In a new feature, AZoOptics talks with Dr. Quentin Meyer and Dr. Ying Da Wang about their novel technology that allows for superior imaging of hydrogen fuel cells.
Please can you introduce yourself and your professional background?
We are emerging leaders in our field of research, working currently in the UNSW School of Chemistry and School of Mineral and Energy Resources, both with PhDs obtained in the last seven years. We are based in sunny Sydney, Australia. Dr. Quentin Meyer works on hydrogen fuel cell research from catalysis to systems and Dr. Ying Da Wang works on multi-scale imaging of porous media.
What are the basic principles of X-ray imaging, and what are its applications?
X-ray microcomputed imaging, also known as X-ray micro-CT, is a non-destructive imaging technique that uses X-rays to create 3D images of an object's internal structure. The basic principles of X-ray micro-CT involve the following steps:
- X-ray source: X-ray micro-CT uses a high-resolution X-ray source to generate a beam of X-rays that passes through the object being imaged.
- Sample preparation: The object being imaged is typically placed in a sample holder that keeps it stationary during the imaging process. In some cases, the sample may need to be coated with a contrast agent to enhance the visibility of certain structures.
- X-ray detection: As the X-ray beam passes through the sample, it is absorbed by the different materials in the sample to varying degrees. An X-ray detector on the opposite side of the sample measures the intensity of the X-rays that pass through the sample.
- Reconstruction: The data collected by the X-ray detector is used to reconstruct a 3D image of the internal structure of the sample using mathematical algorithms.
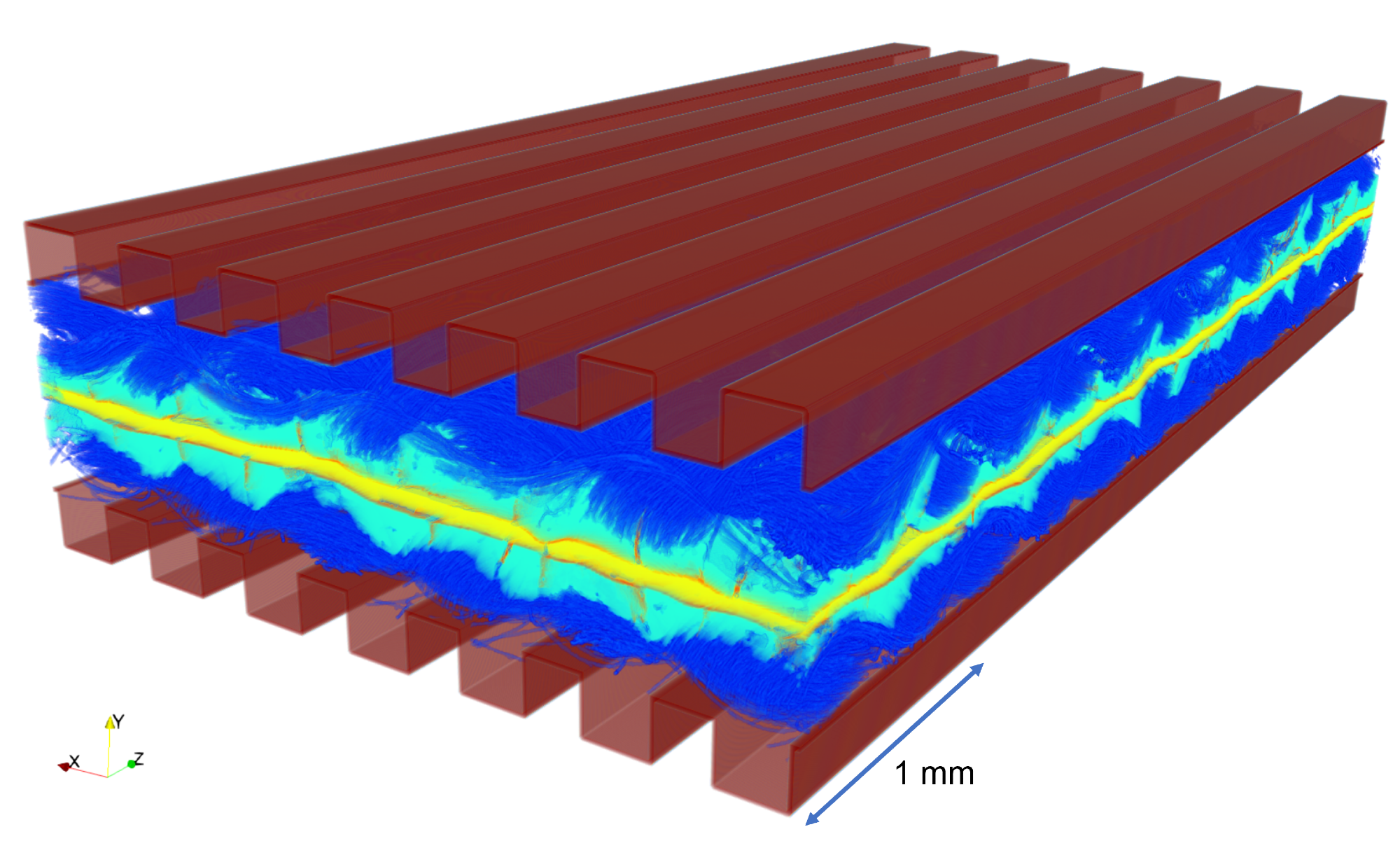
X-ray micro-CT has a wide range of applications in various fields, including materials science, biology, medicine, and engineering. Some of the specific applications of X-ray micro-CT include:
- Material analysis: X-ray micro-CT can be used to study the internal structure and composition of a wide range of materials, such as metals, polymers, and ceramics. This can be useful for understanding the properties and performance of these materials.
