A study published in Remote Sensing proposed a multilevel stacked context network (MSCNet) to enhance target detection accuracy and the feature pyramid network (FPN) representation by aggregating the logical relationships between different contexts and objects in remote sensing images.
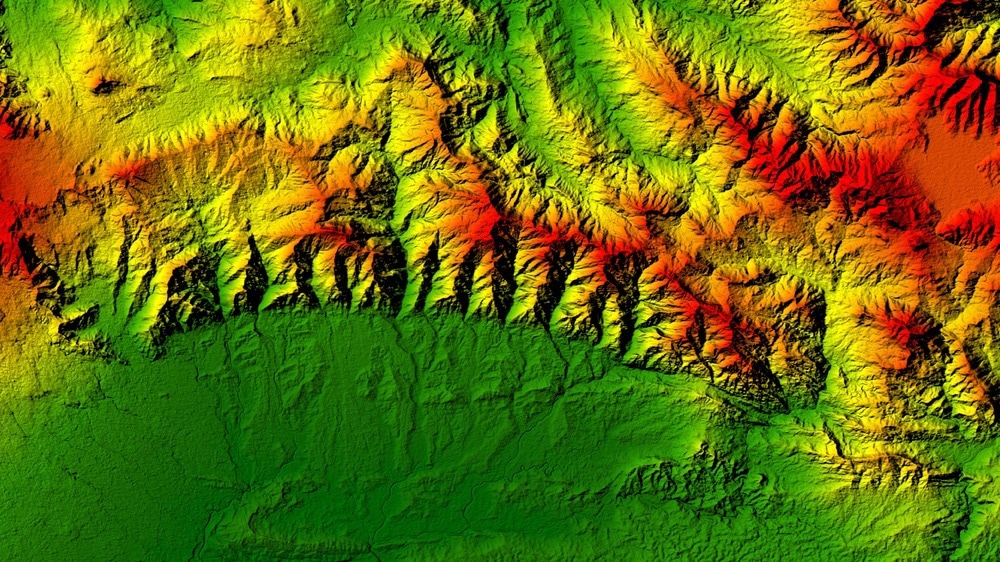
Study: MSCNet: A Multilevel Stacked Context Network for Oriented Object Detection in Optical Remote Sensing Images. Image Credit: Rahman Hilmy Nugroho/Shutterstock.com
A Review of Convolutional Neural Networks in Remote Sensing Image
The remote sensing data’s acquisition costs have been steadily decreasing, data sources have been steadily expanding, and image resolution and quality have improved due to the exponential growth of remote sensing technology. As a result, remote sensing imagery is increasingly adopted across various sectors.
Convolutional neural networks have recently emerged as a powerful technique for analyzing remote sensing data. It generates feature representations directly from the original image pixels, while its deep stacked structure aids in extracting more abstract semantic features. These outcomes are unattainable via traditional image construction techniques.
However, the orthographic nature of optical remote sensing images makes it challenging for object identification networks to deal with objects moving in random directions in remote sensing images.
The impact of direction is negligible in natural images than in remote sensing images since most objects in natural images are inclined towards the ground due to gravity. This creates new challenges since remote sensing images are captured from above Earth, and objects on the surface have different orientations.
To overcome these challenges, researchers created oriented detection bounding boxes in place of typical horizontal detection bounding boxes to increase detection performance in remote-oriented object detection and classification.
The use of faster deep convoluted neural networks with a feature pyramid network (FPN) structure, such CAD-Net and DRBox, have led to significant advancements in this area. Although these approaches have shown good performance across multiple datasets, there is still potential for improvement.
Numerous studies have shown that deeper image features in deep convolutional networks include more semantic information, whereas lower features reveal more boundary details of objects. In addition, these images demonstrate extra semantic and spatial connections between objects than natural images.
Unfortunately, modeling the connection between two distant objects is challenging with the convolution method due to its local restrictions. Therefore, enhancing the detection network’s performance is essential by capturing and modeling the relationships between contexts and objects and explicitly modeling long-range linkages.
Using Multi-Sector Oriented Object Detector for Accurate Localization in Optical Remote Sensing Images
This study developed a multilevel stacked context network (MSCNet) for remote sensing-oriented object detection. Using multilevel structures, the network’s modeling ability for the relationship between objects at different distances was improved to address the shortcoming of conventional object detectors in remote sensing images.
The multilevel stacked semantic capture (MSSC) module was placed within the network to capture the interaction between distant items. This module obtained diverse receiving domains by adding numerous parallel dilated convolution layers with varying dilated rates to ResNet’s c5 layer and overlaying the convolutions locally to maximize the context relations at different distances.
Additionally, a multichannel weighted fusion of the region of interest (RoI) was carried out in the region proposal network stage to improve the RoI features for subsequent classification and regression operations.
Significant Findings of the Study
The feature pyramid network (FPN) representation on remote sensing images and the spatial relationship and recognition accuracy were improved by the proposed multilevel stacked semantic enhancement and acquisition module.
The characteristics produced by various region proposal network levels increased the detection precision of small targets when the MSSC and adaptive RoI assignment (ARA) modules were combined. In addition, the standard-oriented bounding box representation’s criticality issues were addressed by the Gaussian Wasserstein distance (GWD) loss.
A multichannel weighted fusion structure, rather than a single layer allocation method, was used in an upgraded RoI allocation strategy to increase the FPN multilevel information aggregation, which improved the detection performance of level targets.
Experimental results also reveal that the proposed technique has the highest performance in the relevant oriented object detection networks and surpasses the baseline network on two open-source remote sensing datasets.
However, this study still has various shortcomings. For example, in the ablation study, the combined MSSC and ARA result on the DOTA dataset was significantly worse than the ARA result. Future research must investigate why these issues arise and identify a better mix of MSSC and ARA to produce a more successful multilevel feature allocation approach.
Reference
Zhang, R., Zhang, X., Zheng, Y., Wang, D., & Hua, L. (2022) MSCNet: A Multilevel Stacked Context Network for Oriented Object Detection in Optical Remote Sensing Images. Remote Sensing. https://www.mdpi.com/2072-4292/14/20/5066
Disclaimer: The views expressed here are those of the author expressed in their private capacity and do not necessarily represent the views of AZoM.com Limited T/A AZoNetwork the owner and operator of this website. This disclaimer forms part of the Terms and conditions of use of this website.