An article published in Sensors reveals fluorescence lifetime imaging (FLIM), an important tool that provides unique information for biomedical research. The FLIM-MLP-Mixer, a multi-layer perceptron-based mixer (MLP Mixer), and a deep learning (DL) technique are used for quick and reliable fluorescence lifetime imaging analysis.
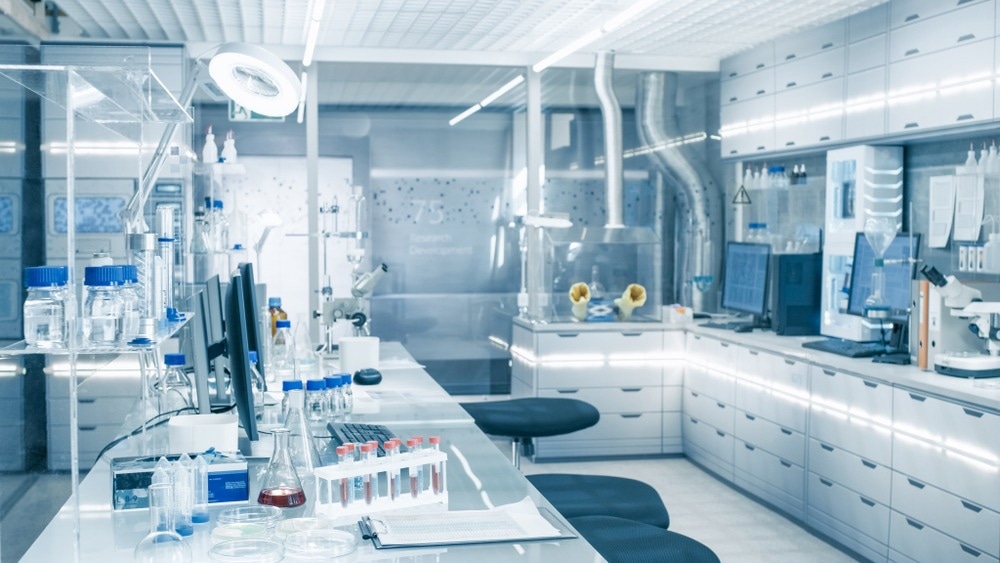
Study: Simple and Robust Deep Learning Approach for Fast Fluorescence Lifetime Imaging. Image Credit: Gorodenkoff/Shutterstock.com
The FLIM-MLP- Mixer exhibits more remarkable performance in terms of accuracy and computation time when compared to the conventional fitting and previously published deep learning methods. The results show that the suggested method has much potential for various real-time fluorescence lifetime imaging (FLIM) applications and is well suited for correctly calculating lifetime parameters from recorded fluorescence histograms.
Deep Learning Technique in Fluorescence Lifetime Imaging
In biomedical research, fluorescence lifetime imaging (FLIM) is an effective method for examining physiological parameters such as pH, viscosity, temperature, and ion concentrations, as well as cellular microenvironments.
Fluorescence lifetimes are local features of fluorophores that only depend on the local microenvironment’s physicochemical state. Due to variations in laser power, fluorophore concentrations, and optical concentration, they are devoid of artifacts.
Fluorescence lifetimes can be measured using both time-domain and frequency-domain methods.
Deep learning techniques for fluorescence lifetime imaging (FLIM) analysis have shown to be effective in mapping the input of the lifetime parameters after extracting high dimensional features from the input decay histograms.
Several neural networks were presented for multi-exponential analysis, including multilayer perceptions, one- and high-dimensional convolutional neural networks, online training extreme learning machines, and generative adversarial networks.
High-precision multi-exponential fluorescence lifetime imaging (FLIM) analysis has used high-dimensional convolutional neural networks.
A lightweight one-dimensional (1D) CNN has been suggested for fluorescence lifetime imaging analysis. High efficacy, quick training, and quick inference speed are all strengths of the 1D-CNN. It can also be used in embedded devices because it is hardware friendly.
