Motion-blurred image restoration has always been extremely challenging due to the limitations of single-blurred image algorithms. A pre-proof study in Pattern Recognition has proposed a novel motion-blurred image restoration framework based on blur parameter estimation and fuzzy inference neural networks. The proposed restoration framework produces high-quality image restoration results.
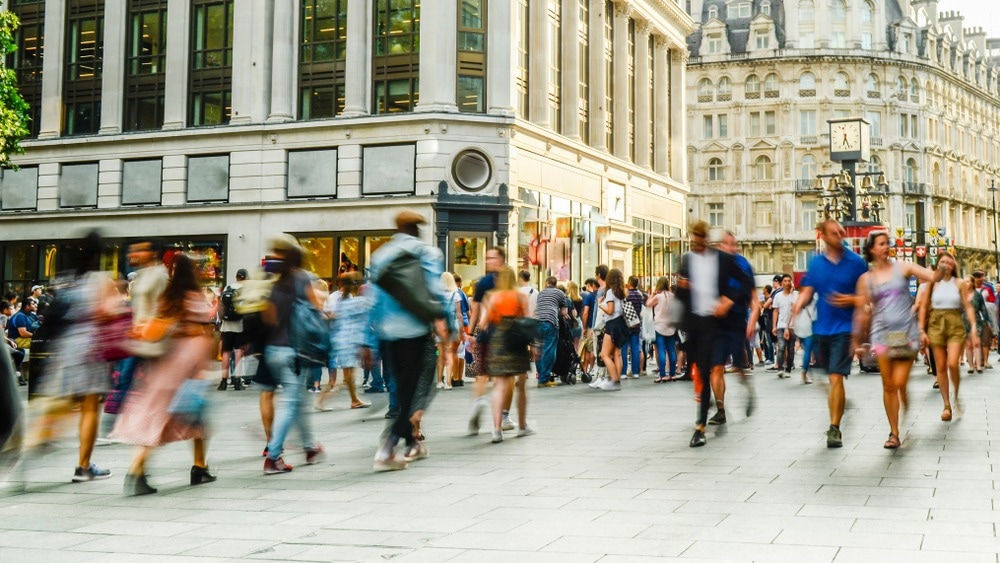
Study: Motion-Blurred Image Restoration Framework Based on Parameter Estimation and Fuzzy Radial Basis Function Neural Networks. Image Credit: William Barton/Shutterstock.com
Motion-Blurred Imaging
Camera vibration during the exposure time causes motion blur in the image. As one of the primary sources of image deterioration, it significantly influences the computer vision systems' performance in various domains.
Therefore, an efficient motion blur image restoration technology will aid in improving the dependability of relevant applications in medical imaging, aerospace, traffic surveillance, military search, public security, space, and satellite imaging.
Current Image Restoration Techniques
Deep learning is being used in some of the most recent technologies to predict the distribution of motion blur and recover degraded images. These deep learning techniques are classified into two types; the first employs a single image, while the second combines video and multi-frame images to restore the degraded image.
Point Spread Function (PSF) is used in neural modeling to create motion-blurred images from the source image. Therefore, motion-blur image restoration methods often try to reduce or remove PSF's impact on degraded images.
Early motion denoising research recommended effective algorithms for decoding blurry images or reversing image deterioration. These researches are separated into blind and non-blind deconvolution.
Numerous non-blind deconvolution algorithms recover images through a predefined PSF. These well-known algorithms are commonly used in the image restoration industry.
An efficient method for recovering motion-blurred images is the blind image deconvolution technique, which reconstructs the original shape using the PSF detected after estimating the motion parameters from the blurred image. However, this approach is more complicated than non-blind deconvolution and more decisive in predicting PSF.
Most blind image deblurring algorithms successfully estimate complicated blur kernels and give precise results. In actual applications, however, the computational framework of these approaches is challenging and time-consuming since several equations have to be solved.
Recently, several researchers have developed various PSF calculation techniques for blurred image restoration that concentrate on calculating blur angle and length.
Unfortunately, most approaches continue to yield poor accuracy and insignificant blur length estimations. Overall, current algorithms still struggle to strike a compromise between precision, time efficiency, and robustness.
Using Parameter Estimation and P-RBFNN Motion-Blurred Image Restoration Framework for Image Quality Assessment
Zhao et al. developed a blurred image restoration system in three steps, motion-blur image production, blur parameter estimation, and image quality assessment.
Eight range values for varying degrees of motion blur were set with defocus noise when producing motion-blurred images, simulating various levels of motion blur in natural scenes.
A parameter estimation approach based on PSO optimization was presented for calculating blur parameters. The approach used the pre-estimator to initialize the particle's 3D location based on the projected values of the two estimation techniques.
The particle space parameters were continuously updated using the naturalness image quality evaluator (NIQE) until the optimum estimated value was obtained. NIQE was also used as the fitness value function to determine the ideal particle site.
Regarding the assessment of image quality, the restored dataset was thoroughly examined by full-reference image quality assessment (FR-IQA) and no-reference image quality assessment (NR-IQA), both of which use the radial basis function neural network (RBFNN) algorithm as the no-reference quality assessor.
This framework evaluated image quality using the polynomial-based radial basis function neural network (P-RBFNN) classifier, which is unique from previous algorithms such as peak signal-to-noise ratio (PSNR), structural similarity (SSIM), and NIQE.
Important Findings of the Study
In this study, a thorough framework for motion-blurred image restoration was developed. The blur length, defocus radius, and angle parameters can be concurrently optimized using the proposed blur parameter estimation based on the particle swarm optimization (B-PSO) algorithm.
B-PSO performs perfectly in estimating blur angle or length parameters for F-type degenerate sets while maintaining the error within an acceptable range. The image set recovered by the proposed had higher quality and a higher classification rate.
After a series of experiments, it was determined that the suggested restoration framework could successfully recover motion-blurred images. The classification accuracy of the restored image was 96%, the structural similarity was 0.9044, and PSNR was 29.976dB. The results suggest that the proposed restoration approach outperforms traditional image restoration methods.
The performance of this algorithm can be further increased by combining the motion deblurring network with a deep learning network.
Reference
Shengmin Zhao, Sung-Kwun Oh, Jin-Yul Kim, Zunwei Fu, and Witold Pedrycz. (2022). Motion-Blurred Image Restoration Framework Based on Parameter Estimation and Fuzzy Radial Basis Function Neural Networks, Pattern Recognition. https://www.sciencedirect.com/science/article/abs/pii/S0031320322004630
Disclaimer: The views expressed here are those of the author expressed in their private capacity and do not necessarily represent the views of AZoM.com Limited T/A AZoNetwork the owner and operator of this website. This disclaimer forms part of the Terms and conditions of use of this website.