AZoOptics speaks to Dr. Keith Paulsen about the importance of breast cancer detection and the introduction of his team's deep-learning algorithm that associates spatial images of tissue optical properties with optical signal patterns measured during an imaging experiment or patient exam. If the combined MRI, plus optical instrumentation and associated imaging algorithm, are successful, and larger clinical studies validate its performance, contrast injection could be eliminated from use during breast imaging. The research was published in the Optica journal.
Can you tell us briefly about your role and how you began researching ways to improve breast cancer detection?
Working with a number of faculty colleagues at Dartmouth’s Thayer School of Engineering and Geisel School of Medicine, we have developed a very productive and well-funded research program in biomedical engineering and imaging.
I serve as Principal Investigator of a number of research projects supported by the US National Institutes of Health’s National Cancer Institute and National Institute of Biomedical Imaging and Bioengineering. Our teams have been working on ways to improve breast cancer detection and treatment for about 25 years.
We have focused on developing and translating new imaging/therapy technologies in the form of novel instruments and algorithms which we hope will change clinical practice if we can demonstrate our methods are effective.
Can you explain multi-modality imaging processes and their role in medical imaging?
Multi-modality imaging refers to the combination of more than one imaging method, often administered at the same time, to gain more information about the tissues or organs of interest. Typically, each method provides image data and associated medical information that is not available from its partner modality. A good example is PET-CT – the combination of PET and CT scanning. In this case, CT provides high-resolution data about tissue geometry and structure whereas PET returns information on tissue function.
The structure-function combination is a common goal in multi-modality imaging. In many ways, tissue structure is easier to image and a number of methods are available to produce this information at high spatial resolution.
Tissue function is harder to image and/or measure, and most functional imaging methods return data at lower spatial resolution. The combination, especially when obtained concurrently, is particularly powerful because the underlying structure when imposed at a high spatial resolution on top or in combination with lower resolution functional data makes the latter much easier to understand and interpret. In the case of PET-CT, the images from each are, in effect, independent of one other. In other situations, for example in the MRI combination with optical imaging, we are investigating the use of image data from one modality to improve the imaging results of the other modality.
What are the current limitations of breast cancer imaging?
Breast imaging over the last 20+ years has focused largely on early detection as the best way to improve patient survival from breast cancer. The effort has been mostly very successful in terms of screening for breast cancer with x-ray mammography. That said, breast mammography is a planar projection technique, which is two-dimensional and obscures the 3D structure of the underlying tissue, making interpretation difficult, especially in breasts that have larger amounts of fibrous and glandular structure in which cancers originate.
3D mammography, also known as breast tomosynthesis, does exist, is gaining traction, and mitigates the tissue overlap limitation of 2D projections, but it does not provide a true volumetric 3D view of the breast.
MRI offers exquisite 3D views of the breast’s underlying parenchymal structure and is recognized as the most sensitive breast imaging method for cancer when contrast is injected, but contrast enhancement also occurs in breast tissues that are not malignant. As a result, contrast-enhanced breast MRI generates a lot of potential positive cancer indications that need to be biopsied in order to obtain a definitive diagnosis that often turns out to be false. In our MRI plus optical imaging technique, we are working to address the false positive problem that MRI alone presents. We are also seeking to determine if we can eliminate the need for contrast injection with MRI altogether by introducing optical imaging as a surrogate for the imaging advantages afforded by contrast injection in the breast. Contrast injection for breast MRI is generally considered to be safe but does carry some risks that would be very desirable to eliminate if a multi-modality approach could be developed that would achieve the same level of sensitivity of contrast-enhanced breast MRI but without the contrast agent.
Why is it beneficial to combine optical and magnetic resonance imaging data, and how is this possible?
Combining optical imaging with MRI offers several potential benefits. First, it creates a classic structure-function imaging pair with MRI generating high-resolution structural information in the breast in 3D, and optical imaging producing lower resolution functional data on breast tissue blood hemoglobin content and oxygen saturation, and lipid (fat) and water content. Because optical imaging tracks blood content through hemoglobin as its primary imaging variable, it has the potential to reach tissue compartments associated with cancer that are elucidated by injecting a contrast agent with MRI, which track vascular leakage associated with cancers. Second, the high-resolution structural data from MRI can be incorporated into optical image formation; thus, the optical method can leverage MRI spatial data when forming the optical images, and by doing so recover the functional optical information at higher spatial resolution. The light illuminating and sensing instrumentation associated with optical imaging can be realized with components that are compatible with MRI. Breast MRI uses coils for signal detection that do not touch the surface of the breast. Thus, the optical light sources and detectors which are placed on the breast surface can be applied without interfering with the standard breast MRI exam.
