The industrial automation and machine vision market was worth 13.23 billion USD in 2021 and is expected to continue its growth at a sizeable 7.7 % year on year.1 Machine vision applications enable more sophisticated automation processes where live information on product production can be fed into a feedback loop for manufacturing automation.
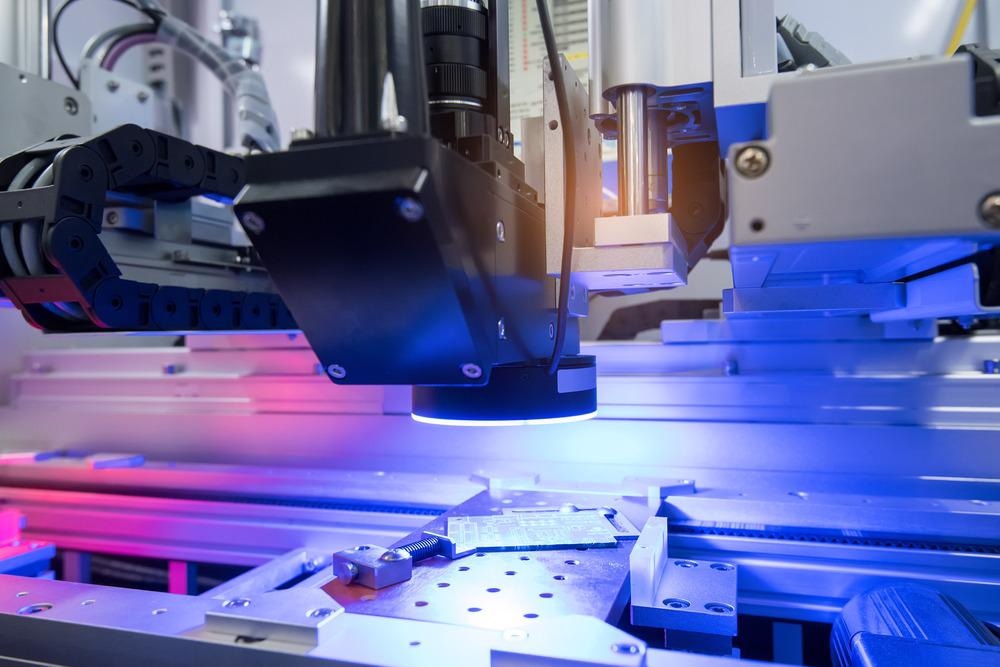
Image Credit: asharkyu/Shutterstock.com
Creating a machine vision live feedback loop improves quality control in manufacturing and the creation of more complex and sophisticated devices, such as the use of ion-beam methods for nanoscale and micro-electromechanical systems (MEMS) manufacturing.2
Using live feedback in automated manufacturing processes allows greater accuracy and precision as any issues with sample positioning can be corrected. This is very important for nanoscale engineering, where the slightest imprecision can lead to manufacturing errors.
The addition of spectroscopy to machine vision systems makes them even more effective. While a combination of machine learning analysis and visible light cameras can be used to inspect defects or check size tolerances in a manufacturing process, spectroscopic methods provide new dimensions of information for quality control and product performance assessment.
Spectroscopy in Machine Vision
Spectroscopic techniques are now being used as part of machine vision systems in many areas, including agriculture for crop health diagnosis and the food industry to identify product freshness and help with product sorting.
In manufacturing, spectroscopies such as infra-red spectroscopy can provide crucial information on the quality of starting materials such as wood.3
By using infrared spectroscopy, it is possible to enhance how the machine vision system recognizes surface defects in materials such as wood.3 Many features in materials such as surface defects are not just characterized by their visual appearance but often have subtly different infrared absorption features.
The infrared absorption frequency and band shape of a local material region can also reflect local stress and strain in the material environment and be used to identify potential failure points in a new product.4
Another application for infrared spectroscopy in machine vision is to identify the degree of blending in pharmaceutical production.5 In combination with another spectroscopic technique, UV-vis spectroscopy, researchers were able to create a highly sensitive and accurate system for monitoring the blending process to provide meaningful feedback in real-time. This way, the concentration of the active pharmaceutical ingredient (API) could be monitored over time. Observing how the API moved through the system during blending was also possible. Many pharmaceutical regulations specify that API concentrations must not be greater in local regions of a given treatment as this can lead to inaccuracies in dosing for patients with potentially dangerous effects.
Sustainability
With a move towards the use of recycled starting materials in manufacturing to improve the sustainability of the manufacturing process, one challenge for automation is to try and deal with inconsistencies in the starting materials.
