Food contact materials (FCMs) are challenging to identify because of their complicated matrices and restricted commercial standards. Using tools based on machine-learning algorithms can help identify these substances.
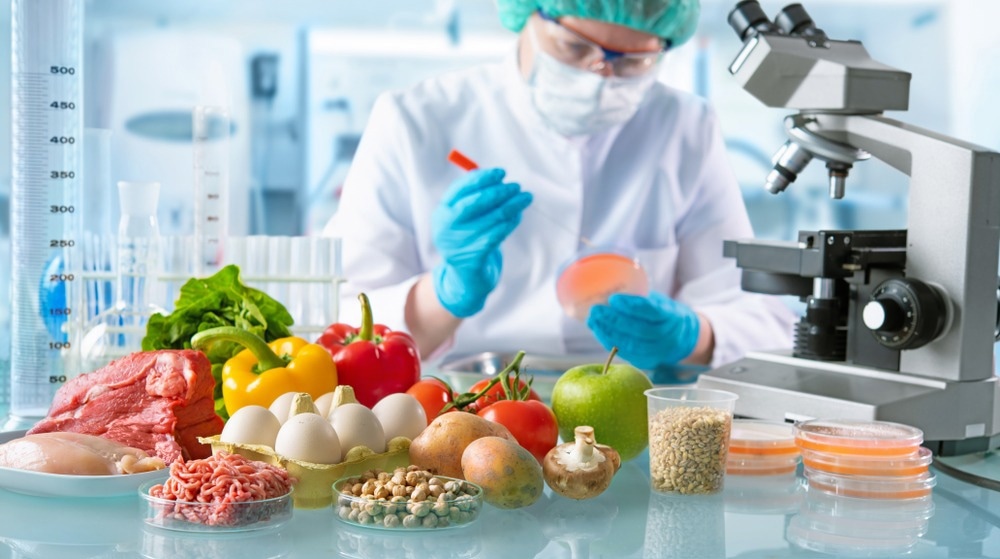
Study: Identification of Nonvolatile Migrates from Food Contact Materials Using Ion Mobility–High-Resolution Mass Spectrometry and in Silico Prediction Tools. Image Credit: Alexander Raths/Shutterstock.com
Recent research published in the Journal of Agricultural and Food Chemistry demonstrates the usage of in silico collision cross-section modeling (CSS) and retention time (RT) techniques to detect inert migrates from food contact materials using liquid chromatography-ion mass spectrometry. Investigating the chemical migration from polyamide (PA) spatulas helps determine this approach's suitability.
Detrimental Effects of Food Contact Chemicals (FCCs)
During processing, transportation, preservation and delivery, food and drinks can come into contact with various materials.
Chemicals in food contact materials can migrate into food and cause health issues for consumers.
In some instances, food-contact materials can also include unwanted chemicals (FCCs) such as the degradation products of additives, the polymer itself, industrial solvents, or the by-products of chemical reactions.
Paper, wood, glassware, metal, plastic, and biomaterials are all FCMs.
Plastic polymers most frequently come into contact with food. Additives are employed in plastics manufacturing to improve the finished product's performance. Plasticizers, inhibitors, binders, and lubricants are some of the most often used additives. These additives can migrate into food since they are not usually chemically bonded to the polymers.
It has been reported that the food contamination caused by chemical materials from FCMs could be 100 times greater than that from synthetic pesticides and industrial pollutants.
Challenges Associated with Identification of FCCs
Accurate detection of FCCs from FCMs is difficult due to the complexity of the plastic matrix, unknown contents, and restricted standards. The elemental composition of detected substances can be estimated from the primary ion and isotopic dispersion using high-resolution mass spectrometry (HRMS), incorporating time-of-flight (TOF).
Molecules with a specific chemical formula in ChemSpider or PubChem reveal several probable structures. Analysts with knowledge in MS spectrum interpretation are needed for elimination of implausible entries and to reduce false positives.
Potential of Ion Mobility Mass Spectrometry for FCCs Detection
In recent years, ion mobility spectroscopy has been extensively employed for the controlled and uncontrolled screening of complex samples.
Collision cross-section derived from ion mobility mass spectrometry is a stable characteristic associated with particle size, morphology, and potential.
Collision cross-section measurements combine retention time (RT), precise mass, atomic pattern, and fragmented ions for chemical identification.
Limitation of Collision Cross-Section Based Ion Mobility Mass Spectrometry
A constraint in detecting FCCs emerging from FCMs is that many suspected compounds are not commercially accessible. Consequently, accurate and definite identification cannot be validated by matching the collision cross-section values of unknowns to those of standard values.
Development of Collision Cross-section Prediction Models Using Machine Learning Approaches
To improve FCC detection by ion mobility mass spectrometry's, researchers have developed machine learning-based collision cross-section prediction models. Based on liquid chromatography, ion mobility mass spectrometry and collision cross-section (CCS) prediction tools, Song et al. have proposed a method to detect non-volatile chemicals in FCMs.
The Food Contact Chemicals Database (FCCdb) and the Chemicals linked with Plastic Packaging Database (CPPdb) are two databases with information on FCMs. The predicted models designed by researchers were employed to forecast the RT and CCS values of the chemicals in the CPPdb and FCCdb. The structural elucidation method included the two databases as screening libraries.
By analyzing the migration of chemicals from PA spatulas, the efficacy of these two databases in detecting FCCs were assessed.
After using the RT and CCS prediction filters, the number of relevant compounds was decreased by around 75% and 29%, respectively. The PA spatulas included 95 different chemicals, 54 verified using reference standards.
According to researchers, the detection of chemicals in FCMs can be aided by creating a database comprising projected RT and CCS values of compounds associated to FCMs.
Predicted RT and CCS values can be added to the identification procedure to increase identification confidence, although they do not conclusively show the compound's existence.
Conclusion
By screening against chemicals in two public databases (CPPdb and FCCdb) with improved predicted RT and CCS values based on machine learning, different compounds present in FCMs can be discovered.
Predicted RT and CCS values can be employed efficiently to drastically reduce false positives and enhance the accuracy of identification. Adding more measured values to the appropriate training sets increases RT and CCS forecasts' accuracy.
Reference
Song, X.-C., Canellas, E., Dreolin, N., Goshawk, J., & Nerin, C. (2022). Identification of Nonvolatile Migrates from Food Contact Materials Using Ion Mobility–High-Resolution Mass Spectrometry and in Silico Prediction Tools. Journal of Agricultural and Food Chemistry. https://pubs.acs.org/doi/10.1021/acs.jafc.2c03615
Disclaimer: The views expressed here are those of the author expressed in their private capacity and do not necessarily represent the views of AZoM.com Limited T/A AZoNetwork the owner and operator of this website. This disclaimer forms part of the Terms and conditions of use of this website.