Cryo-electron microscopy (EM) has made it possible to obtain structural information on a wealth of biological samples that could previously not be imaged. This is because cryo-EM methods rely on flash freezing samples for imaging rather than being able to successfully grow a crystalline structure of sufficient high quality that is required for X-ray crystallography methods.
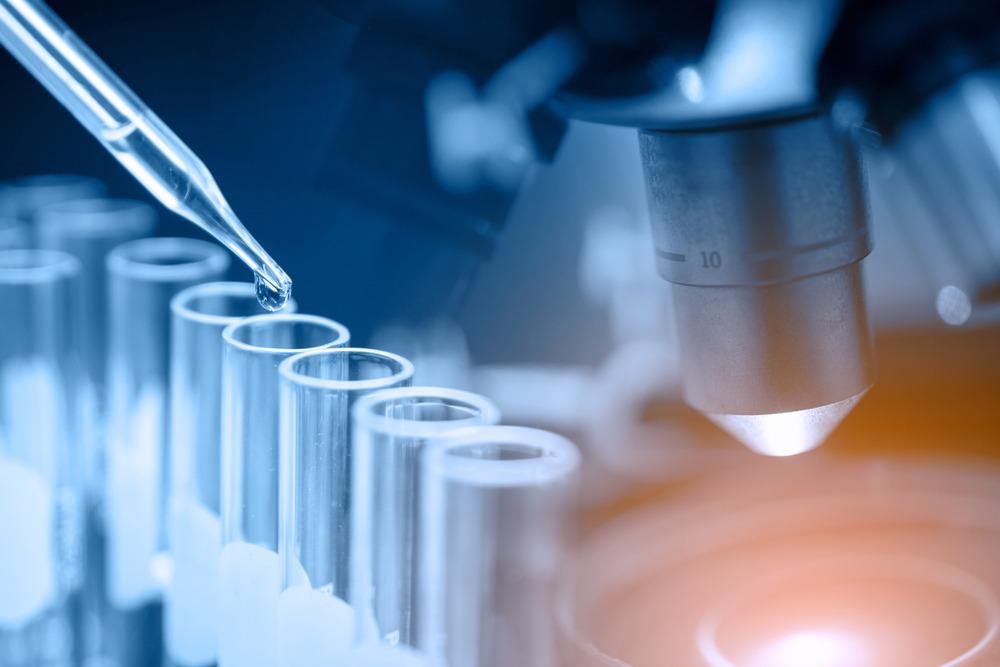
The number of biological structures identified with cryo-EM has been growing exponentially, with predictions that by 2024, the number of structures identified with EM methods will exceed that of crystallography.1 Proteins that are found in cell membranes are particularly amenable to structural imaging with cryo-EM.
Improvements in the achievable resolutions with a combination of electron beams and the corresponding imaging cameras have enhanced the level of structural detail that is possible and will play an important future in further cryo-EM developments. The ability to use lower power electron beams that induce less sample damage has also been a key development in enabling this technology to be used with fragile biological samples.2
Structural Problems
One of the reasons understanding the structures of biological species is so important is because the structure of a given protein influences the types of interactions that are possible with a therapeutic compound.3 Often, the drug design process uses structural information of proteins as a way of trying to predict which molecular shape a drug candidate will need to have to successfully bind and interact.
As the successful synthesis and trials of potential drug treatment are so expensive, drug discovery and design often rely heavily on modeling approaches to perform large-scale screening of candidates that are likely to be viable therapeutic treatments.
Having access to high-resolution accurate structures is therefore key for providing good starting information for the screening process. However, one of the issues most structural techniques face, including diffraction, crystallography, and cryo-EM, is that the experimental signal needs some type of analysis and post-processing to be converted into a series of atomic coordinates.
Solving Structures
In cryo-EM, a density map on the detector is measured, related to the electrostatic potential of the target. Within this data is an averaging of all the possible dynamical configurations of the protein that it has been able to sample in the image exposure time.4 Although the cryo-cooling inherent to the cryo-EM process does limit some of the dynamical configuration samplings that is possible within the exposure time, particular labile regions of the protein can still undergo some conformational change.
This structural flexibility introduces a degree of uncertainty into the fitting procedures for the density maps as the measured signal will represent an average of several structural conformers. The probability of a given conformer being sampled is proportional to how energetically accessible it is, so some structures may only be seen as very rare events.
Recent work published in Matter has looked at how to model this structural flexibility using a number of techniques, including cryo-EM X-ray and nuclear magnetic resonance (NMR).
The researchers found that for membrane and soluble proteins, the optimal approach is to consider an ensemble of structures. From this, it is possible to achieve better fitting for the higher resolution areas while understanding some of the conformational dynamics that contribute to the fuzzier regions of the image.
Inhomogeneity
In cryo-EM, there is an issue of heterogeneity in the signal, where some regions of the protein may have a better definition than others. This means that when comparing a cryo-EM image to a database of structures, different regions of the image can be fitted with varying degrees of confidence. Many cryo-EM datasets are largely of poorer resolution than other structural techniques such as protein crystallography, which can further enhance poor definition.
To combat this, the team has developed CryoFold, a multiphysics algorithm that can be used to investigate different equilibrium ensembles of folded proteins from cryo-EM data. It uses molecular dynamics methods and, to allow for sampling of rare events that could lead to more unusual configurations, a Bayesian engine for working with incomplete datasets.
The structures resulting from the molecular dynamics simulations have an electron-density map calculated from them that is then compared to the experimental map. The structure and result maps are refined to improve the guess at the protein configuration.
It is the inclusion of both the molecular dynamics approaches with the Bayesian engine that makes it possible to explore how changes in the protein secondary structure might affect the resulting density maps.
From these modeling approaches and cryo-EM databases even of poor-quality images, the CryoFold algorithms can search and refine both the individual molecular dynamics models as well as the whole ensemble of configurations that may be represented by a single cryo-EM density map. This enhanced structural information can then be used to better understand the function of proteins and how to target them with therapeutics.
References and Further Reading
- Callaway, E. (2020). The protein-imaging technique taking over structural biology. Nature, 578, 201. https://media.nature.com/original/magazine-assets/d41586-020-00341-9/d41586-020-00341-9.pdf
- Dubochet, J., Frank, J., & Henderson, R. (2018). Cryo-EM in drug discovery: achievements, limitations and prospects. Nature Publishing Group, 17(7), 471–492. https://doi.org/10.1038/nrd.2018.77
- Gallina, A. M., Bork, P., & Bordo, D. (2014). Structural analysis of protein-ligand interactions : the binding of endogenous compounds and of synthetic drugs. Journal of Molecular Recognition, 27, 65–72. https://doi.org/10.1002/jmr.2332
- Shekhar, M., Terashi, G., Gupta, C., Dill, K., Perez, A., Shekhar, M., Terashi, G., Gupta, C., Sarkar, D., Debussche, G., Horn, W. D. Van, Tajkhorshid, E., Kihara, D., Dill, K., & Perez, A. (2021). Article CryoFold : Determining protein structures and data-guided ensembles from cryo-EM density maps CryoFold : Determining protein structures and data-guided ensembles. Matter, 4(10), 3195–3216. https://doi.org/10.1016/j.matt.2021.09.004
Disclaimer: The views expressed here are those of the author expressed in their private capacity and do not necessarily represent the views of AZoM.com Limited T/A AZoNetwork the owner and operator of this website. This disclaimer forms part of the Terms and conditions of use of this website.