In an article published in Remote Sensing, researchers demonstrated a novel technique for bottom depth retrieval based on Hydrolight simulation. The proposed model's remote sensing reflectance (Rrs (l)) values were derived from the radiative transfer principle rather than accurate satellite data.
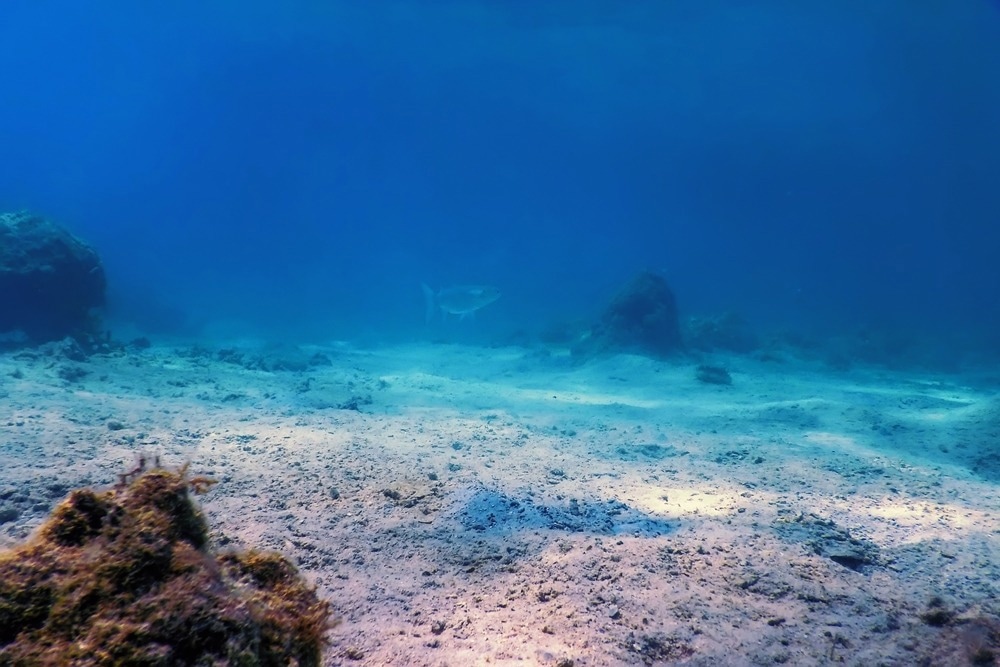
Study: Satellite-Derived Bottom Depth for Optically Shallow Waters Based on Hydrolight Simulations. Image Credit: Allexxandar/Shutterstock.com
The Hydrolight simulation approach also accounted for the fluctuating conditions of chlorophyll levels, water depth, and bottom reflectance. A data-driven machine learning (ML) approach based on the random forest (RF) concept was used to determine the bottom depth from Rrs (l).
For the validation and training datasets, the root mean squared error (RMSE) value was less than 0.4 m, and the determination coefficient (R2) was higher than 0.98. The Hydrolight simulation approach for bottom depth retrieval showed potential for use in various coastal areas while extending the range of benefits for satellite data. Specifically, using Sentinel 2 data, the authors calculated the bottom depth in three locations in the South China Sea, including Xincun Bay, the coasts of Wenchang City, and Huaguang Reef.
The bathymetric data obtained by the satellite remote sensing photon-counting lidar Ice, Cloud, land Elevation Satellite-2 (ICESat-2), which sufficiently identified the bottom depth in shallow waters, was used to verify the derived bottom depth.
The genuine bottom depth and expected bottom depth were in good agreement, and large-scale mapping made up for the shortcomings of the along-track ICESat-2 data. This general-purpose bottom depth retrieval model based on ICESat-2 data could be successfully used with high-spatial-resolution imagery such as Sentinel 2 to enable bottom depth mapping in optically shallow water (OSW) under various conditions.
Coastal Benthic Habitats and Environmental Sustainability
The movement of nutrients and energy, the carbon balance, and the state of the global environment are all significantly influenced by coastal benthic habitats such as seagrass and coral reefs. These delicate ecosystems are in danger due to global climate change and are severely prone to deterioration. Human activities, notably the effects of sediment loading and terrestrial nutrient loading, pose an increasing threat to them.
Bottom depth measurements are crucial for mapping and health status monitoring of these dynamic ecosystems and transportation and nautical navigation. Even though the bathymetric data for these ecosystems is significant, typical field measures such as shipborne sounding or aerial lidar frequently fail to provide precise bottom depth estimations.
