A study published in ACS Photonics discusses how deep learning and conventional optimization-based methods create a new conceptual foundation for freeform optical engineering. These algorithm-driven methodologies will help scientists and engineers streamline the optical systems near the physical limitations of structured media.
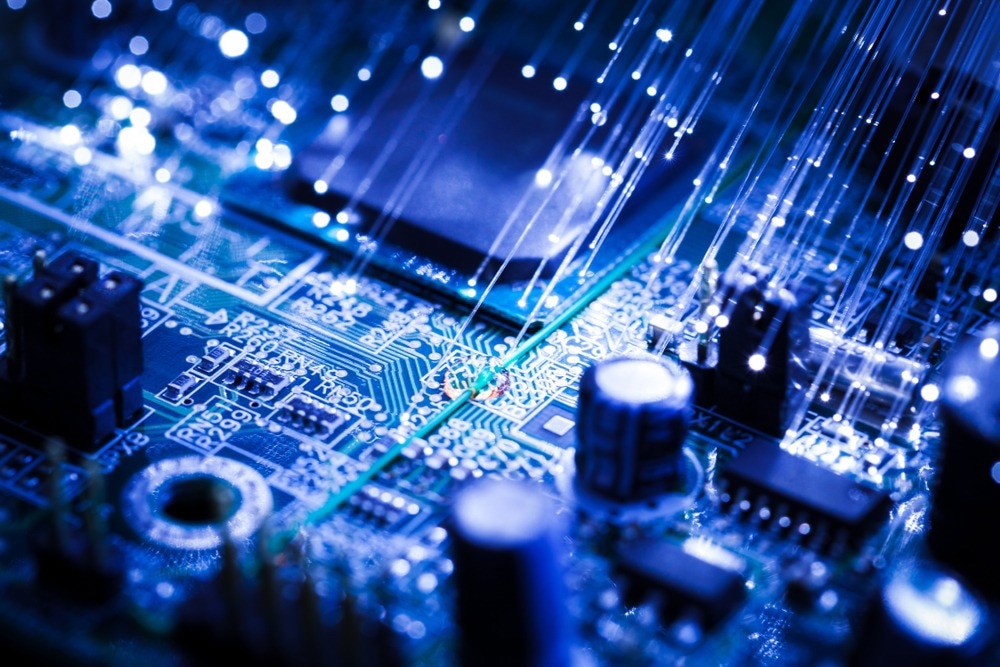
Study: Algorithm-Driven Paradigms for Freeform Optical Engineering. Image Credit: AerialVision_it/Shutterstock.com
Significance of Freeform Design in Optical Systems
Understanding and utilizing the relationship between electromagnetic response and geometric shape optimization enables the experimental implementation of optical systems within the discipline of optical engineering.
This relation has been based primarily on physical laws. However, a combination of simplifications, approximations, and limitations to physical systems led to intuitive models and design techniques.
Recent advances in high-precision manufacturing have opened up new possibilities for experimental patterning of geometric features, which in turn has the potential to reshape the optical engineering process.
Modern machining techniques enable the fabrication of refractive optical components with arbitrary profiles. Additive manufacturing generates nearly arbitrary 3D structures with feature sizes ranging from the macro- to the submicrometer scale. In addition, lithography enables the routine patterning of thin films into nanoscale freeform shapes.
Each voxel in the pattern process is a free design parameter, making the geometric feature space for optical design presented by these fabrication processes significant. This is transforming the design and fabrication workflow for photonic technologies.
How Will Deep Learning Algorithms Streamline Optical Systems Design?
Algorithmic optical engineering tools redefine the conceptual underpinnings of how optical system design is approached.
By approaching the relationship between the optical response and the device as a physics-constrained data relationship, algorithmic-based methods can uncover novel, non-intuitive structure-function solutions, as opposed to standard conceptions based on intuitive physical models established by people.
Data science algorithms are playing an essential part in this progress. However, they have shown to be especially destructive in photonics, owing to the field's well-developed quantitative physical underpinnings.
Full-wave Maxwell simulators effectively act as ground truth oracles, and they can be utilized to compute structure-function solutions to generate training data quickly or meticulously calculate gradients quickly.
Algorithms in data science explicitly use physics and are particularly effective at learning and leveraging non-intuitive structure-function connections.
Recent years have seen a confluence of factors that have enabled the emergence of a new breed of optical engineering. These factors include the rapid development of machine learning, optimization theory improvements, new computing hardware production, and the ongoing improvement of experimental fabrication tools.
Challenges
For these tools to be widely recognized and utilized by the photonics community, numerous developments will be required.
The processes must be scalable to broad domains of any size without sacrificing accuracy. This is an issue for many algorithms, particularly data science algorithms with fixed and generally narrow domain dimensions.
The interface between electromagnetics and data sciences and the interaction between neural network architecture and scientific computer operations must be enhanced further.
The designed algorithms must be open to the public, robust, and simple to use, and many of these techniques require the user to have extremely specialized knowledge in data sciences, freeform optimization, or physics.
The widespread use of machine learning principles and their adaption to user-specific situations will necessitate the development of algorithms that use automated and efficient hyper-parameter tweaking, neural network design specification, and active-learning techniques.
The study of electromagnetic performance bounds computations is still in its infancy, and nontrivial bounds have only recently been established for a small number of photonic devices.
While many of these ideas provide limited device design and execution solutions, comprehensible algorithmic approaches will allow more fundamental advancements in optical engineering that connect non-intuitive structure-function solutions to wider physical insights.
Future Outlook
Algorithms will enable new paradigms in optical engineering. One example is the creation of real multiscalar optical design and production platforms that include scalar diffractive, refractive, and full-wave optics.
Optical systems at each length scale possess unique characteristics. However, these modalities can be synergistic, resulting in systems with excellent bandwidth, functional multiplexing, and aberration correction qualities. They also open new possibilities for connecting chip-based and free space optical systems.
There are enormous prospects to connect optical engineering to non-electromagnetic physical and software domains, resulting in optical systems with entirely new functionalities.
Reference
Mingkun Chen, Jiaqi Jiang, and Jonathan A. Fan. (2022). Algorithm-Driven Paradigms for Freeform Optical Engineering. ACS Photonics. https://pubs.acs.org/doi/10.1021/acsphotonics.2c00612
Disclaimer: The views expressed here are those of the author expressed in their private capacity and do not necessarily represent the views of AZoM.com Limited T/A AZoNetwork the owner and operator of this website. This disclaimer forms part of the Terms and conditions of use of this website.