In an article published in Remote Sensing, researchers proposed a novel double branch-based Siamese change detection network popularly known as DSNUNet to efficiently integrate synthetic aperture radar (SAR) and optical data in forest change detection.
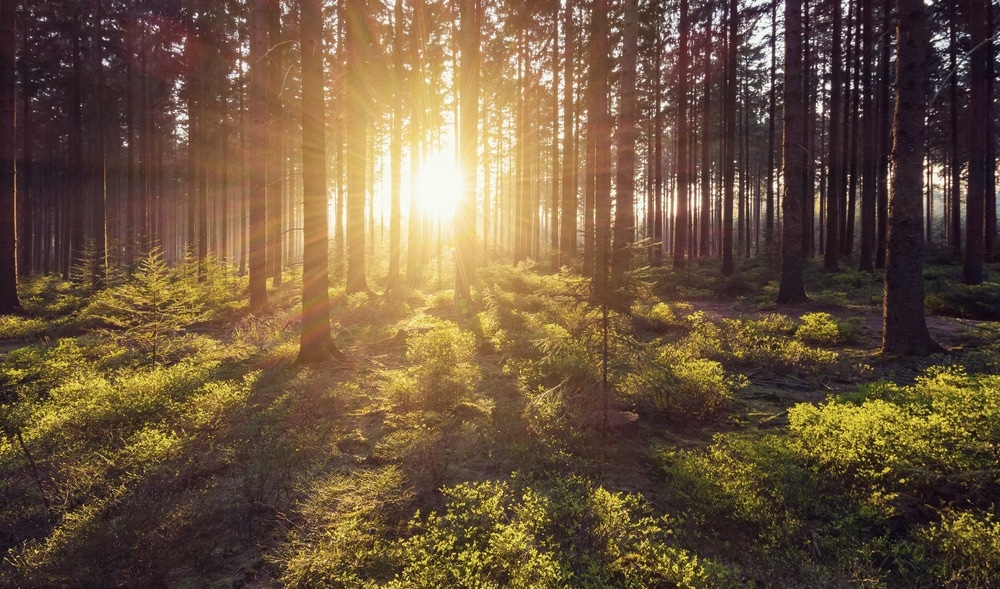
Study: DSNUNet: An Improved Forest Change Detection Network by Combining Sentinel-1 and Sentinel-2 Images. Image Credit: r.classen/Shutterstock.com
DSNUNet used dual-phase SAR and optical images to extract features, which were then combined into groups using shared weights. Various feature extraction branch widths were employed in the proposed DSNUNet to account for the information amount differences between SAR and optical images.
Investigations on manually annotated forest change detection databases validated the proposed DSNUNet. According to the findings, the approach outperformed existing change detection methods, earning a 76.40% F1-score. In this study, several permutations of width among feature extraction branches were also examined.
The findings showed that the model performed best at the initial channel number of 32 for the optical imaging branch and eight for the SAR image branch. The prediction results demonstrated the accuracy of the proposed strategy in predicting changes in the forest and partially controlling cloud interferences.
Applying Remote Sensing in Forest Change Detection
In remote sensing, which seeks to uncover surface changes in multi-temporal distant sensing data, change detection is a crucial task. Natural resources such as forests are vital for preserving the planet's ecological balance. Forest change detection has been utilized extensively in land and resource inventory, forest management, and deforestation prevention as a sub-task of change detection.
Traditional forest change detection was typically performed by applying optical images with distinct color properties and specific color bands sensitive to certain changes. The primary data source in change detection at present is optical images. Clouds and fog, however, significantly impact the effectiveness of these optical images.
Spectral shifts for the same objects may be visible in multi-phase images taken by a sensor across a temporal difference. Numerous forest change detection research based on SAR images has been carried out thanks to the advancement of SAR technology recently.