- Biomedical research: X-ray micro-CT can be used to image biological specimens, such as tissues, organs, and bones. This can be useful for studying the anatomy and morphology of these structures, as well as for understanding diseases and their progression.
- Archaeology: X-ray micro-CT can be used to study the internal structure of archaeological artifacts, such as fossils and ancient pottery. This can provide insights into the history and development of these objects.
- Non-destructive testing: X-ray micro-CT can be used to inspect and evaluate the internal structure of manufactured components, such as electronic components and composite materials. This can help to detect defects or flaws that may affect their performance or reliability.
Overall, X-ray micro-CT is a powerful imaging technique that can provide detailed 3D images of the internal structure of a wide range of objects, with numerous applications in various fields.
What are hydrogen fuel cells and why are they important?
Hydrogen fuel cells are electrochemical energy devices that use hydrogen fuel to generate electricity and are a quiet and clean energy source that can power homes, vehicles, and industries. These fuel cells convert the hydrogen, via an electrochemical process, into electricity with the only by-product of the reaction being pure water. In addition, they are compact and lightweight, so they can be used in vehicles. Hydrogen fuel cells have the advantage of rapid refueling, as quickly as refueling a car with petrol, giving them a key advantage over battery-powered EVs which can take many hours even with a rapid charger. They are crucial for the hydrogen economy and future decarbonization efforts and are considered the ultimate clean energy source, given that hydrogen is produced from clean sources as well.
Why is it necessary to produce detailed images of hydrogen fuel cells, and why is X-ray imaging used for this?
Proton exchange membrane fuel cells (PEMFCs for short) are the type of fuel cell we have used in our study. They are multi-scale porous materials with a solid electrolyte membrane sandwiched between nanoporous electrocatalyst on both sides, covered by a microporous layer (MPL), a microporous gas diffusion layer (GDL), and topped by millimeter-scale flow channels.
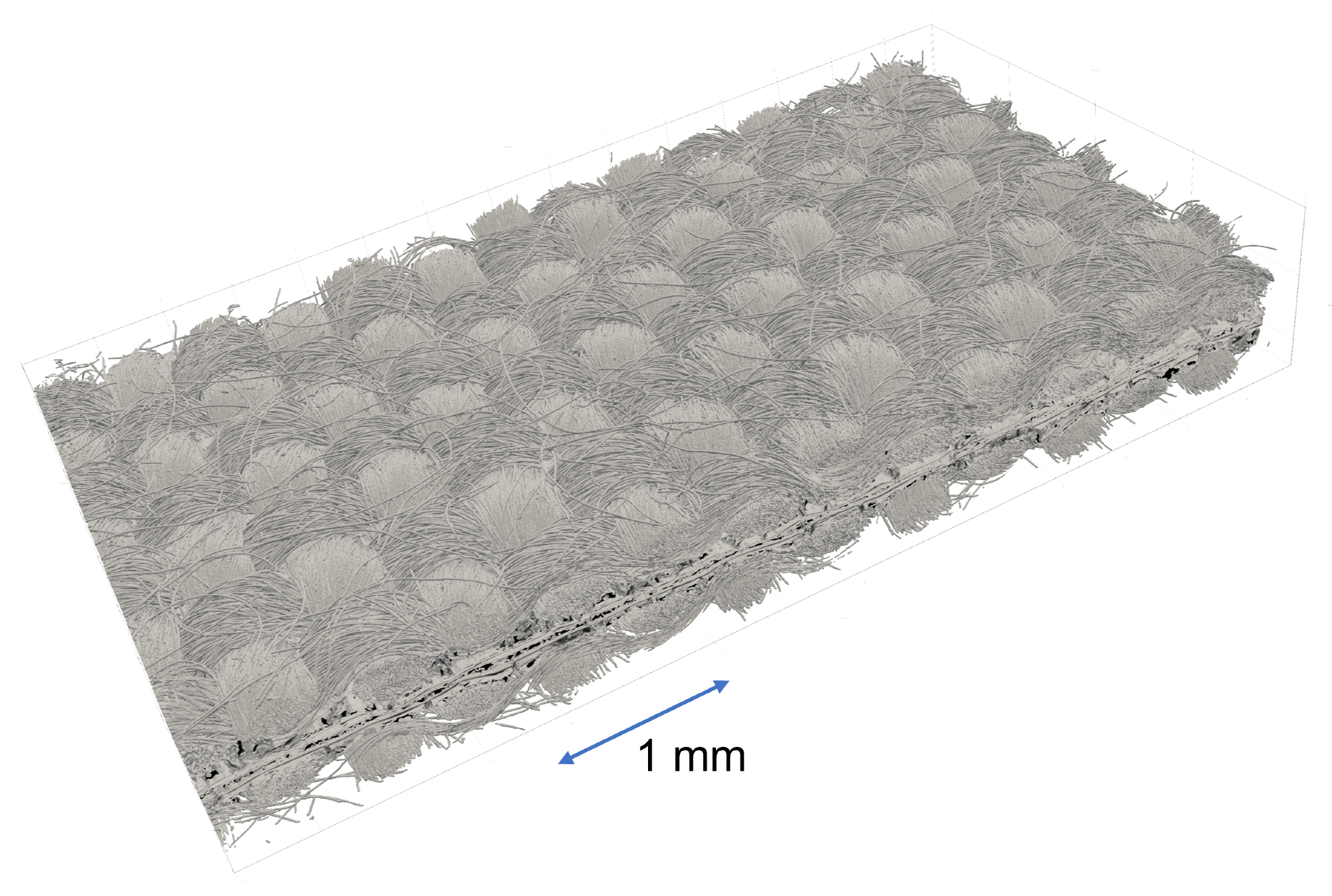