Polymers in particular are proving to be a significant environmental challenge. Non-biodegradable polymers pose a long-term ecological hazard, but for sterile medical applications and where precise strength-to-weight ratios are needed, it can be challenging to find suitable replacement materials.
One way to circumvent this problem is to recycle polymers, but this often creates complex blends and a need to be able to pre-sort plastics by type. Most types of polymers, while not distinguishable by the eye, have very different infra-red absorption spectra. Therefore, by combining infrared spectroscopy with machine vision, it is possible to characterize even complex blends of polymers to be used as starting materials and optimize manufacturing conditions to the blend as necessary.
Such infrared spectroscopy machine vision is now being used to calculate the polyester content in recycled textile clothing6 and plastic sorting for residential and commercial plastic waste.7
While the initial investment in hyperspectral imaging cameras and feedback systems may be expensive, all of this helps improve the viability of using recycled starting materials and reduce the costs associated with recycling. It also opens the possibility of using more significant numbers of more complex materials in industrial automation.
Machine Learning
While improvements in detector sensitivities and light source qualities have made it easier to rapidly acquire the spectroscopic information needed to provide the data for industrial automation, improvements in the efficiency of the analysis of such complex datasets have been vital in making industrial automation an accurate real-time process.
For feedback systems to benefit and reduce manufacturing times, the time required to acquire the initial spectrum with sufficient signal to noise for analysis and analysis time itself must be incredibly short.
Advances in the efficiency of machine learning algorithms and the development of highly accurate chemometrics methods that can deal with even complex samples in natural environments have made it possible to apply infra-red spectroscopy and several other techniques, including UV-visible and X-ray spectroscopy to industrial automation.8
Spectroscopy now plays a vital role in both quality control and manufacturing automation. As process analytical technologies develop further, this role is likely to become more critical.
References and Further Reading
- Grand View Research (2022) Machine Vision Market Size, https://www.grandviewresearch.com/industry-analysis/machine-vision-market, accessed April 2022
- Peng, M., Cokbas, M., Gallastegi, U. D., Ishwar, P., Konrad, J., Kulis, B., & Goyal, V. K. (2021, October). Convolutional neural network denoising of focused ion beam micrographs. In 2021 IEEE 31st International Workshop on Machine Learning for Signal Processing (MLSP) (pp. 1-6). IEEE. https://doi. 10.1109/MLSP52302.2021.9596272
- Yu, H., Liang, Y., Liang, H., & Zhang, Y. (2019). Recognition of wood surface defects with near infrared spectroscopy and machine vision. Journal of Forestry Research, 30(6), 2379–2386. https://doi.org/10.1007/s11676-018-00874-w
- Bretzlaff, R. S., & Wool, R. P. (1983). Frequency Shifting and Asymmetry in Infrared Bands of Stressed Polymers. Macromolecules, 16(12), 1907–1917. https://doi.org/10.1021/ma00246a019
- Galata, D. L., Mészáros, L. A., Ficzere, M., Vass, P., Nagy, B., Szabó, E., Domokos, A., Farkas, A., Csontos, I., Marosi, G., & Nagy, Z. K. (2021). Continuous blending monitored and feedback controlled by machine vision-based PAT tool. Journal of Pharmaceutical and Biomedical Analysis, 196. https://doi.org/10.1016/j.jpba.2021.113902
- Mäkelä, M., Rissanen, M., & Sixta, H. (2020). Machine vision estimates the polyester content in recyclable waste textiles. Resources, Conservation and Recycling, 161(June), 105007. https://doi.org/10.1016/j.resconrec.2020.105007
- Wu, X., Li, J., Yao, L., & Xu, Z. (2020). Auto-sorting commonly recovered plastics from waste household appliances and electronics using near-infrared spectroscopy. Journal of Cleaner Production, 246, 118732. https://doi.org/10.1016/j.jclepro.2019.118732
- Brunel, B., Alsamad, F., & Piot, O. (2021). Toward automated machine learning in vibrational spectroscopy: Use and settings of genetic algorithms for pre-processing and regression optimization. Chemometrics and Intelligent Laboratory Systems, 219(October), 104444. https://doi.org/10.1016/j.chemolab.2021.104444
Disclaimer: The views expressed here are those of the author expressed in their private capacity and do not necessarily represent the views of AZoM.com Limited T/A AZoNetwork the owner and operator of this website. This disclaimer forms part of the Terms and conditions of use of this website.