Due to its high temporal resolution, sizeable spatial coverage, and high spatial resolution, satellite remote sensing plays a vital role in coastal shallow water bottom depth estimation. The bottom signals are reflected in the remote sensing reflectance (Rrs (l)) and the water-leaving radiance signatures for OSWs. Clean waters are optically shallow, typically up to 20 meters, while in murky coastal waters, the OSW depth may imply one to three meters.
Satellite remote sensing-derived bathymetry methodologies have been widely used since they are cost-effective for quickly mapping depth in a vast area. The optical signatures reflected by the bottom water column and substratum features are collected by satellite remote sensing imagery for bottom depth retrieval. Today, satellite remote sensing accuracy is applied to the most advanced nautical charts.
The recently launched ICESat-2 offers precise along-track bottom depth measurement for OSWs when the light penetration is sufficient, owing to advancements in spaceborne lidar. As a result, the ICESat-2 depth data can be used for worldwide research applications and overcome the shortage of reference "seed depths." However, the high accuracy measurements of water depths collected from ICESat-2 are still insufficient for large-scale mapping because of their along-track observations only and their poor temporal resolution.
This study created a bottom depth retrieval model that could be easily modified for OSW bottom depth mapping. The proposed model used high-resolution satellite remote sensing imagery by using a variety of Hydrolight simulation datasets, such as bathymetry data from ICESat-2 and the corresponding satellite remote sensing reflectance. In particular, the Hydrolight simulation datasets were based on a radiative transfer numerical model for predicting the underwater light field in OSWs. The Hydrolight simulation datasets took into account a variety of relevant benthic type variables and water column parameters.
Finally, using the spectral satellite remote sensing reflectance indexes as the predictor variables, the authors applied the random forest model to retrieve the bottom depth. The precise bottom depth determined by ICESat-2 was used to validate the satellite-derived bottom depths.
Construction and Investigation
Sentinel 2 A and Sentinel 2 B were launched on June 23, 2015, and March 7, 2017. The twin satellites' multispectral instrument (MSI), which had a 290 km swath width, 13 spectral bands, frequent revisit capability, and high spatial resolution (10 m), offered a fresh insight into the natural ecology of the coast and the ocean.
The high spatial resolution of Sentinel 2 allowed it to record the intricate and minute features brought on by tidal changes, steep slopes, and changing bottom depth to estimate bottom depth.
The researchers chose three areas with different benthic habitats in the South China Sea to test the portability and precision of the OSW bottom depth detection algorithm to actual satellite data. These sites were chosen to reflect a range of bottom types, including sand, corals, seagrass, and clean water. In this case, the equivalent acquisition timeframes were similar for ICESat-2 data and Sentinel 2 imagery.
An extensive set of regression trees were combined in a random forest, an ensemble machine learning model. The random forest model could perform sophisticated regression and classification with high precision and judge the relative relevance of different variables. Due to the superior performance of random forest over linear regression, the random forest was used as a substitute for satellite-derived bathymetry. Water depth was the object variable in the random forest regression model, and Rrs (l) spectral characteristics were the independent variables.
A nonlinear relationship between Rrs (l) and water depth was effectively learned using the random forest machine learning technique based on the simulated Rrs (l) generated under various water depths, chlorophyll concentrations, and bottom reflectance in Hydrolight simulation. The proposed model was examined by employing Sentinel 2 high spatial resolution imageries in three South China Sea regions to recover the bottom depth of OSWs.
Significance of the Study
This study provided a novel bottom depth retrieval technique that considered the fluctuating variables of chlorophyll concentrations, water depth, and bottom reflectance and was based on Hydrolight simulation datasets.
The bottom depth retrieval model consistently performed well across three independent validation locations and the Hydrolight simulation datasets. Thus, the model confirmed the possible application of Hydrolight simulation datasets for studies on bottom-depth retrieval.
The center wavelength could be chosen for broader applications according to other multispectral and high-spatial-resolution or hyperspectral satellites. However, this investigation's Hydrolight simulation central spectral bands were identical to Sentinel 2.
Reference
Wang, Y., et al. (2022) Satellite-Derived Bottom Depth for Optically Shallow Waters Based on Hydrolight Simulations. Remote Sensing, 14(18), 4590. https://www.mdpi.com/2072-4292/14/18/4590
Disclaimer: The views expressed here are those of the author expressed in their private capacity and do not necessarily represent the views of AZoM.com Limited T/A AZoNetwork the owner and operator of this website. This disclaimer forms part of the Terms and conditions of use of this website.