Can you outline your study, including the methods taken and key findings?
In this study, we developed a deep-learning algorithm that associates spatial images of tissue optical properties with optical signal patterns measured during an imaging experiment or patient exam, rather than using a physics-based mathematical model to describe how the optical source light propagates through the breast to detector locations as the basis for estimating images of the intervening optical properties in the breast.
The deep-learning approach appears to learn features of the optical signal illumination and detection system that are not represented in the modeling approach but the effects of which are included in the measured optical data. Thus, by deep-learning how patterns in the measurement data are associated with spatial maps of tissue optical properties, the algorithm is able to recover these spatial maps from data acquired during patient breast exams.
Training the deep learning algorithm is a critical step. Here, we used 20,000 sets of computer-generated data from phantom experiments that mimic actual breast exams but under simplified conditions. Once the algorithm was trained, we gave it two sets of data from clinical patient exams – one from a subject who had a known breast malignancy and another from an individual who harbored a benign condition that looked like cancer from the MRI alone. The trained deep-learning algorithm when given these clinical exam data recognizes the image contrast expected from signal data collected from the malignant and benign cases.
We were surprised, and obviously very pleased, that the deep-learning algorithm could be trained with data from phantom experiments, much of which was simulated; yet, learn to recognize signal patterns and associated optical images from human breast exams.
How does the new algorithm help distinguish between malignant and benign tumors?
We use criteria we have established during prior clinical trials for breast MRI plus optical imaging, namely, hemoglobin concentration in suspicious lesions evident on MRI relative to surrounding breast parenchyma.
Benign breast conditions are found when this contrast in hemoglobin is approximately unity whereas a cancer indication occurs for hemoglobin contrast ratios that exceed one. Water images reinforce the hemoglobin signature when their contrast is high for malignancy and low for benign conditions. The new algorithm generates the same types of images we have produced in the past during prior clinical studies, but does so with a deep-learning neural network that was trained to look at patterns in the optical measurements that are indicative of a cancer classification relative to a benign diagnosis.
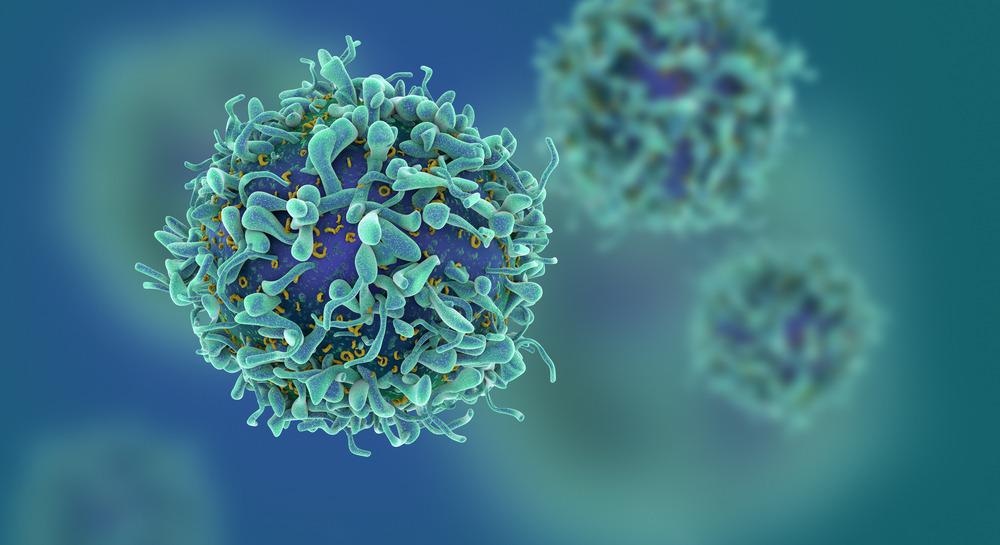
Image Credit: fusebulb/Shutterstock.com
What will this mean for the future of breast cancer diagnosis?