Conventional forest change detection techniques primarily use algebraic algorithms, data transformation techniques, classification-based approaches, and canonical correlation analysis. The initial properties of an image are the exclusive features used by these conventional algorithms, which typically have poor precision in forest change detection. Deep learning algorithms have been applied in image classification, semantic segmentation, and target recognition showing remarkable performance due to the recent rapid growth of computer vision and deep learning algorithms.
The double-Siamese nested U-Net (DSNUNet) model, based on the encoder-decoder structure, was suggested to increase the accuracy of forest change detection. Two sets of Siamese branches were included in the encoder, which was utilized to extract the features from SAR and optical images. At the same time, the decoder returned the scale features while aggregating the SAR and optical characteristics.
Various feature channel permutations were employed in the proposed model to extract useful features from the SAR and optical images and account for the variations in these image data.
The model's loss function included focal loss and dice loss to address the differences between positive and negative values in the change detection task. The suggested model was then verified using Sentinel-1 and Sentinel-2 data. Compared to state-of-the-art approaches, the results showed that the method was more effective in detecting forest change in terms of accuracy, recall, and F1-Score.
Conducting the Investigations
The Sentinel-2 satellite's L1C level data were used in this investigation as the optical image data. The RGB input of the detection model was chosen from three bands, including NIR, green, and red. From September to November 2020-2021, data was gathered from the research region and utilized for the change detection analysis.
The Sentinel-1 satellite data's first-order ground-ranging (GRD) product was used to create the SAR image data in this study. The optical image and the SAR data's temporal phase were kept consistent. The intra-quarter mean synthesis was used to reduce the influence of speckles on data quality.
The suggested DSNUNet was a forest change detection system based on Siamese networks that detected changes in forests using optical and SAR data. SNUNet-CD model served as the foundation for the DSNUNet paradigm.
The DSNUNet model, in contrast to other change detection models, could effectively extract data features from various time phases and modes. Moreover, it accepted both SAR and optical images (VV+VH) as input. The DSNUNet output was found to be a good change map.
Diverse channels of features were typically regarded as various patterns of elements in the deep learning domain. For each of the two categories of data, different convolutional kernel combinations were therefore employed in this work.
Several experiments were used to validate the proposed DSNUNet model's performance. The findings were compared with those obtained using several deep-learning change detection models to judge the efficacy of the DSNUNet model.
Since SAR could produce high-resolution images even in cloudy conditions, DSNUNet was more tolerant of clouds in images. Comparatively to previous models, the DSNUNet was better able to reduce the pseudo-variation brought on by cloud layers. Since these cloud-covered images were excluded from the training process, it could be concluded that the model's change detection capabilities improved due to the properties of SAR images.
DSNUNet Enhances Forest Change Detection by Integrating Sentinel-1 and Sentinel-2 Images
This paper demonstrated the DSNUNet, a double branch-based Siamese network for forest change detection. Both optical and SAR images could be used as inputs when employing the DSNUNet model.
The effectiveness and reliability of a change detection algorithm could be significantly increased by using SAR images as a single data source in forest change detection networks.
In contrast to merely integrating two different types of input images, DSNUNet achieved information fusion through convolutional operations in the decoder stage. It extracted features from SAR and optical images using two set coding branches of various widths. The suggested model demonstrated higher assessment metrics when compared to previous forest change detection models.
The results showed that DSNUNet could successfully combine optical and SAR image data, which was crucial for enhancing the effectiveness of forest change detection. This work enhanced the effectiveness of forest resource assessments for regions constantly covered in clouds.
Reference
Jiang, J., Xing, Y., Wei, W., Yan, E., Xiang, J., Mo, D. (2022) DSNUNet: An Improved Forest Change Detection Network by Combining Sentinel-1 and Sentinel-2 Images. Remote Sensing, 14(19), 5046. https://www.mdpi.com/2072-4292/14/19/5046/htm
Disclaimer: The views expressed here are those of the author expressed in their private capacity and do not necessarily represent the views of AZoM.com Limited T/A AZoNetwork the owner and operator of this website. This disclaimer forms part of the Terms and conditions of use of this website.