It’s a few millimeters thick in total (~2 mm usually including 1 mm flow channels). This structure both maximizes gas diffusion to the active catalytic sites and minimizes water accumulation in the catalyst layers. As the materials are bonded together, they cannot be easily separated for imaging without destroying the entire structure, and therefore it is difficult to understand how all these materials interact with each other. X-ray imaging has become widely used to characterize these materials without destroying them.
What limitations with this method led to your search for an enhanced imaging technique?
Typical fuel cell samples imaged with laboratory X-rays are less than 2 millimeters to resolve 1 micrometer (0.001 mm), so pretty tiny. But hydrogen fuel cells are pretty large (1-200 cm2), so it's hard to know if the 2 mm imaged area is representative of a large area. If you zoom out to capture a larger area, you capture fewer features. It is therefore very difficult (or impossible really!) to image a large area (1 cm) with high resolution, which was the drive for our research toward an enhanced imaging technique using the new and exciting deep-learning method.
How is your new algorithm able to improve the resolution of X-ray images?
Our DualEDSR algorithm solution uses deep-learning artificial intelligence (AI) to create a detailed 3D model by utilizing a low-resolution X-ray image of the entire cell and extrapolating data from an accompanying high-res scan of a small sub-section. As an analogy, we have taken a blurry aerial view of a town and an optically zoomed-in photo of a few streets and then accurately predicted the layout of every road in the area! In the case of 3D X-ray images of fuel cells as per this study, our custom neural network is designed to efficiently generate terabyte-scale models on desktop computers. This is particularly exciting because we are pushing the imaging resolution limits by a few orders of magnitude using machine learning and AI!
How significantly does the DualEDSR algorithm improve the resolution?
Our DualEDSR algorithm improves the field of view by around one hundred times compared to the upper limits of the hardware. A key advantage is its unprecedented computational efficiency, which is well suited to 3D data, which traditional deep learning methods struggle with as the domain sizes get much larger much faster compared to 2D images.
