In an article published in Remote Sensing, researchers developed a hybrid rule-based and machine learning methodology using a combination of Sentinel multispectral and Synthetic Aperture Radar (SAR) composite imagery to provide the first national-scale pixel-based landcover classification for the open coast of New Zealand.
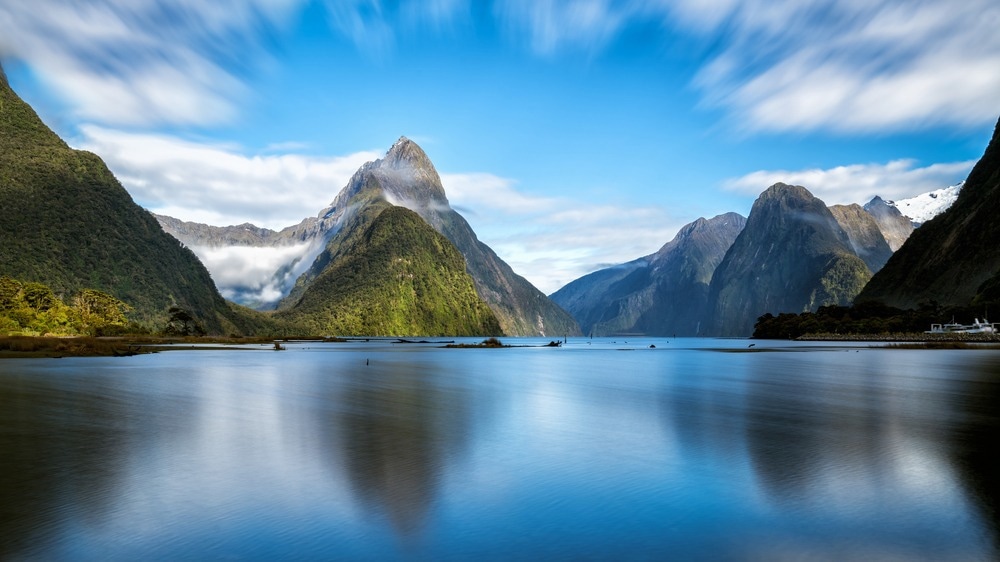
Study: A Methodology for National Scale Coastal Landcover Mapping in New Zealand. Image Credit: Blue Planet Studio/Shutterstock.com
Coastal areas are susceptible to environmental and anthropogenic dangers, which may emerge at many temporal and geographical dimensions. Coastal adaptation is a major worldwide problem, and human-induced climate change is one of the main drivers of coastal change.
Shoreline change studies are essential to such practices since coastal monitoring is crucial in planning efforts to minimize and adapt to future risks such as erosion and floods.
Monitoring Beach Geomorphological Characteristics
Through repeated beach surveys or visual analysis of aerial photographs, remote sensing and land surveying methods have been employed to track change.
Beach geomorphological characteristics, such as the location of the coastline up to regional scales, may be monitored using in situ or aerial data collected from video imaging or light detecting and ranging (LiDAR) sensors. However, the cost of acquiring such data may restrict its temporal and geographical coverage.
Historical aerial picture analysis may provide information at a larger global scale, but it is limited by the temporal resolution and quality of the data.
Cloud Computing Platforms Improve Accessibility to Satellite Data Repositories
Increased access to earth observation satellite datasets offers worldwide coverage at spatial and temporal resolutions ideal for mapping shorelines.
Accessibility to these data repositories has been improved further by the advent of cloud computing platforms, which now provide analysis-ready imagery that is orthorectified, atmospherically corrected, and simple to query in space and time.
New methods to track change along the shore have been developed. Most current efforts have concentrated on deriving shoreline position with accuracy exceeding the native resolution of the sensor using interpolation techniques, which has allowed for the extraction of shoreline proxies/indicators with sub-pixel accuracy from publicly accessible multispectral earth observation data archives such as Sentinel or Landsat.
Designing a Hybrid Rule-Based and Supervised Machine Learning Classification Methodology
This study aims to design and test a hybrid rule-based and supervised machine learning classification methodology that, using all 2019 Sentinel-1 (S1) and Sentinel-2 (S2) data, identifies coastal-specific land cover classes for the New Zealand coastal zone. Annual composite photos were created and utilized in a per-pixel classification approach that uses a hierarchical system of rules to distinguish between different forms of spectrally diverse land cover.
The remaining spectrally related groups were identified using supervised machine learning using spectrum and SAR data. This was developed using a manually compiled national-scale training dataset for coastal regions. The finished output was compared to the outcomes of an earlier per-pixel strategy.
In the context of monitoring coastal change at various geographical and temporal scales, the methodology's applicability was assessed, and the temporal and spatial constraints were examined.
Using GEE to Produce SAR and Multispectral Composite Photos
To produce SAR and multispectral composite photos for 2019, Google Earth Engine (GEE) was utilized to process the data from S1 and S2.
The year 2019 was chosen since it was the most recent year for which national-scale satellite imagery and the supporting data needed to verify and train the classifier were available.
Composites were created by combining the pixel values from many photographs taken during a specific period. Instead of compressing data to concentrate on a specific shoreline proxy, pixel-based techniques evaluate change throughout the coastal zone.
To evaluate change throughout the coastal zone rather than only the change in the location of the coastline and decrease environmental uncertainty in change analysis, accurate identification of land cover types is the first step in per-pixel change detection. This method can minimize errors related to cloud cover and maritime processes (waves and tides) that cause short-term and episodic volatility.
Significant Findings of the Study
A coastal landcover cover classification for the New Zealand coastline was created by mapping coastal areas according to the major sediment types using a mix of open-source multispectral and synthetic-aperture radar data. When validation data was normalized by class area, overall accuracy approached 90%. The categorization procedure was created using free, open-source programs and code, making it adaptable to other situations.
The accuracy was 30% higher overall than earlier methods, emphasizing the need to consider coastal settings' complexity.
Combining SAR and multispectral earth observation sensor types improved accuracy for coastal-specific sedimentary classes (10–50%), yielding reliable findings.
Future Prospects
To better equip decision-makers and coastal scientists with knowledge to prepare for upcoming change at the coast, this technique may be expanded further with change-detection analyses delivering insights into long-term land cover change at the coast in New Zealand and worldwide.
Reference
Benedict Collings, Murray Ford and Mark Dickson (2022) A Methodology for National Scale Coastal Landcover Mapping in New Zealand. Remote Sensing. https://www.mdpi.com/2072-4292/14/19/4827/htm
Disclaimer: The views expressed here are those of the author expressed in their private capacity and do not necessarily represent the views of AZoM.com Limited T/A AZoNetwork the owner and operator of this website. This disclaimer forms part of the Terms and conditions of use of this website.