A study published in the journal Cells proposes a lacunarity-based quantitative analysis method for assessing the nanoscale cellular structure and homogeneity of target molecules in single-molecule localization microscopy. The proposed method provides accurate and fast information about the subcellular structure of biological samples.
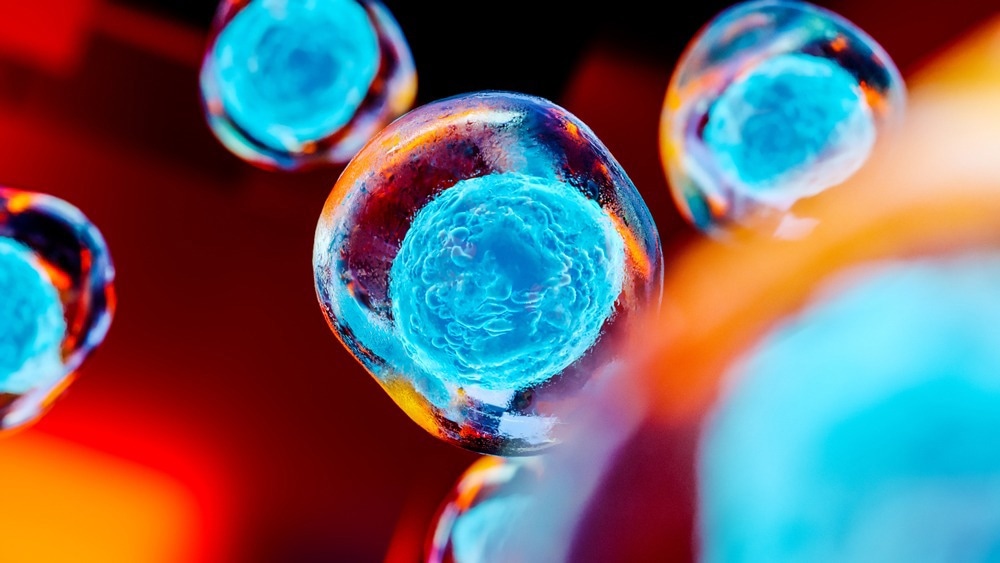
Study: Application of Lacunarity for Quantification of Single Molecule Localization Microscopy Images. Image Credit: pinkeyes/Shutterstock.com
Limitations of Conventional Optical Microscopes
Conventional microscopes have a resolution of 200 nanometers. The diffraction of light restricts their spatial resolution. Below this spatial resolution, images become blurry and cellular structures cannot be distinguished. This implies that conventional microscopy cannot detect most subcellular structures and molecular complexes.
Single Molecule Localization Microscopy: A High-Resolution Fluorescence Microscopy Technique
Various high-resolution microscopy techniques have been developed to overcome conventional microscopes' shortcomings, such as stimulated emission depletion (STED) microscopy, structured illumination microscopy (SIM), and single molecule localization microscopy (SMLM).
The SMLM techniques are more precise than other microscopy techniques in determining the locations of single-emitting fluorophores. These powerful microscopy techniques employ the blinking of fluorescent molecules to detect their position with precision below the diffraction limit on the scale of tens of nanometers.
Some of the most common SMLM techniques employed in biology are photo-activated localization microscopy (PALM), direct stochastic optical reconstruction microscopy (dSTORM), and ground state depletion microscopy (GSDM).
Quantitative Analysis of SMLM
The quantification of SMLM images and datasets has attracted a lot of attention. However, its unique 3D data format necessitates new evaluation algorithms, merit functions, and visualization techniques.
Various techniques have been suggested to quantify target molecules' density and underlying structure in SMLM.
Cluster analysis techniques
SMLM images are generated by localization points indicating the positions of individual fluorophores linked to the target molecules, such as proteins. Cluster formation is a common configuration of these proteins.
Cluster analysis techniques such as Voronoi tessellation and DBSCAN are frequently used when clusters' composition, area, and size can be directly evaluated from the raw localization data.
However, these techniques rely on predetermined parameters, and their operations can be computationally demanding.
Lacunarity analysis
Lacunarity analysis is a multi-scale method that has been successful in material sciences. It was primarily employed in fractal analysis to differentiate between fractals with different structures but the same dimensions.
Lacunarity can identify patterns at multiple scales flexibly and systematically. This makes it a viable tool for defining the structure of subcellular systems unraveled by SMLM.
Using Lacunarity Algorithm for Quantitative Analysis of SMLM Datasets
An algorithm for calculating lacunarity was developed using the gliding-box technique. TestSTORM, a dSTORM simulation software, developed test data in which the target molecules were organized into various clusters of nanofoci to replicate the real-life samples.
A modified disc pattern generator randomly distributed epitopes in a circular area at a specified orientation and density and generated 8000 frames for the simulated data. The samples were not drifted, and a Gaussian point spread function was applied.
The rainSTORM reconstruction program performed a multi-Gaussian 2D analysis to capture each blinking event.
The size of clusters, the number of clusters in the region of interest, the distance between adjacent clusters, the size of nanofoci, the density of nanofoci, localization density in a cube micron volume, and the number of localizations per nanofoci were evaluated five times at different values.
Significant Findings of the Study
SMLM data processing has always been computationally challenging due to the size of the datasets. However, the proposed lacunarity algorithm can quantitatively assess homogeneity and subcellular structures in SMLM data.
The lacunarity algorithm can provide precise information on the structure of SMLM data more quickly than cluster analysis. Therefore, the lacunarity data can be analyzed and used for quantitatively determining the structured localization of SMLM data using computed and simulated datasets. In addition, the visualization technique enables instantaneous evaluation of the image's relative homogeneity.
Practical scope
DNA double-strand break (DSB) is a harmful lesion that must be repaired as fast and effectively as possible to minimize chromosomal loss and translocation.
The DNA repair proteins form foci around the DSB to carry out the repair processes. The foci size is in the region of 100 nanometers, making the high-resolution SMLM essential for comprehending the process of DNA repair.
The proposed method demonstrates that the DSB-inducing chemical agents and radiation increase the frequency of DSBs and lead to the formation of bigger repair foci.
Limitations
The lacunarity algorithm uses a single value to describe the structure of a sample of a particular size. This implies that distinct mechanisms can have a significant effect on lacunarity.
In experimentation, the lacunarity curve responded similarly to an increase in cluster number or size. Therefore, lacunarity data cannot be interpreted without a preliminary computational and simulation analysis.
Reference
Kovács, B.B.H., Varga, D., Sebők, D., Majoros, H., Polanek, R., Pankotai, T., Hideghéty, K., Kukovecz, Á., & Erdélyi, M. (2022). Application of Lacunarity for Quantification of Single Molecule Localization Microscopy Images. Cells. https://www.mdpi.com/2073-4409/11/19/3105
Disclaimer: The views expressed here are those of the author expressed in their private capacity and do not necessarily represent the views of AZoM.com Limited T/A AZoNetwork the owner and operator of this website. This disclaimer forms part of the Terms and conditions of use of this website.