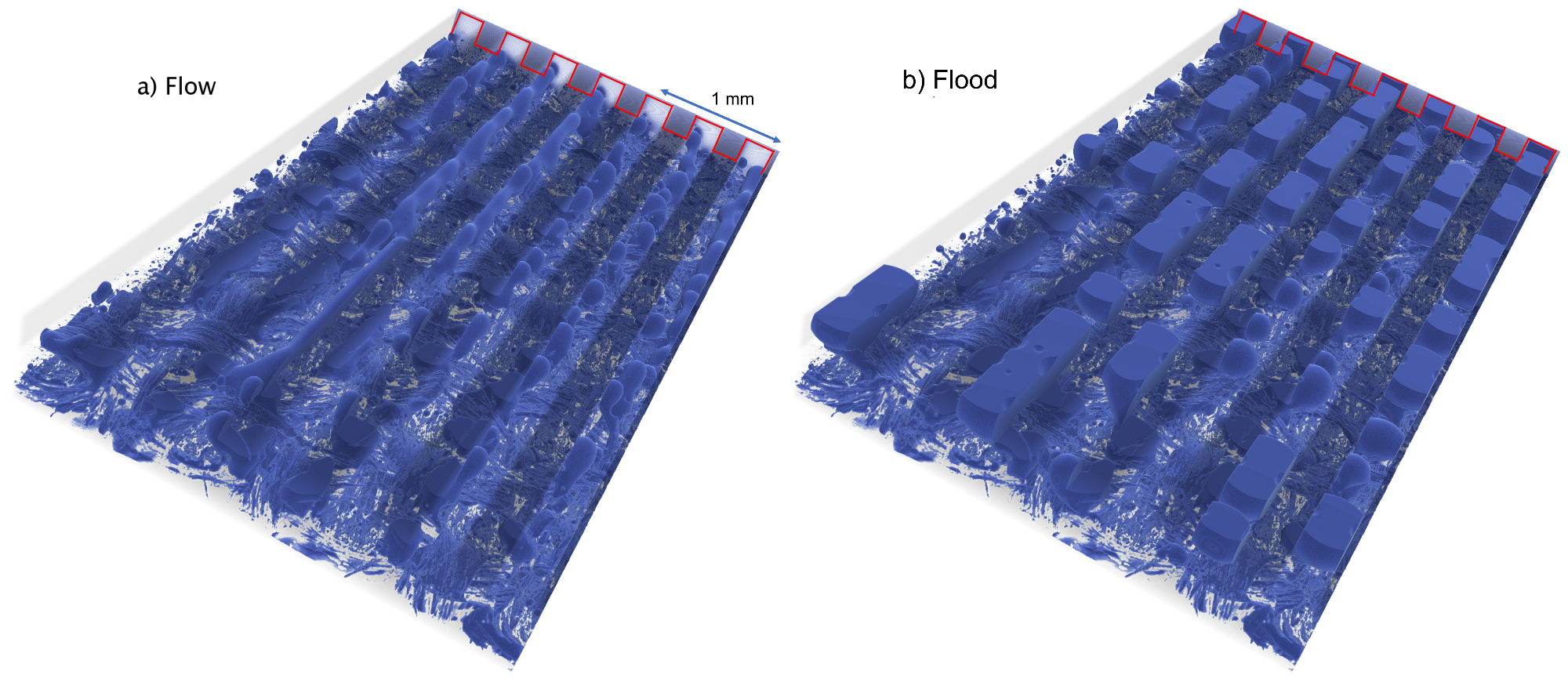
What did you learn about hydrogen fuel cells using your new technology, and what developments can now be achieved?
From our model, we quickly and precisely identify where water accumulates, and we potentially solve those problems in future designs. There is a huge untapped performance improvement that could be made using these cells just by improved water management, and that is estimated to be a 60 percent increase overall. For the past 20 years, up until now, it has been extremely hard to have an accurate model of these fuel cells because of the complexity of both the materials and the way gases and liquids are transported, as well as the electrochemical reactions taking place. Our team has enabled us to do just that, bringing so much different expertise to the table. This is what research is about.
PEMFC simulation video
Video Credit: UNSW Community/Shutterstock.com
How significant do you think machine learning will become to fields such as photonics and medical imaging?
If you were to look at what we are doing now and apply it to the medical field, we could for instance image blood vessels and model the flow of red blood cells through the capillary network in greater detail. In fact, machine learning has already made significant contributions in these areas.
In photonics, machine learning algorithms are being used to design and optimize optical devices and systems. For example, machine learning has been used to optimize the design of photonic crystals, which are materials that can manipulate light in unusual ways. Machine learning algorithms can also be used to improve the performance of optical sensors, such as those used for chemical sensing or bioimaging. In medical imaging, machine learning is being used to improve the accuracy of diagnosis and treatment planning. For example, machine learning algorithms can analyze medical images to identify patterns that are difficult for humans to discern. This can help doctors to detect and diagnose diseases earlier, leading to better outcomes for patients. Machine learning can also be used to help doctors plan surgeries and other treatments by predicting how different interventions will affect the patient.
