Only around 12% of American waste is converted to energy by waste-to-energy plants, and more waste goes to landfills than any other destination despite recent increases in composting and recycling rates. Lehigh University researchers have received a $3.5 million grant to develop rapid waste sorting using advanced spectroscopy and artificial intelligence (AI) technologies.
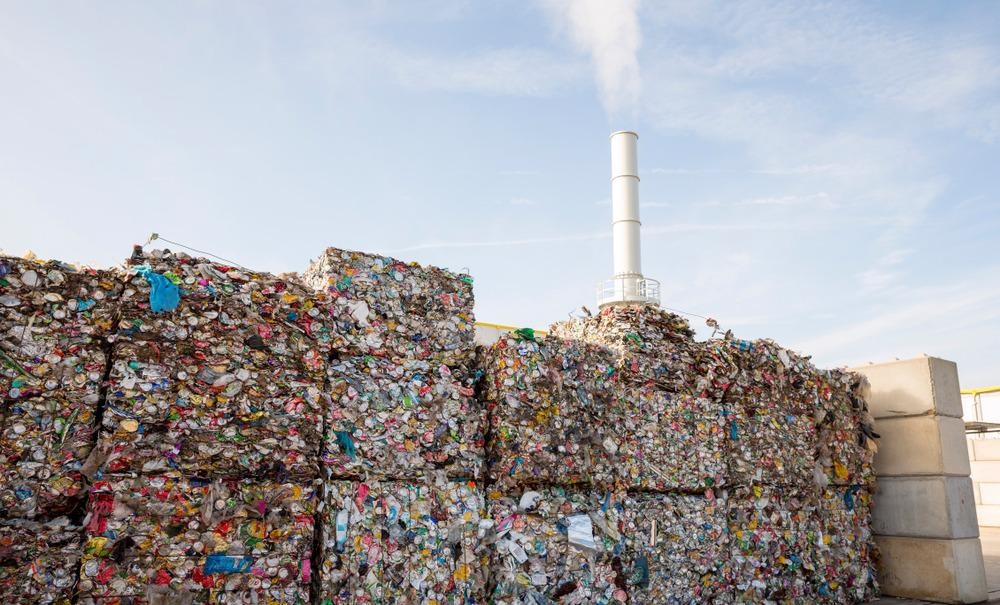
Image Credit: Belish/Shutterstock.com
The new system being developed at Lehigh’s Energy Research Center (ERC) in Pennsylvania, US, will provide rapid detection and analysis of municipal solid waste streams with advanced technologies.
The aim of this research is to make waste-to-energy plants more cost-effective, therefore increasing capacity in the waste-to-energy industry as investment rises to meet demand.
Collaborative Approach
Lehigh’s ERC is leading the research into AI-based waste analysis with spectroscopy, but the team working on the project represents a broad mix of stakeholders.
The US government’s Department of Energy (DOE) granted the $3.5 million funds to get the project going, which are part of a $34 million program from the DOE’s Bioenergy Technology Office (BETO) of the Office of Energy Efficiency & Renewable Energy (EERE). The BETO program is intended to support high-impact research and development for better biofuels, biopower, and bioproducts.
Lehigh’s team will include the private firm, the Energy Research Company (ERCo), the DOE’s National Energy Technology Laboratory (NETL), biorefinery facilities equipment manufacturer ThermoChem Recovery International (TRI), waste-to-energy producer Covanta Energy, the University of Toledo, and a consulting partner.
The project aims to optimize municipal solid waste conversion processes. These currently pose significant challenges for waste-to-energy producers, due to their variable physical, chemical, and biological characteristics.
How to Optimize Municipal Solid Waste Conversion
The project will streamline waste material analysis, one of the most complex parts of the whole waste-to-energy process. It will introduce AI to laser-induced breakdown spectroscopy (LIBS) and Raman spectroscopy data streams to rapidly analyze municipal waste streams.
The fast, in-situ characterization the researchers hope to achieve can provide critical characterization and chemical analysis data for municipal solid waste feedstock in just a few minutes.
This data then informs feed-forward process control downstream from the analysis, telling sorting machines and robots how to sift the waste.
The researchers expect to improve characterization throughput over baseline methods for municipal solid waste characterization by at least 25%.
