Camera sensors are light-sensitive detectors that convert the incident photons into an electrical signal that can be read out by a digital device. Most cameras make use of 2D array detectors and choosing the right sensor type for a given application is often a trade-off between cost, desired final image resolution and the necessary read-out speed.
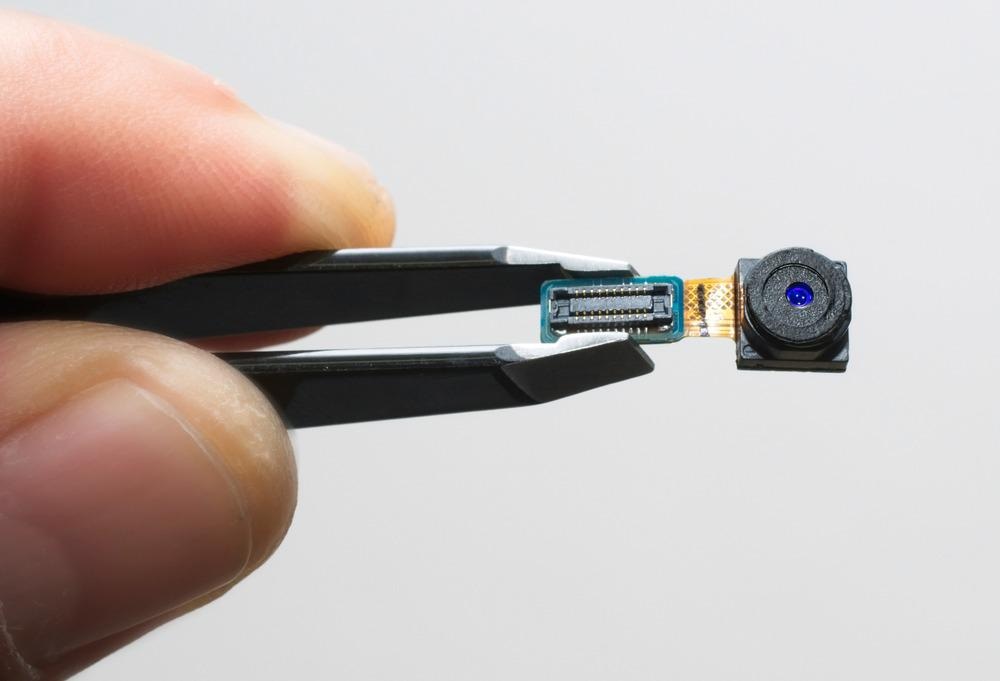
Image Credit: AlessandroZocc/Shutterstock.com
The most common types of camera sensors are charged-couple devices (CCD) or complementary metal-oxide-semiconductor (CMOS) image sensors. These are two different sensor technologies that have different strengths and weaknesses depending on the likely operating conditions of the final device.
Historically, CCD sensors have been the preferred choice of sensor for high-resolution and low-light applications.1 A CCD sensor is an array of pixels that are made from a material that produces electrons when struck with a photon.
A series of electrodes collect and read out the charge produced, typically through a single amplifier. Each pixel is read out by transferring the charge across the other pixels.
CCDs generally have a very low noise readout due to the design of the amplification stage. A CCD can be designed with an excellent dynamic range and good linearity over most of this detection range which can simplify many measurements. As each pixel needs to be read out individually, read-out times can be problematic for creating particularly large array detectors using this architecture.
In recent years, however, there have been many advances in CMOS technologies and, particularly for small footprint, lightweight ‘camera on a chip’ applications, CMOS sensors have now become the most widely adopted technology. Improvements in manufacturing have helped reduce noise levels and, while few CMOS sensors boast the same dynamic range as CCD sensors, the power efficiency and cost-effective nature of CMOS sensors often compensate.2
Machine Vision
Machine vision is a rapidly growing area that was worth 3.23 billion USD in 2021, with a projected growth of 7.7% year on year.3 Machine vision involves the capture of visual information, perhaps with a camera sensor, and the use of image recognition so the computer can ‘interpret’ this visual information as useful data.
The success and rapid development of machine vision are because of its utility in a whole range of applications, including industrial automation, the creation of autonomous vehicles and robots, and more generally ‘smart’ systems where devices can respond to external stimuli in their environment.
One of the main challenges in developing a machine vision system is the creation of a visual capture system that can provide sufficiently high-quality images that an automated image recognition algorithm can meaningfully interpret the data with high accuracy.
Many machine vision applications are also for autonomous vehicles or robots that are not necessarily connected to a wired network or power source, so any image processing needs to be sufficiently lightweight that it can be carried out in a sufficiently short time for the machine to have a meaningful response without huge energy or computational demands.
CMOS Camera Sensors
CMOS sensors have become one of the key technologies in facilitating the development of many machine vision applications.4 Many automation tasks require 3D object recognition, not just 2D and so it is often essential to use multiple camera sensors to provide different viewpoints for recognition of the full 3D seen. The cost-effective nature of CMOS technologies has made more complex machine vision applications now economically feasible.
Improvements in the quantum efficiency of CMOS sensors have also helped as images require shorter exposure times and more challenging low-light environmental conditions no longer pose an impossible challenge.
The quality of a camera sensor is key to the overall optical performance of the device, so while focusing optics and apertures can be used to help create sharper images, the quality of the chip will normally dictate the ultimate resolution.
As CMOS sensors are highly responsive and have very short readout times, they can be used to rapidly capture a series of images for dealing with dynamically evolving situations. While this is very challenging from a data handling perspective if many images need to be analyzed as part of a sequence, in terms of total information content, such image stacks provide a huge amount of potential material for analysis. Rapidly capturing images also helps circumvent issues such as motion blur.
Developments in Machine Vision Sensors
Machine vision is becoming a huge application area for CMOS sensors, though it is very important to choose the right type of sensor for the application.
One way to reduce the costs of the camera sensor is to use sensors that are only sensitive to a single color. However, for applications such as assessing fruit ripeness that often uses the color of the fruit as useful information in the image interpretation step, a single color detector may not be suitable.
Developing faster frame rates for image capture and sensors that can operate outside of the visible light region are two major areas for camera sensor development for machine vision.
Faster capture means fewer issues with motion blur. Many applications would also benefit from the types of spectral information that can be obtained from wavelengths in the infrared.
References and Further Reading
- Magnan, P. (2003). Detection of visible photons in CCD and CMOS: A comparative View. Nuclear Instruments and Methods in Physics Research Section A, 504, 199–212. https://doi.org/10.1016/S0168-9002(03)00792-7
- Carlos, L., & Gouveia, P. (2016). Advances on CMOS image sensors. Sensors, 3, 231–239. https://doi.org/10.1108/SR-11-2015-0189
- Grand View Research (2022) Machine Vision Market Size, https://www.grandviewresearch.com/industry-analysis/machine-vision-market, accessed April 2022
- Coffey, V. C. (2018). Machine Vision. Optics and Photonics News, 42–49. https://opg.optica.org/opn/abstract.cfm?URI=opn-29-7-42
Disclaimer: The views expressed here are those of the author expressed in their private capacity and do not necessarily represent the views of AZoM.com Limited T/A AZoNetwork the owner and operator of this website. This disclaimer forms part of the Terms and conditions of use of this website.