Optical microscopy has been adopted as one of the workhorse techniques in a number of different areas. From histology and medical diagnosis to materials analysis, the magnifying power of optical microscopy has facilitated great insights into microscopic structures that cannot be seen by the naked eye.
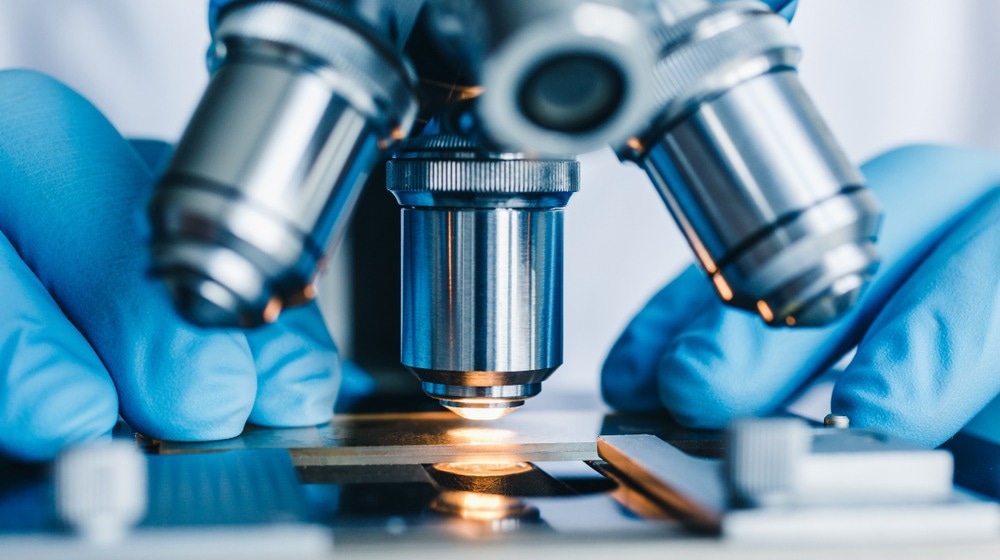
Image Credit: Konstantin Kolosov/Shutterstock.com
The development of histology – the study of tissues and their structure – began with the development of optical microscopy which made it possible to see these complex structures for the first time.1 Now, histology, or histopathology – the study of tissues affected by disease – is one of the most useful diagnostic tools in the medical laboratory.2
Optical microscopy is a highly versatile imaging method for disease diagnosis. It is compatible with a number of tissue types and the sample preparation is fairly universal without the need for the often highly specific fluorophores required for fluorescence microscopy.
Sample preparation for optical microscopy is relatively straightforward. It requires slicing and staining the sample and the use of specific stains to highlight certain features in the tissue. This can help identify different regions of the tissue and simplify diagnosis.
Image Analysis
Time is of the essence when making clinical decisions and that means turnaround times for analysis need to be as short as possible. Developing high throughput methodologies is therefore very attractive for busy hospital laboratories that may need to process thousands of samples a day.
There has been great interest in developing high-throughput variations of optical microscopy methods, both with very standard optical microscopies as well as variations of the technique such as non-linear optical microscopy.3
Some design aspects to improve sample throughput includes sample loaders and advanced autofocusing systems to reduce downtime between changing samples. Greater degrees of automation on optical microscopy systems can also help achieve greater sample throughput.
However, another significant bottleneck in optical microscopy is the time it takes for a human to process the images. In histopathology, deformations or abnormalities of the tissue structure are used to diagnose the disease in question.
The accuracy of diagnosis is highly dependent on the analyst’s skills as well as the quality of the sample preparation and preservation. Requiring manual inspection of the images means a fully automated workflow is not possible and introduces a bottleneck into the sample processing.
To move towards fully automated and on-demand analysis to improve the throughput in hospital laboratories, there has been great interest in the adopted machine-aided or fully computerized diagnosis of images.4 Image recognition algorithms can recognize the characteristic tissue deformations associated with particular diseases. The changes in tissue are often associated with disease and are so definitive for diagnosis that histopathology is considered the ‘gold standard’ for cancer diagnosis.
Deep Learning
While there is great motivation in terms of improving efficiency, and potentially accuracy, in terms of medical diagnostics through the use of automated image processing, there are a number of challenges to be addressed. One of these is the volume of data generated.5 This means the data requires a large amount of storage and often data reduction steps need to be taken to make the dataset a manageable size for analysis and processing.
Automated image analysis has been successfully applied to images acquired from a number of optical microscopy techniques, including quantitative phase microscopy.6 The deep learning model uses training datasets of images to learn the characteristic histological markers of a particular disease. Once the model has been trained, it can then classify images based on criteria such as the presence or absence of those features.
The quality and size of training datasets is a key issue for deep learning algorithms. If the datasets are too small, overfitting of the model and poor generalizability can become a problem and compromise the accuracy; the training sets are expanded by reusing the same images but after some processing, such as rotation.6
Deep learning approaches are particularly demanding in terms of the size of the training dataset compared to other machine learning approaches but methods such as convolutional neural networks (CNNs) tend to boast better significant feature extraction, improving their accuracy.
There are now models achieving over 90% sensitivity and 85% specificity, even with microscopy images taken on a smartphone, showing the promise of such approaches for the analysis of optical microscopy images.6
Deep learning for image processing would be a significant step in offering point-of-care diagnostics that could provide diagnostic information with rapid turnaround times and without the need for an experienced clinician. As well as accelerating diagnostics times, one of the key benefits of moving towards artificial intelligence in healthcare is that the clinician’s skill level no longer factors into the probability of a successful diagnosis. Where there are ambiguities in images that mean deep learning methods cannot make a clear diagnosis, then these images can then be flagged for manual processing.
References and Further Reading
- Hussein, I. H., & Raad, M. (2015). Once Upon a Microscopic Slide: The Story of Histology. Journal of Cytology & Histology, 06(06). https://doi.org/10.4172/2157-7099.1000377
- Musumeci, G. (2014). Past, present and future: overview on histology and histopathology. Journal of Histology and Histopathology, 1(1), 5. https://doi.org/10.7243/2055-091x-1-5
- So, P. T. C., Yew, E. Y. S., & Rowlands, C. (2013). High-Throughput Nonlinear Optical Microscopy. Biophysical Journal, 105(12), 2641–2654. https://doi.org/10.1016/j.bpj.2013.08.051
- Yanase, J., & Triantaphyllou, E. (2019). A systematic survey of computer-aided diagnosis in medicine: Past and present developments. Expert Systems with Applications, 138, 112821. https://doi.org/10.1016/j.eswa.2019.112821
- Xu, Y., Jia, Z., Wang, L., Ai, Y., Zhang, F., Lai, M., & Chang, E. I. (2017). Large scale tissue histopathology image classification, segmentation, and visualization via deep convolutional activation features. BMC Bioinformatics, 18, 281. https://doi.org/10.1186/s12859-017-1685-x
- Kaniyala, S., Dharshini, M., Shweta, G., Anirudh, C., & Kashyap, A. (2022). Deep learning-based image processing in optical microscopy. Biophysical Reviews, 463–481. https://doi.org/10.1007/s12551-022-00949-3
Disclaimer: The views expressed here are those of the author expressed in their private capacity and do not necessarily represent the views of AZoM.com Limited T/A AZoNetwork the owner and operator of this website. This disclaimer forms part of the Terms and conditions of use of this website.