To achieve this, new hardware and software elements need to be designed, as well as integrating systems to make them work together. But if the team is successful, waste materials could be processed for energy production in minutes instead of hours.
Raman Spectroscopy and Laser-Induced Breakdown Spectroscopy (LIBS)
The new waste characterization method will use advanced Raman as well as LIBS spectroscopy technologies.
Raman spectroscopy is a technique used to determine vibrational modes of molecules, as well as other modes. In chemistry, it is used to obtain a spectroscopic “fingerprint” of materials to determine exactly which molecules are present.
Raman spectroscopy devices such as the Renishaw structural and chemical analyzer (SCA) (which unites two well-established technologies, scanning electron microscopy (SEM) and Raman spectroscopy) do this with an emitted source of light, creating a Raman scattering effect. The colors that are scattered indicate precisely what molecules are present in the material.
LIBS is a kind of atomic emission spectroscopy that uses a highly energetic laser pulse as an excitation source, forming plasma. The plasma atomizes and excites the material under inspection. Plasma only starts forming when the focused laser gets to a specific threshold for optical breakdown. This threshold depends on the environment and the target material.
Combining Spectroscopy with AI
ERC and ERCo have already worked together to combine LIBS and AI for better coal analysis for power generation. The AI neural networks employed in that project improved measurement accuracy and correlated elemental composition to other high-order parameters.
This approach has been successful elsewhere. French scientists in 2018 developed a machine-learning program to predict how atoms react to an applied magnetic field. The program was combined with nuclear magnetic resonance (NMR) spectroscopy, and the team’s successful results were published in Nature Communications.
An interdisciplinary team from Finland and Denmark published results of a deep learning project to predict molecular excitation spectra instantly in Advanced Science in 2019. They showed how 10,000 previously unseen organic molecules’ structures were scanned and instant spectra predictions obtained almost instantly, enabling the identification of molecules for potential applications in materials science.
A 2021 paper in PNAS by Chinese researchers demonstrated AI-based spectroscopic monitoring of interactions between SARS-CoV-2 and human Angiotensin-converting enzyme 2 (ACE2) receptors. They showed that time-resolved spectroscopy could monitor structural information such as this with the help of machine learning.
Next Steps for Lehigh’s Waste-to-Energy Project
The Lehigh project hopes to make waste-to-energy a more attractive alternative to landfills, which is the cheapest option available to municipal budgets dealing with solid waste. This would support BETO’s goal of innovating to speed up feedstock technologies and propel a bioeconomy.
References and Further Reading
AI to increase waste-to-energy production. (2021) Waste Management World. Available at: https://waste-management-world.com/a/ai-to-increase-waste-to-energy-production.
Award for using AI and spectroscopy to increase waste-to-energy production. (2021) EnviroTec. Available at: https://envirotecmagazine.com/2021/10/11/award-for-using-ai-and-spectroscopy-to-increase-waste-to-energy-production/.
Candeias, A. and J.M. Madariaga (2019) Applications of Raman spectroscopy in art and archaeology. Journal of Raman Spectroscopy. https://doi.org/10.1002/jrs.5571.
Ghosh, K., et al (2019) Deep Learning Spectroscopy: Neural Networks for Molecular Excitation Spectra. Advanced Science. https://doi.org/10.1002/advs.201801367.
Gornushkin, I.B. et al. (2004) Microchip Laser-Induced Breakdown Spectroscopy: A Preliminary Feasibility Investigation. Applied Spectroscopy. https://doi.org/10.1366/0003702041389427.
Paruzzo, F.M., et al. (2018). “Chemical shifts in molecular solids by machine learning.” Nature Communications. Available at: https://doi.org/10.1038/s41467-018-06972-x.
Ye, S., G. Zhang, and J. Jiang (2021). “AI-based spectroscopic monitoring of real-time interactions between SARS-CoV-2 and human ACE2.” PNAS. Available at: https://doi.org/10.1073/pnas.2025879118.
Disclaimer: The views expressed here are those of the author expressed in their private capacity and do not necessarily represent the views of AZoM.com Limited T/A AZoNetwork the owner and operator of this website. This disclaimer forms part of the Terms and conditions of use of this website.