-resolution images to high-resolution label images. The super-resolution images are then obtained after the test dataset is fed into the A-net network for prediction.
Research Findings
The molecular structure of the fibroblast microtubule networks from low-quality confocal images is extracted using a novel technique in this study that advances the A-net neural network and DWDC method. The process eliminates the challenge of acquiring biological images in biomedical and medical imaging disciplines and only needs a little amount of data.
The experimental results show a 10-fold increase in spatial resolution, with raw confocal images revealing a super-resolution of 120 nm. With fewer computational resources, the approach offers a general method for increasing the resolution of filament-like structures. Research in broad biological and biomedical fields, which heavily rely on optical imaging technology, will profit from the technique.
Reference
Chen, Q., Bai, H., Che, B., Zhao, T., Zhang, C., Wang, K., Bai, J., & Zhao, W. (2022). Super-Resolution Reconstruction of Cytoskeleton Image Based on A-Net Deep Learning Network. Micromachines, 13(9), 1515. https://www.mdpi.com/2072-666X/13/9/1515/htm
Disclaimer: The views expressed here are those of the author expressed in their private capacity and do not necessarily represent the views of AZoM.com Limited T/A AZoNetwork the owner and operator of this website. This disclaimer forms part of the Terms and conditions of use of this website.