In a study published in Biosensors, a label-free and rapid identification technique based on artificial intelligence (AI)-assisted terahertz imaging is proposed to identify the therapeutic effect of low-level laser therapy for acute lung injury.
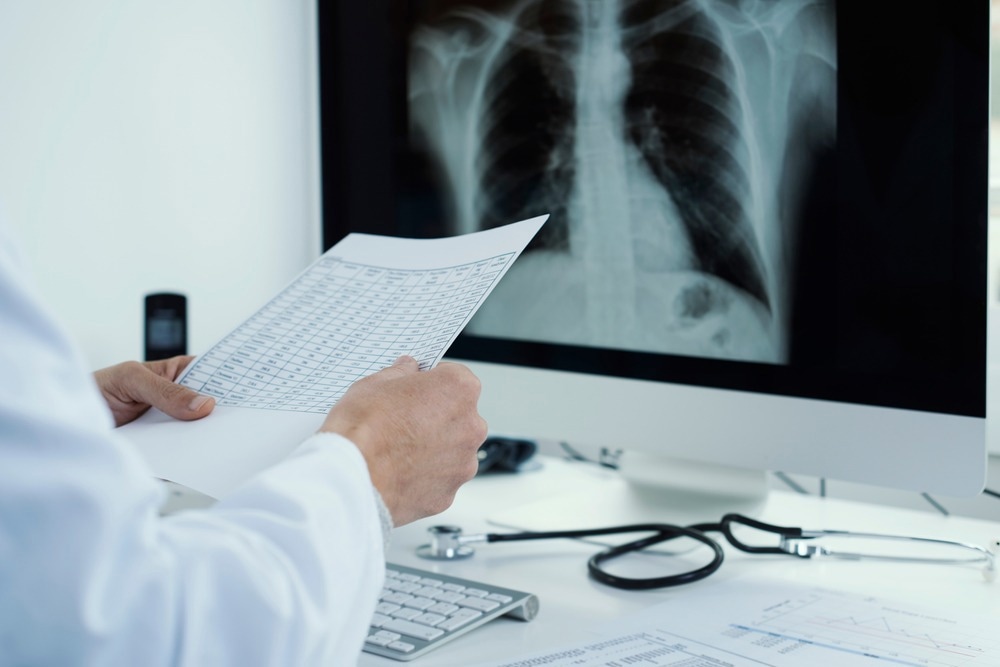
Study: Artificial Intelligence-Assisted Terahertz Imaging for Rapid and Label-Free Identification of Efficient Light Formula in Laser Therapy. Image Credit: nito/Shutterstock.com
What is Acute Lung Injury?
Acute lung injury is a severe respiratory failure characterized by hypoxemia and the development of bilateral infiltrates on chest radiographs and is sometimes referred to as acute respiratory distress syndrome.
Acute lung injury continues to substantially cause high mortality and morbidity in critically sick patients.
Low-Level Laser Therapy Treatment for Acute Lung Injury
Due to the ineffectiveness of current therapies for acute lung injury, it is critical to develop an effective therapeutic approach to significantly lower mortality and enhance the life expectancy of patients.
Low-level laser therapy holds significant potential as a noninvasive therapeutic technique in biomedicine. The therapeutic use of low-level laser therapy was documented about 50 years ago. Since then, it has been an effective treatment for traumatic and inflammatory diseases with fewer side effects than similar therapies.
The therapeutic efficacy of low-level laser therapy is primarily determined by specific light formulae, including irradiation time and wavelength. However, current techniques for identifying effective light formulae are complex and time-consuming.
Therefore, developing label-free, highly sensitive, and rapid identification methodologies will significantly boost the advancement and use of low-level laser therapy.
Identifying the Therapeutic Effect of Low-Level Laser Therapy with Terahertz Imaging
Terahertz imaging has received a lot of interest as a potential radiological technique in biomedical applications. This is because it has high diagnostic safety and sensitivity and a unique IR fingerprint spectrum.
Terahertz imaging has investigated various disorders, such as skin burns, traumatic brain damage, arthritis, silkworm egg development phases, and various cancers. This makes it capable of quantifying cellular and tissue processes and tracking the development and remission of disease to assess the treatment efficacy.
However, hardware constraints in imaging systems restrict the use of terahertz imaging for biological diagnostics. For example, the major hindrance is the slow imaging speed resulting from the low imaging resolution and severe blurring.
Therefore, enhancing the sharpness and clarity of terahertz imaging while decreasing the imaging duration is essential for rapid image analysis.
Using AI Algorithms to Improve Identification Time of Terahertz Imaging
Automatic identification methodology based on a voting classifier and rapid super-resolution (SR) image reconstruction were implemented to design and improve the label-free terahertz imaging-based artificial intelligence identification method.
Using low-level laser therapy, the therapeutic effects of acute lung injury were determined by experimenting with various irradiation times and wavelengths. Vertical reflecting terahertz imaging systems and their gray histograms at 0.14 terahertz imaging were designed as the biophysical features to determine the treatment impact based on the sensitive terahertz spectrum response of acute lung injury.
The gray histograms were then processed with feature extraction algorithms, such as principal component analysis (PCA).
Lastly, the peak signal-to-noise ratio, mean square error, and structural similarity were applied to compare the performance of the four SR reconstruction algorithms implemented in rapid terahertz imaging: enhanced super-resolution generative adversarial network (ESRGAN), super-resolution generative adversarial network (SRGAN), enhanced deep residual network (EDSR), and residual channel attention network (RCAN).
Significant Findings of the Study
Although scanning terahertz imaging offers higher image resolution and needs only half the time of the systematic biological measurements in identifying light formulae, it is still not fast enough for real-time identification.
On the other hand, the modified terahertz camera imaging technique offers a rapid imaging rate, but its poor resolution restricts its biological application. Therefore, this study developed a super-resolution imaging reconstruction-based rapid terahertz imaging technology to minimize imaging time without compromising image resolution.
The proposed approach enables fast and label-free identification of the effective low-level laser therapy light formula for acute lung injury. It demonstrated 91.22% accuracy and 33 seconds identification time, 400 times faster than the biological method and over 200 times faster than the scanning terahertz imaging technique.
The terahertz spectral response of acute lung injury revealed an impressive absorption peak in the 0.1-0.15 THz region.
AI-assisted terahertz imaging was solely employed to identify biological tissue samples encased in paraffin. However, it could be applied to develop and enhance other biological techniques, such as photodynamic therapy.
The AI-assisted terahertz imaging can be improved by integrating super-resolution and compressed sensing algorithms.
Reference
Shi, J., Guo, Z., Chen, H., Xiao, Z., Bai, H., Li, X., Niu, P., & Yao, J. (2022) Artificial Intelligence-Assisted Terahertz Imaging for Rapid and Label-Free Identification of Efficient Light Formula in Laser Therapy. Biosensors. https://www.mdpi.com/2079-6374/12/10/826
Disclaimer: The views expressed here are those of the author expressed in their private capacity and do not necessarily represent the views of AZoM.com Limited T/A AZoNetwork the owner and operator of this website. This disclaimer forms part of the Terms and conditions of use of this website.