Our current results are too preliminary to offer any concrete answers at this time. However, if the combined MRI plus optical instrumentation and associated imaging algorithm prove successful, and larger clinical studies validate its performance, in the best case, contrast injection would be eliminated from use during breast imaging for cancer diagnosis and surveillance which would be a risk reduction for patients. Further, if the false positive rate of breast MRI alone can be reduced significantly with the MRI plus optical imaging approach, the number of false-positive biopsies could be reduced substantially and/or even eliminated.
How will this technology be adaptable for use with other cancers and diseases?
Results from the study suggest that machine learning methods are applicable to image formation problems where biophysical mathematical models are used as the framework for generating images of tissue property parameters from sensor data acquired by the imaging instrumentation. Thus, the approach may generalize beyond the specifics reported here (optical data/measurements to recover images of hemoglobin concentration, for example) to other signal sources and diseases in addition to the breast cancer optical detection problem we investigated.
How is your team able to confirm the quality and useability of the reconstructed images?
In this study, we have not yet confirmed the quality and usability of the images that were generated. That said, we have many years of experience with this technology, including in clinical trials in which the quality and usability of image data were evaluated. Image data we generated in the paper is of quality and usability similar to past results. Hence, while we have more work to do, we are confident the quality and usability of reconstructed images will meet or exceed clinical acceptance thresholds if the image data continues to perform similarly to the results generated so far.
What challenges has your team faced during your research and how have these been overcome?
Probably our biggest recent challenges have been COVID-related in terms of access to laboratory and clinical spaces for research and development, and clinical study execution. We have also faced supply-chain delays; much of our instrumentation involves customized design and fabrication, and access to these services and receipt of custom-designed products and components have been slowed and extended considerably in time. Since our instrumentation is a custom design, validating it and finding errors in its design and manufacture through performance testing that need to be fixed has taken more time.
What are the next steps for the project?
We hope to finalize our 3rd generation instrument and begin testing it, first in human volunteers and then in patients, during the next year. In terms of the algorithm described in this paper, we plan to apply it to a much larger database of clinical breast imaging cases where we know the gold standard diagnosis to determine if the very promising initial performance holds up in a larger series of clinical cases. We will then, of course, apply the algorithm prospectively to data collected in new clinical cases we hope to begin in the near future.
Where can readers find more information?
https://opg.optica.org/optica/fulltext.cfm?uri=optica-9-3-264&id=469839
https://link.springer.com/article/10.1245/s10434-019-07531-4
https://link.springer.com/article/10.1007/s10549-020-05780-6
https://www.pnas.org/doi/abs/10.1073/pnas.0509636103
https://aacrjournals.org/clincancerres/article/21/17/3906/117559/MR-Guided-Near-Infrared-Spectral-Tomography
About Dr. Keith Paulsen
Dr. Keith Paulsen holds the Robert A Pritzker Chair in Biomedical Engineering at Dartmouth’s Thayer School of Engineering, and also has appointments at the Professor rank in Radiology and Surgery at the Geisel School of Medicine.
He is a Fellow of the International Society for Optics and Photonics (SPIE), the Institute of Electrical and Electronic Engineers (IEEE), the American Institute for Medical and Biological Engineering (AIMBE), the Optical Society of America (OSA), and National Academy of Inventors (NAI).
According to Google Scholar, Paulsen is a contributing author on papers with an h- and i-indices of 103 and 481, respectively. He is an inventor on more than 35 patents, and a founding member of two start-ups; InSight Surgical Technologies and Cairn Surgical (https://www.cairnsurgical.com/breast-cancer-locator/ ), the latter having won the 2021 New Hampshire Product of the Year for its Breast Cancer Locator technology: https://www.dartmouth-hitchcock.org/stories/article/new-hampshire-cancer-researchers-breast-cancer-surgery-innovation-wins-coveted-award.
Disclaimer: The views expressed here are those of the interviewee and do not necessarily represent the views of AZoM.com Limited (T/A) AZoNetwork, the owner and operator of this website. This disclaimer forms part of the Terms and Conditions of use of this website.