The researchers in this study proposed a new MLP-based deep learning algorithm to solve the difficulties. This method uses the MLP-Mixer to give a quick and accurate analysis even with a low signal-to-noise ratio.
The MLP-Mixer is superior to CNNs in three key ways. First, since just matrix multiplications are required, it has higher computational efficiency. Second, the MLP-Mixer is also better suited to analyze sequence signals. With a broad field of vision, it processes the entire decay chain simultaneously. Third, the MLP implementation Mixer and optimization are easy to understand and suited for many applications.
Proof-of-Concept Investigation for the Proposed FLIM-MLP-Mixer Structure
A regression layer, mixer layers, and per-patch linear embedding make up the fluorescence lifetime imaging (FLIM) - multi-layer perceptron-based MLP Mixer. The mixer block has two token-mixing MLPs (MLP1) and MLP (MLP2), each comprising two wholly linked layers and a GELU nonlinearity.
The mixer's purpose is to divide the channel-mixing and token-mixing activities. Basic matrix multiplication routines, which are used by the mixer, require less hardware in terms of memory capacity and power usage. Without complicated matrix calculations, it is substantially faster. Field-programmable gate array (FPGA) devices enable the quick implementation of hardware-friendly MLP neural networks and their integration into a wide-field SPAD FLIM system.
A synthetic mono-exponential signal with a lifespan of 1 or 4 ns was initially used to test the MLP-performance Mixers. There are 20–40 dB SNRs and photon counts between 100–10,000. The MLP-output, Mixer's for signals with a lifespan of 1 ns, reaches 0.95, 0.96, and 0.98 ns at SNRs of 20–32 dB, 32–38 dB, and 38–40 dB, respectively. The MLP-Mixer is a more accurate estimator because its estimation biases for short and long lifetimes are less than 2% and 5%, respectively. All methodologies' short lifespan standard deviations are less than 0.06, which is substantially lower.
A commercial two-photon fluorescence lifetime imaging (FLIM) device was experimentally validated using dye-based solutions and plant cell samples. Saturated aqueous solutions of Rhodamine B and 6G were applied on a glass slide with coverslips. In our investigation, low photon count pixels were masked using a threshold (10% of total counts).
In terms of accuracy, the MLP-Mixer and 1D-CNN perform better. According to the results, the MLP-Mixer is superior to others, even when the SNR is low. This is because a lower SNR results in a lower photon count, which is insufficient for fitting techniques.
The cell samples from Convallaria majalis to assess the MLP-Mixer further. High photon counts (HPC) at a 30 s time, middle photon counts (MPC) at a 15 s time, and low photon counts (LPC) at a 3 s time were all used to measure the sample.
In low SNR scenarios, the structural similarity index (SSIM) values for the MLP-Mixer and nonlinear least-square method (NLSM) are 0.95 and 0.81; respectively, the MLP-Mixer performs noticeably better than NLSM. The deep learning methods are insensitive to varied SNR levels since they extract the characteristics of decay histograms in high-dimensional space.
Significance of the Study
The design and analysis of the MLP-mixer for fluorescence lifetime imaging (FLIM) are discussed in this study. Rhodamine 6G, Rhodamine B, and Convallaria majalis cell two-photon FLIM pictures were analyzed using the trained model. The simulated fluorescence lifetime imaging (FLIM) data study shows that the MLP-Mixer performs better, particularly for components with short lifetimes.
The outcomes also point to the MLP-outstanding Mixer's functionality and dependability. It may be implemented on FPGA devices to speed up analysis for real-time fluorescence lifetime imaging (FLIM) applications because of its straightforward architecture.
Reference
Wang, Q., et al. (2022) Simple and Robust Deep Learning Approach for Fast Fluorescence Lifetime Imaging. Sensors, 22(19), 7293. https://www.mdpi.com/1424-8220/22/19/7293/htm
Disclaimer: The views expressed here are those of the author expressed in their private capacity and do not necessarily represent the views of AZoM.com Limited T/A AZoNetwork the owner and operator of this website. This disclaimer forms part of the Terms and conditions of use of this website.