Overall, machine learning is likely to become increasingly significant in photonics, and medical imaging as researchers and engineers continue to develop new applications and techniques.
Of course, these methods are not without their limitations and cautions for their uses.
- Data quality: Machine learning algorithms require high-quality data to function properly. In photonics and medical imaging, data quality can be affected by a range of factors, such as noise, artifacts, and imaging artifacts. If the data is of poor quality, this can affect the accuracy of the machine learning results.
- Bias: Machine learning algorithms can be biased, meaning that they may produce inaccurate results or recommendations based on biases in the data. This can be particularly problematic in medical imaging, where bias could lead to incorrect diagnoses or treatment plans.
- Interpretability: Machine learning algorithms can be difficult to interpret, meaning that it may be hard to understand how they arrived at a particular result or decision. This can be a problem in medical imaging, where doctors need to be able to understand how the algorithm arrived at a particular diagnosis or treatment plan.
- Generalizability: Machine learning algorithms can be limited by their ability to generalize to new data or situations. For example, an algorithm trained on one type of medical imaging data may not perform as well when applied to a different type of imaging data.
- Ethical considerations: The use of machine learning in medical imaging raises ethical considerations around issues such as privacy, security, and informed consent. For example, there may be concerns about how patient data is being used and shared and whether patients are fully informed about the risks and benefits of machine learning-based diagnoses and treatments.
Did you learn anything surprising or unexpected during the course of conducting this study?
We were able to see the difference between different modes of operations and realized that small cracks inside the gas diffusion layer made a huge difference in water transport. We also saw how water easily forms big clusters and floods localized in some areas, and it can be very difficult to remove it.
What are the next steps for this research?
We have many different directions. We are considering applying this technology to a water electrolyzer that produces green hydrogen to capture hydrogen formation and transport, to other types of fuel cells operating at higher temperatures, and to other energy applications. We could also change the materials' properties (porosity and hydrophobicity) and see how the water map changes. We have endless possibilities to go to, and just need to find the most promising one.
Where can readers find more information?
Ying Da Wang et al, Large-scale physically accurate modelling of real proton exchange membrane fuel cell with deep learning, Nature Communications (2023). DOI: 10.1038/s41467-023-35973-8
About Dr. Quentin Meyer and Dr. Ying Da Wang
Dr. Quentin Meyer defended his Ph.D. in Advanced Diagnosis for Proton Exchange Membrane Fuel Cells in 2015 with an Award for Research Excellence (University College London, London UK). After a post-doctoral position in 3-D imaging of hydrogen fuel cells at University College London, he relocated to Australia in 2017 and joined Prof Zhao’s group as a senior researcher and laboratory manager (>20 people) at the University of New South Wales (Sydney, Australia) in 2018. His research topics deal with the electrocatalysis of complex reactions, focusing on the structure-to-performance relationships, and investigating the activity and durability of low-cost electrocatalysts for fuel cell and electrolyzer applications. As of February 2023, he has published 52 journal articles, filed a patent, and given over twenty-five communications at national and international conferences and ten invited seminars.
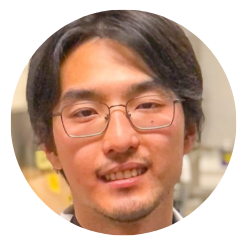
Dr. Ying Da Wang obtained his Ph.D. in Machine Learning and Efficient Computational Methods for Digital Porous Media in 2020 at the University of New South Wales, Australia, and is currently a post-doctoral researcher at the same institution. He supervises and manages the computational modelling side of the MUTRIS (Multiscale Transport in Porous Systems) research group under Prof. Ryan Armstrong and Prof. Peyman Mostaghimi. His research focuses on (a) Machine Learning Methods for Digital Porous Media, and (b) Efficient and Massive Computations of Pore Scale Flow, which has garnered him expertise in Super Resolution and Image Enhancement, Multi-Label Segmentation, and High-Performance Flow Simulation. As of February 2023, he has published 27 journal articles and presented his findings at multiple technical conferences.
Disclaimer: The views expressed here are those of the interviewee and do not necessarily represent the views of AZoM.com Limited (T/A) AZoNetwork, the owner and operator of this website. This disclaimer forms part of the Terms and Conditions of use of this website.