In a study published in the European Journal of Remote Sensing, researchers proposed a two-step classification method for mapping non-photosynthetic vegetation or crop residue using spectroscopic and machine learning techniques from the spaceborne imaging spectroscopy PRISMA dataset.
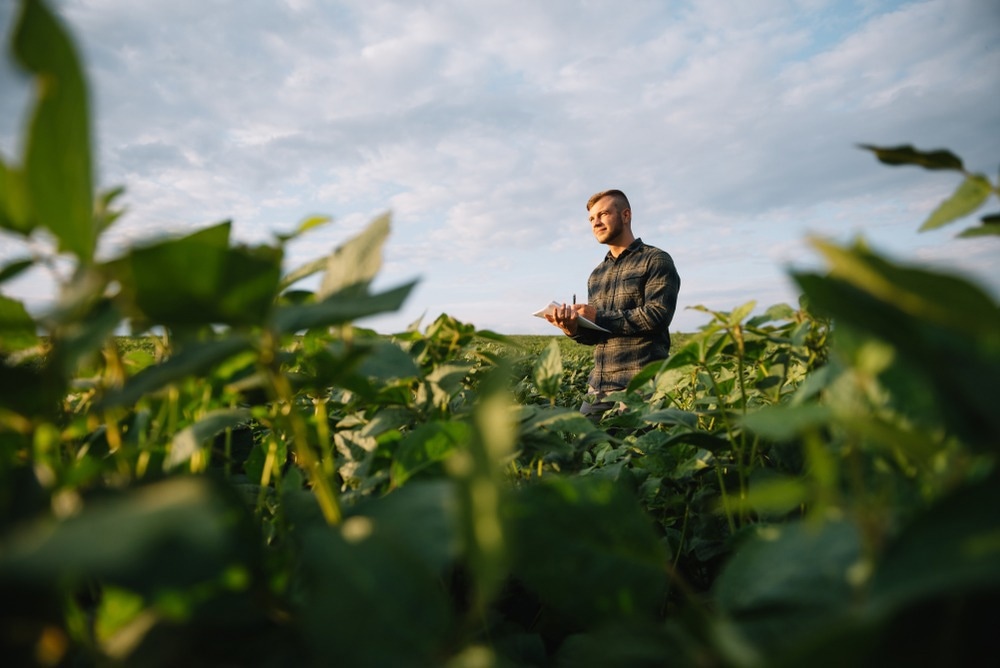
Study: Mapping spatial distribution of crop residues using PRISMA satellite imaging spectroscopy. Image Credit: Hryshchyshen Serhii/Shutterstock.com
Non-Photosynthetic Vegetation and Crop Residue in Agriculture
Non-photosynthetic vegetation refers to vegetation that cannot use photosynthesis for its growth and development. It mainly comprises plant litter, dead or dying vegetation, and crop residues.
Crop residue and dying vegetation are essential in carbon farming and sustainable agriculture. Their significance stems from their roles in the carbon, nitrogen, and water cycles and soil conservation.
The presence of crop residue on the fields protects the soil from erosion, regulates temperature and moisture levels, enhances soil organic carbon, minimizes soil compaction caused by agricultural machines, and improves soil structure.
The relative abundance of crop residue indicates agricultural management practices since it is associated with crop rotations, tillage, and harvesting techniques.
Hyperspectral Remote Sensing of Crop Residue or Non-Photosynthetic Vegetation
Aerial and satellite remote-sensing systems can identify and evaluate NPV more quickly, accurately, and objectively than conventional field operator-based reporting. By mapping the availability and quantity of NPV, remote sensing can assist in monitoring and controlling the implementation and effectiveness of agricultural conservation policies.
Various non-photosynthetic vegetation remote sensing techniques in agricultural settings rely on spectral variations between crop residues, bare soil, and green vegetation to perform class detection.
In particular, they employ multispectral broadband data to estimate spectral indices for NPV mapping.
PRISMA mission
Previous non-photosynthetic vegetation research using hyperspectral data relied on terrestrial and aerial data due to the limited availability of spaceborne sensors. Therefore, they could not differentiate NPV from different types of bare soils, particularly in the near-infrared spectral and visible domain.
However, the necessary continuous bands can be provided by imaging spectrometers to detect NPV and differentiate it from bare soils.
The PRISMA satellite mission is one of the latest spaceborne imaging spectroscopy missions for Earth Observation (EO), and its first pillar application is agriculture.
PRISMA is a precursor to the next generation of hyperspectral missions; it targets specific EO applications relating to environmental preservation, monitoring, and sustainable development.
However, PRISMA's 30-kilometer coverage and lengthy return interval might not be ideal for operational monitoring. Despite such restrictions, acquisitions should be frequent enough for in-season research.
Spectroscopic and Machine Learning Techniques for Mapping Crop Conditions
This study aims to build a framework for categorizing distinct field conditions using hyperspectral satellite data to generate a reliable map for quantitative NPV, crop residue evaluation, and field condition monitoring over time.
A two-step classification method is developed for mapping crop conditions based on PRISMA data from spaceborne imaging spectroscopy.
First, four diagnostic spectral intervals are modeled using Exponential Gaussian Optimization such that the hyperspectral space is limited to the model parameters. Second, a pixel-level decision tree (DT) is trained to categorize crop residues, bare soil, standing dead vegetation, and emerging and green vegetation.
The categorization results are then combined at the parcel level to provide thematic maps for monitoring and assessing farm-level agro-practices.
Significant Findings of the Study
Crop residue mapping is precise at pixel and parcel levels (overall accuracy > 90%; K > 0.9). Results demonstrated that PRISMA data is adequate for parcel-scale mapping of crop conditions.
The accuracy of the forecasts has been evaluated and analyzed based on the change in trajectories of target conditions over time, which is also relevant for tracking soil conservation activities. This investigation of trajectories demonstrated that categorization outcomes are compatible with independent data and verified the method's efficacy for mapping field conditions.
It also demonstrates that using machine learning classifiers in conjunction with spectroscopic approaches confined by the known optical characteristics of the examined surfaces results in a mapping methodology that is dependable and transferrable and can be used with time series data.
Limitations and future recommendations
In this study, classification supervision was performed once. The decision tree model was employed throughout a time series that includes several seasons, different types of crops, and various environmental variables. The train sample selection was image-based.
Although helpful in situations with no additional spectrum dataset, this strategy has several drawbacks.
A reference spectral library that includes a wide range of surface spectra recorded under controlled settings should be accessible to broadly adopt the decision tree model for various field situations and a quantitative examination.
The machine learning paradigm could be trained using these libraries to improve the splitting rules by considering various crops and agricultural conditions. The performance of several narrow-band remote sensors with potentially varied spectral designs can be tested using the same spectral libraries.
Multiple machine learning algorithms should be employed to enhance the categorization of PRISMA images for enhanced classification of NPV and crop residue in agricultural landscapes.
Reference
Monica Pepe, Loredana Pompilio, Luigi Ranghetti, Francesco Nutini & Mirco Boschetti (2022) Mapping spatial distribution of crop residues using PRISMA satellite imaging spectroscopy. European Journal of Remote Sensing. https://www.tandfonline.com/doi/full/10.1080/22797254.2022.2122872
Disclaimer: The views expressed here are those of the author expressed in their private capacity and do not necessarily represent the views of AZoM.com Limited T/A AZoNetwork the owner and operator of this website. This disclaimer forms part of the Terms and conditions of use of this website.